This is a preprint.
Integrating Multiplexed Imaging and Multiscale Modeling Identifies Tumor Phenotype Transformation as a Critical Component of Therapeutic T Cell Efficacy
- PMID: 38106218
- PMCID: PMC10723382
- DOI: 10.1101/2023.12.06.570168
Integrating Multiplexed Imaging and Multiscale Modeling Identifies Tumor Phenotype Transformation as a Critical Component of Therapeutic T Cell Efficacy
Update in
-
Integrating multiplexed imaging and multiscale modeling identifies tumor phenotype conversion as a critical component of therapeutic T cell efficacy.Cell Syst. 2024 Apr 17;15(4):322-338.e5. doi: 10.1016/j.cels.2024.03.004. Cell Syst. 2024. PMID: 38636457 Free PMC article.
Abstract
Cancer progression is a complex process involving interactions that unfold across molecular, cellular, and tissue scales. These multiscale interactions have been difficult to measure and to simulate. Here we integrated CODEX multiplexed tissue imaging with multiscale modeling software, to model key action points that influence the outcome of T cell therapies with cancer. The initial phenotype of therapeutic T cells influences the ability of T cells to convert tumor cells to an inflammatory, anti-proliferative phenotype. This T cell phenotype could be preserved by structural reprogramming to facilitate continual tumor phenotype conversion and killing. One takeaway is that controlling the rate of cancer phenotype conversion is critical for control of tumor growth. The results suggest new design criteria and patient selection metrics for T cell therapies, call for a rethinking of T cell therapeutic implementation, and provide a foundation for synergistically integrating multiplexed imaging data with multiscale modeling of the cancer-immune interface.
Keywords: Multiplexed tissue imaging; T cells; Vivarium; agent-based modeling; cellular therapy; computational analysis of tissue imaging data; immunotherapy; multiscale modeling; spatial biology; systems biology; theory experiment cycle; tumor cell phenotype.
Conflict of interest statement
DECLARATION OF INTERESTS GPN has equity in and is a scientific advisory board member of Akoya Biosciences, Inc. The other authors declare no competing interests.
Figures
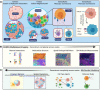
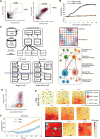
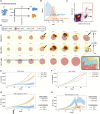
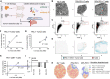
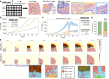
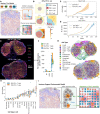
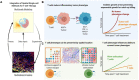
Similar articles
-
Integrating multiplexed imaging and multiscale modeling identifies tumor phenotype conversion as a critical component of therapeutic T cell efficacy.Cell Syst. 2024 Apr 17;15(4):322-338.e5. doi: 10.1016/j.cels.2024.03.004. Cell Syst. 2024. PMID: 38636457 Free PMC article.
-
Integrating single cell sequencing with a spatial quantitative systems pharmacology model spQSP for personalized prediction of triple-negative breast cancer immunotherapy response.Immunoinformatics (Amst). 2021 Oct;1-2:100002. doi: 10.1016/j.immuno.2021.100002. Epub 2021 Jul 24. Immunoinformatics (Amst). 2021. PMID: 34708216 Free PMC article.
-
Multiscale Agent-Based and Hybrid Modeling of the Tumor Immune Microenvironment.Processes (Basel). 2019 Jan;7(1):37. doi: 10.3390/pr7010037. Epub 2019 Jan 13. Processes (Basel). 2019. PMID: 30701168 Free PMC article.
-
Integrating Multiscale Modeling with Drug Effects for Cancer Treatment.Cancer Inform. 2016 Jan 13;14(Suppl 5):21-31. doi: 10.4137/CIN.S30797. eCollection 2015. Cancer Inform. 2016. PMID: 26792977 Free PMC article. Review.
-
Predictive oncology: a review of multidisciplinary, multiscale in silico modeling linking phenotype, morphology and growth.Neuroimage. 2007;37 Suppl 1(Suppl 1):S120-34. doi: 10.1016/j.neuroimage.2007.05.043. Epub 2007 Jun 7. Neuroimage. 2007. PMID: 17629503 Free PMC article. Review.
References
-
- Ahmed N., Brawley V.S., Hegde M., Robertson C., Ghazi A., Gerken C., Liu E., Dakhova O., Ashoori A., and Corder A. (2015). Human epidermal growth factor receptor 2 (HER2)–specific chimeric antigen receptor–modified T cells for the immunotherapy of HER2-positive sarcoma. J. Clin. Oncol. 33, 1688. - PMC - PubMed
-
- Bhate S.S., Barlow G.L., Schürch C.M., and Nolan G.P. (2021). Tissue schematics map the specialization of immune tissue motifs and their appropriation by tumors. Cell Syst. - PubMed
Publication types
Grants and funding
- UG3 DK114937/DK/NIDDK NIH HHS/United States
- U19 AI100627/AI/NIAID NIH HHS/United States
- R01 HL120724/HL/NHLBI NIH HHS/United States
- U01 AI101984/AI/NIAID NIH HHS/United States
- U54 HG010426/HG/NHGRI NIH HHS/United States
- 27145/CRUK_/Cancer Research UK/United Kingdom
- F99 CA212231/CA/NCI NIH HHS/United States
- U01 AI140498/AI/NIAID NIH HHS/United States
- T32 CA196585/CA/NCI NIH HHS/United States
- R33 CA183692/CA/NCI NIH HHS/United States
- U19 AI135976/AI/NIAID NIH HHS/United States
- P01 AI131374/AI/NIAID NIH HHS/United States
- U19 AI057229/AI/NIAID NIH HHS/United States
- U2C CA233238/CA/NCI NIH HHS/United States
- F32 CA233203/CA/NCI NIH HHS/United States
- F32 GM137464/GM/NIGMS NIH HHS/United States
- U2C CA233195/CA/NCI NIH HHS/United States
- R01 HL128173/HL/NHLBI NIH HHS/United States
LinkOut - more resources
Full Text Sources
Miscellaneous