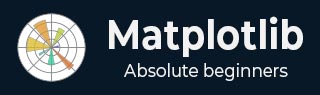
- Matplotlib Basics
- Matplotlib - Home
- Matplotlib - Introduction
- Matplotlib - Vs Seaborn
- Matplotlib - Environment Setup
- Matplotlib - Anaconda distribution
- Matplotlib - Jupyter Notebook
- Matplotlib - Pyplot API
- Matplotlib - Simple Plot
- Matplotlib - Saving Figures
- Matplotlib - Markers
- Matplotlib - Figures
- Matplotlib - Styles
- Matplotlib - Legends
- Matplotlib - Colors
- Matplotlib - Colormaps
- Matplotlib - Colormap Normalization
- Matplotlib - Choosing Colormaps
- Matplotlib - Colorbars
- Matplotlib - Working With Text
- Matplotlib - Text properties
- Matplotlib - Subplot Titles
- Matplotlib - Images
- Matplotlib - Image Masking
- Matplotlib - Annotations
- Matplotlib - Arrows
- Matplotlib - Fonts
- Matplotlib - Font Indexing
- Matplotlib - Font Properties
- Matplotlib - Scales
- Matplotlib - LaTeX
- Matplotlib - LaTeX Text Formatting in Annotations
- Matplotlib - PostScript
- Matplotlib - Mathematical Expressions
- Matplotlib - Animations
- Matplotlib - Celluloid Library
- Matplotlib - Blitting
- Matplotlib - Toolkits
- Matplotlib - Artists
- Matplotlib - Styling with Cycler
- Matplotlib - Paths
- Matplotlib - Path Effects
- Matplotlib - Transforms
- Matplotlib - Ticks and Tick Labels
- Matplotlib - Radian Ticks
- Matplotlib - Dateticks
- Matplotlib - Tick Formatters
- Matplotlib - Tick Locators
- Matplotlib - Basic Units
- Matplotlib - Autoscaling
- Matplotlib - Reverse Axes
- Matplotlib - Logarithmic Axes
- Matplotlib - Symlog
- Matplotlib - Unit Handling
- Matplotlib - Ellipse with Units
- Matplotlib - Spines
- Matplotlib - Axis Ranges
- Matplotlib - Axis Scales
- Matplotlib - Axis Ticks
- Matplotlib - Formatting Axes
- Matplotlib - Axes Class
- Matplotlib - Twin Axes
- Matplotlib - Figure Class
- Matplotlib - Multiplots
- Matplotlib - Grids
- Matplotlib - Object-oriented Interface
- Matplotlib - PyLab module
- Matplotlib - Subplots() Function
- Matplotlib - Subplot2grid() Function
- Matplotlib - Anchored Artists
- Matplotlib - Manual Contour
- Matplotlib - Coords Report
- Matplotlib - AGG filter
- Matplotlib - Ribbon Box
- Matplotlib - Fill Spiral
- Matplotlib - Findobj Demo
- Matplotlib - Hyperlinks
- Matplotlib - Image Thumbnail
- Matplotlib - Plotting with Keywords
- Matplotlib - Create Logo
- Matplotlib - Multipage PDF
- Matplotlib - Multiprocessing
- Matplotlib - Print Stdout
- Matplotlib - Compound Path
- Matplotlib - Sankey Class
- Matplotlib - MRI with EEG
- Matplotlib - Stylesheets
- Matplotlib - Background Colors
- Matplotlib - Basemap
- Matplotlib Event Handling
- Matplotlib - Event Handling
- Matplotlib - Close Event
- Matplotlib - Mouse Move
- Matplotlib - Click Events
- Matplotlib - Scroll Event
- Matplotlib - Keypress Event
- Matplotlib - Pick Event
- Matplotlib - Looking Glass
- Matplotlib - Path Editor
- Matplotlib - Poly Editor
- Matplotlib - Timers
- Matplotlib - Viewlims
- Matplotlib - Zoom Window
- Matplotlib Widgets
- Matplotlib - Cursor Widget
- Matplotlib - Annotated Cursor
- Matplotlib - Buttons Widget
- Matplotlib - Check Buttons
- Matplotlib - Lasso Selector
- Matplotlib - Menu Widget
- Matplotlib - Mouse Cursor
- Matplotlib - Multicursor
- Matplotlib - Polygon Selector
- Matplotlib - Radio Buttons
- Matplotlib - RangeSlider
- Matplotlib - Rectangle Selector
- Matplotlib - Ellipse Selector
- Matplotlib - Slider Widget
- Matplotlib - Span Selector
- Matplotlib - Textbox
- Matplotlib Plotting
- Matplotlib - Line Plots
- Matplotlib - Area Plots
- Matplotlib - Bar Graphs
- Matplotlib - Histogram
- Matplotlib - Pie Chart
