Human mobility networks reveal increased segregation in large cities
- PMID: 38030732
- PMCID: PMC10733138
- DOI: 10.1038/s41586-023-06757-3
Human mobility networks reveal increased segregation in large cities
Abstract
A long-standing expectation is that large, dense and cosmopolitan areas support socioeconomic mixing and exposure among diverse individuals1-6. Assessing this hypothesis has been difficult because previous measures of socioeconomic mixing have relied on static residential housing data rather than real-life exposures among people at work, in places of leisure and in home neighbourhoods7,8. Here we develop a measure of exposure segregation that captures the socioeconomic diversity of these everyday encounters. Using mobile phone mobility data to represent 1.6 billion real-world exposures among 9.6 million people in the United States, we measure exposure segregation across 382 metropolitan statistical areas (MSAs) and 2,829 counties. We find that exposure segregation is 67% higher in the ten largest MSAs than in small MSAs with fewer than 100,000 residents. This means that, contrary to expectations, residents of large cosmopolitan areas have less exposure to a socioeconomically diverse range of individuals. Second, we find that the increased socioeconomic segregation in large cities arises because they offer a greater choice of differentiated spaces targeted to specific socioeconomic groups. Third, we find that this segregation-increasing effect is countered when a city's hubs (such as shopping centres) are positioned to bridge diverse neighbourhoods and therefore attract people of all socioeconomic statuses. Our findings challenge a long-standing conjecture in human geography and highlight how urban design can both prevent and facilitate encounters among diverse individuals.
© 2023. The Author(s).
Conflict of interest statement
The authors declare no competing interests.
Figures
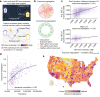
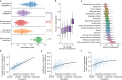
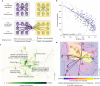
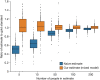
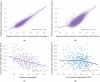
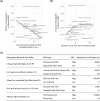
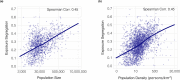
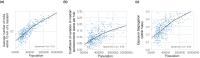
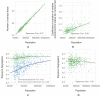
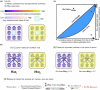
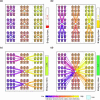
Similar articles
-
Estimating experienced racial segregation in US cities using large-scale GPS data.Proc Natl Acad Sci U S A. 2021 Nov 16;118(46):e2026160118. doi: 10.1073/pnas.2026160118. Proc Natl Acad Sci U S A. 2021. PMID: 34764221 Free PMC article.
-
Separate and Sick: Residential Segregation and the Health of Children and Youth in Metropolitan Statistical Areas.J Urban Health. 2019 Apr;96(2):149-158. doi: 10.1007/s11524-018-00330-4. J Urban Health. 2019. PMID: 30506135 Free PMC article.
-
Socioeconomic Segregation in Large Cities in France and the United States.Demography. 2016 Aug;53(4):1051-84. doi: 10.1007/s13524-016-0491-9. Demography. 2016. PMID: 27393232
-
Multi-Contextual Segregation and Environmental Justice Research: Toward Fine-Scale Spatiotemporal Approaches.Int J Environ Res Public Health. 2017 Oct 10;14(10):1205. doi: 10.3390/ijerph14101205. Int J Environ Res Public Health. 2017. PMID: 28994744 Free PMC article. Review.
-
Towards a new paradigm for segregation measurement in an age of big data.Urban Inform. 2022;1(1):5. doi: 10.1007/s44212-022-00003-3. Epub 2022 Sep 9. Urban Inform. 2022. PMID: 36124239 Free PMC article. Review.
Cited by
-
Pattern detection in the vehicular activity of bus rapid transit systems.PLoS One. 2024 Oct 29;19(10):e0312541. doi: 10.1371/journal.pone.0312541. eCollection 2024. PLoS One. 2024. PMID: 39471165 Free PMC article.
-
Disparities in Liver Transplant Outcomes: Race/Ethnicity and Individual- and Neighborhood-Level Socioeconomic Status.Clin Nurs Res. 2024 Sep;33(7):509-518. doi: 10.1177/10547738241273128. Epub 2024 Aug 27. Clin Nurs Res. 2024. PMID: 39192612 Free PMC article.
-
Predictability of human mobility during the COVID-19 pandemic in the United States.PNAS Nexus. 2024 Jul 26;3(8):pgae308. doi: 10.1093/pnasnexus/pgae308. eCollection 2024 Aug. PNAS Nexus. 2024. PMID: 39114577 Free PMC article.
-
Investigation on the coupling coordination of pseudo human settlements in the urban agglomerations in eastern China.Sci Rep. 2024 Jul 29;14(1):17402. doi: 10.1038/s41598-024-67851-8. Sci Rep. 2024. PMID: 39075083 Free PMC article.
-
Temporal dynamics of public transportation ridership in Seoul before, during, and after COVID-19 from urban resilience perspective.Sci Rep. 2024 Apr 18;14(1):8981. doi: 10.1038/s41598-024-59323-w. Sci Rep. 2024. PMID: 38637570 Free PMC article.
References
-
- Jacobs, J. The Death and Life of Great American Cities (Random House, 1961).
-
- Wirth, L. Urbanism as a way of life. Am. J. Sociol.44, 1–24 (1938).10.1086/217913 - DOI
-
- Gomez-Lievano, A., Patterson-Lomba, O. & Hausmann, R. Explaining the prevalence, scaling and variance of urban phenomena. Nat. Hum. Behav.1, 0012 (2016).10.1038/s41562-016-0012 - DOI
MeSH terms
Grants and funding
LinkOut - more resources
Full Text Sources