Identifying Hub Genes and Metabolic Pathways in Collagen VI-Related Dystrophies: A Roadmap to Therapeutic Intervention
- PMID: 39595553
- PMCID: PMC11592009
- DOI: 10.3390/biom14111376
Identifying Hub Genes and Metabolic Pathways in Collagen VI-Related Dystrophies: A Roadmap to Therapeutic Intervention
Abstract
Collagen VI-related dystrophies (COL6RD) are a group of rare muscle disorders caused by mutations in specific genes responsible for type VI collagen production. It affects muscles, joints, and connective tissues, leading to weakness, joint problems, and structural issues. Currently, there is no effective treatment for COL6RD; its management typically addresses symptoms and complications. Therefore, it is essential to decipher the disease's molecular mechanisms, identify drug targets, and develop effective treatment strategies to treat COL6RD. In this study, we employed differential gene expression analysis, weighted gene co-expression network analysis, and genome-scale metabolic modeling to investigate gene expression patterns in COL6RD patients, uncovering key genes, significant metabolites, and disease-related pathophysiological pathways. First, we performed differential gene expression and weighted gene co-expression network analyses, which led to the identification of 12 genes (CHCHD10, MRPS24, TRIP10, RNF123, MRPS15, NDUFB4, COX10, FUNDC2, MDH2, RPL3L, NDUFB11, PARVB) as potential hub genes involved in the disease. Second, we utilized a drug repurposing strategy to identify pharmaceutical candidates that could potentially modulate these genes and be effective in the treatment. Next, we utilized context-specific genome-scale metabolic models to compare metabolic variations between healthy individuals and COL6RD patients. Finally, we conducted reporter metabolite analysis to identify reporter metabolites (e.g., phosphatidates, nicotinate ribonucleotide, ubiquinol, ferricytochrome C). In summary, our analysis revealed critical genes and pathways associated with COL6RD and identified potential targets, reporter metabolites, and candidate drugs for therapeutic interventions.
Keywords: collagen VI-related dystrophies; drug repurposing; genome-scale metabolic models; network analysis; systems biology.
Conflict of interest statement
A.M. and H.T. are the founders and shareholders of Trustlife Therapeutics, while the remaining authors report no conflicts of interest.
Figures
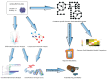
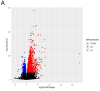
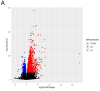
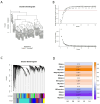
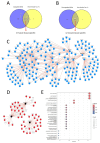
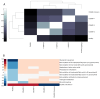
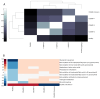
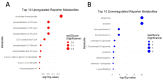
Similar articles
-
Depressing time: Waiting, melancholia, and the psychoanalytic practice of care.In: Kirtsoglou E, Simpson B, editors. The Time of Anthropology: Studies of Contemporary Chronopolitics. Abingdon: Routledge; 2020. Chapter 5. In: Kirtsoglou E, Simpson B, editors. The Time of Anthropology: Studies of Contemporary Chronopolitics. Abingdon: Routledge; 2020. Chapter 5. PMID: 36137063 Free Books & Documents. Review.
-
Unlocking data: Decision-maker perspectives on cross-sectoral data sharing and linkage as part of a whole-systems approach to public health policy and practice.Public Health Res (Southampt). 2024 Nov 20:1-30. doi: 10.3310/KYTW2173. Online ahead of print. Public Health Res (Southampt). 2024. PMID: 39582242
-
The interplay between dysregulated metabolites and signaling pathway alterations involved in osteoarthritis: a systematic review.Ther Adv Musculoskelet Dis. 2024 Nov 25;16:1759720X241299535. doi: 10.1177/1759720X241299535. eCollection 2024. Ther Adv Musculoskelet Dis. 2024. PMID: 39600593 Free PMC article. Review.
-
Genedrive kit for detecting single nucleotide polymorphism m.1555A>G in neonates and their mothers: a systematic review and cost-effectiveness analysis.Health Technol Assess. 2024 Oct;28(75):1-75. doi: 10.3310/TGAC4201. Health Technol Assess. 2024. PMID: 39487741 Free PMC article.
-
Pharmacological treatments in panic disorder in adults: a network meta-analysis.Cochrane Database Syst Rev. 2023 Nov 28;11(11):CD012729. doi: 10.1002/14651858.CD012729.pub3. Cochrane Database Syst Rev. 2023. PMID: 38014714 Free PMC article. Review.
References
-
- Muscular Dystrophy. NHS. [(accessed on 3 August 2024)]. Available online: https://www.nhs.uk/conditions/muscular-dystrophy/
-
- Topaloğlu H., Poorshiri B. The congenital muscular dystrophies. Ann. Child Neurol. Soc. 2024;2:27–39. doi: 10.1002/cns3.20050. - DOI
MeSH terms
Substances
Grants and funding
LinkOut - more resources
Full Text Sources
Medical
Miscellaneous