- Matplotlib - Scatter Plot
- Matplotlib - Box Plot
- Matplotlib - Arrow Demo
- Matplotlib - Fancy Boxes
- Matplotlib - Zorder Demo
- Matplotlib - Hatch Demo
- Matplotlib - Mmh Donuts
- Matplotlib - Ellipse Demo
- Matplotlib - Bezier Curve
- Matplotlib - Bubble Plots
- Matplotlib - Stacked Plots
- Matplotlib - Table Charts
- Matplotlib - Polar Charts
- Matplotlib - Hexagonal bin Plots
- Matplotlib - Violin Plot
- Matplotlib - Event Plot
- Matplotlib - Heatmap
- Matplotlib - Stairs Plots
- Matplotlib - Errorbar
- Matplotlib - Hinton Diagram
- Matplotlib - Contour Plot
- Matplotlib - Wireframe Plots
- Matplotlib - Surface Plots
- Matplotlib - Triangulations
- Matplotlib - Stream plot
- Matplotlib - Ishikawa Diagram
- Matplotlib - 3D Plotting
- Matplotlib - 3D Lines
- Matplotlib - 3D Scatter Plots
- Matplotlib - 3D Contour Plot
- Matplotlib - 3D Bar Plots
- Matplotlib - 3D Wireframe Plot
- Matplotlib - 3D Surface Plot
- Matplotlib - 3D Vignettes
- Matplotlib - 3D Volumes
- Matplotlib - 3D Voxels
- Matplotlib - Time Plots and Signals
- Matplotlib - Filled Plots
- Matplotlib - Step Plots
- Matplotlib - XKCD Style
- Matplotlib - Quiver Plot
- Matplotlib - Stem Plots
- Matplotlib - Visualizing Vectors
- Matplotlib - Audio Visualization
- Matplotlib - Audio Processing
- Matplotlib Useful Resources
- Matplotlib - Quick Guide
- Matplotlib - Useful Resources
- Matplotlib - Discussion
Matplotlib - Artists
Understanding Artists in Matplotlib
In Matplotlib, almost everything you see on a plot is an instance of an Artist, which are objects that represent various components of a plot. Whether it's a line representing data, a text label, or even the tick marks on an axis, everything in a Matplotlib plot is an Artist object.
Before exploring the Artist hierarchy, let’s observe the below image to understand the basic components of a figure in matplotlib −

In the figure, each element, such as lines and points that represent data, along with minor ticks and points associated text labels, can be identified as individual Artist objects.
Artist Hierarchy
In matplotlib's hierarchy, Artists can be broadly categorized into two types −
- Primitive Artists − These are the basic building blocks of a plot. Examples include Line2D, Rectangle, Text, AxesImage, and more. These are the standard graphical objects that we want to paint onto our canvas.
- Composite Artists − These are higher-level structures that contain other Artists. Examples include Figure, Axis, and Axes. A Figure is like a canvas that holds everything, while Axes represents a specific plot within the figure.
Creating and Accessing the Artists
Artists are typically created through plotting methods on an Axes object. These methods not only facilitate the creation of visual elements but also return specific artist instances corresponding to the plotted elements.
Below are some common plotting methods and the corresponding artists they generate −
- annotate − Generates an Annotation artist for text annotations.
- bar − Creates a Rectangle artist for bar charts.
- errorbar − Produces Line2D and Rectangle artists for error bar plots.
- fill − Generates a Polygon artist for shared areas.
- hist − Creates Rectangle artists for histograms.
- imshow − Generates an AxesImage artist for image data.
- legend − Produces a Legend artist for Axes legends.
- plot − Creates Line2D artists for XY plots. Returns a list when multiple datasets are plotted.
- scatter − Generates a PolyCollection artist for scatter charts.
- text − Produces a Text artist for displaying text on the plot.
Example
Let's see an example for creating and accessing the artists using the plot() method.
import matplotlib.pyplot as plt import matplotlib.artist as martist import numpy as np # Create a subplot fig, ax = plt.subplots() # Generate random data x, y = np.random.rand(2, 100) # Use the plot method to create a Line2D artist lines = ax.plot(x, y, '-', label='example') # Accessing the Line2D artists created by the plot method print('Line2D artists created by the plot method:', lines) # Retrieve all Line2D artists associated with the Axes lines_on_axes = ax.get_lines() # Display the retrieved Line2D artists print('Line2D artists obtained using get_lines():', lines_on_axes) # Accessing the first (and in this case, the only) Line2D artist print('Accessing the first Line2D artist', ax.get_lines()[0])
Output
On executing the above code we will get the following output −
Line2D artists created by the plot method: [<matplotlib.lines.Line2D object at 0x000001DB3666A620>] Line2D artists obtained using get_lines(): Accessing the first Line2D artist Line2D(example)
Modifying the Artists Properties
Artists have various properties that define their appearance and behavior. These properties include color, linestyle, linewidth, and markers. Modifying these properties enables us to control the visual aspects of the plot.
Example
The following example demonstrates how to create a simple plot and then modify the properties of the associated Line2D artist.
import matplotlib.pyplot as plt import numpy as np # Creating a simple plot fig, ax = plt.subplots(figsize=(7, 4)) x = np.linspace(0, 2 * np.pi, 100) y = np.sin(x) # Use the plot method to create a Line2D artist lines = ax.plot(x, y) # Displaying initial properties of the Line2D artist print('Initial properties of the Line2D artist:') print('Color:', ax.get_lines()[0].get_color()) print('Line Style:', ax.get_lines()[0].get_linestyle()) print('Marker Size:', ax.get_lines()[0].get_markersize()) # Modifying properties lines[0].set(color='green', linewidth=2) lines[0].set_linestyle(':') lines[0].set_marker('s') lines[0].set_markersize(10) # Accessing the modified properties of the Line2D artist print('\nModified properties of the Line2D artist:') print('Color:', ax.get_lines()[0].get_color()) print('Line Style:', ax.get_lines()[0].get_linestyle()) print('Marker Size:', ax.get_lines()[0].get_markersize())
Output
On executing the above code we will get the following output −
Initial properties of the Line2D artist: Color: #1f77b4 Line Style: - Marker Size: 6.0 Modified properties of the Line2D artist: Color: green Line Style: : Marker Size: 10.0
Manipulating Artist Data
Beyond styling properties, artists may also contain data. For example, the Line2D object has a data property that can be modified using the set_data() method.
Example
Here is an example that manipulate artist data.
import matplotlib.pyplot as plt import numpy as np # Creating a simple plot fig, ax = plt.subplots(figsize=(7, 4)) x = np.linspace(0, 2 * np.pi, 100) # Initial sinusoidal curve y = np.sin(x) # Use the plot method to create a Line2D artist a sinusoidal curve lines = ax.plot(x, y) # Modifying the artist data with the cosine lines[0].set_data([x, np.cos(x)]) # Displaying the plot plt.show()
Output
On executing the above code we will get the following output −

Manual Addition of Artists
While many artists have helper methods, there are scenarios where manual addition is necessary. For instance, adding patches like circles or rectangles directly to an Axes object can be possible by using the add_artist() method.
Example
In this example, we manually add circle patches to the axes.
import matplotlib.pyplot as plt import numpy as np import matplotlib.patches as mpatches # Creating a simple plot fig, ax = plt.subplots(figsize=(7, 4)) x = np.linspace(0, 2 * np.pi, 100) # Initial sinusoidal curve y = np.sin(x) # Use the plot method to create a Line2D artist for a sinusoidal curve lines = ax.plot(x, y) # Adding a circle circle = mpatches.Circle((3, 0), 0.25, ec="none") ax.add_artist(circle) # Adding another clipped circle clipped_circle = mpatches.Circle((3, 1), 0.125, ec="none", facecolor='C1') ax.add_artist(clipped_circle) ax.set_aspect(1) # Adding a title to the plot plt.title('Manual Addition of Artists') # Displaying the plot plt.show()
Output
On executing the above code we will get the following output −

Removing Artists
Matplotlib provides a flexible way to remove specific artists from a plot. The remove() method of an artist allows for removing an Artist from its Axes list.
Example
Here is an example that demonstrates how to remove specific artists (Line2D and text) from the plot.
import numpy as np import matplotlib.pyplot as plt # Create the figure fig, ax = plt.subplots(figsize=(7, 4)) x = np.linspace(-10, 10, 100) y = np.sin(x) # plot the scatter plot scat = ax.scatter(x, y) # Assign the another Line2D artist to line_2 line_2 = plt.plot(x, np.cos(x), linestyle='dashed') plt.margins(0.2) plt.title("After removing the text and line artist") text = fig.text(0.5, 0.96, "$y=sin(x)$") # Remove the line_2 artist l = line_2.pop(0) l.remove() # Remove the text artist text.remove() # Displaying the plot plt.show()
Output
On executing the above code you will get the following output −
