A transcription factor atlas of directed differentiation
- PMID: 36608654
- PMCID: PMC10344468
- DOI: 10.1016/j.cell.2022.11.026
A transcription factor atlas of directed differentiation
Erratum in
-
A transcription factor atlas of directed differentiation.Cell. 2024 Jun 6;187(12):3161. doi: 10.1016/j.cell.2024.04.038. Epub 2024 May 2. Cell. 2024. PMID: 38697106 No abstract available.
Abstract
Transcription factors (TFs) regulate gene programs, thereby controlling diverse cellular processes and cell states. To comprehensively understand TFs and the programs they control, we created a barcoded library of all annotated human TF splice isoforms (>3,500) and applied it to build a TF Atlas charting expression profiles of human embryonic stem cells (hESCs) overexpressing each TF at single-cell resolution. We mapped TF-induced expression profiles to reference cell types and validated candidate TFs for generation of diverse cell types, spanning all three germ layers and trophoblasts. Targeted screens with subsets of the library allowed us to create a tailored cellular disease model and integrate mRNA expression and chromatin accessibility data to identify downstream regulators. Finally, we characterized the effects of combinatorial TF overexpression by developing and validating a strategy for predicting combinations of TFs that produce target expression profiles matching reference cell types to accelerate cellular engineering efforts.
Keywords: ORF overexpression; cell engineering; cellular disease modeling; combinatorial perturbation; gene regulation; genetic screening; neural progenitor; pluripotent stem cell; single cell profiling; transcription factor.
Copyright © 2022 The Authors. Published by Elsevier Inc. All rights reserved.
Conflict of interest statement
Declaration of interests J.J. and F.Z. are inventors listed on an International PCT Application related to this work. F.Z. is a scientific advisor and co-founder of Editas Medicine, Beam Therapeutics, Pairwise Plants, Arbor Biotechnologies, and Proof Diagnostics. F.Z. is a scientific advisor for Octant. A.R. is a co-founder and equity holder of Celsius Therapeutics, an equity holder in Immunitas, and was an SAB member of ThermoFisher Scientific, Syros Pharmaceuticals, Neogene Therapeutics, and Asimov until 31 July 2020. Since 1 August 2020, A.R. has been an employee of Genentech and has equity in Roche. A.R. is an inventor on patents and patent applications filed at the Board related to single-cell genomics. J.D.B. holds patents related to ATAC-seq and scATAC-seq and serves on the scientific advisory boards of CAMP4 Therapeutics, seqWell, and CelSee. J.S.G. and O.O.A. are cofounders of Sherlock Biosciences, Proof Diagnostics, Moment Biosciences, and Tome Biosciences. Since 16 November 2020, K.R.G.-S. has been an employee of Genentech.
Figures
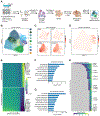
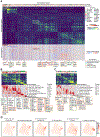
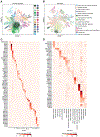
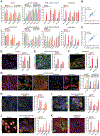
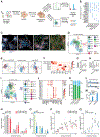
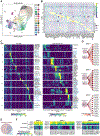
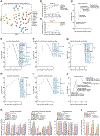
Similar articles
-
Integrated transcriptomic and regulatory network analyses identify microRNA-200c as a novel repressor of human pluripotent stem cell-derived cardiomyocyte differentiation and maturation.Cardiovasc Res. 2018 May 1;114(6):894-906. doi: 10.1093/cvr/cvy019. Cardiovasc Res. 2018. PMID: 29373717
-
Generation and Profiling of 2,135 Human ESC Lines for the Systematic Analyses of Cell States Perturbed by Inducing Single Transcription Factors.Cell Rep. 2020 May 19;31(7):107655. doi: 10.1016/j.celrep.2020.107655. Cell Rep. 2020. PMID: 32433964
-
Atlas of regulated target genes of transcription factors (ART-TF) in human ES cells.BMC Bioinformatics. 2022 Sep 16;23(1):377. doi: 10.1186/s12859-022-04924-3. BMC Bioinformatics. 2022. PMID: 36114445 Free PMC article.
-
Transcription factors: Bridge between cell signaling and gene regulation.Proteomics. 2021 Dec;21(23-24):e2000034. doi: 10.1002/pmic.202000034. Epub 2021 Aug 9. Proteomics. 2021. PMID: 34314098 Review.
-
Molecular biology of the stress response in the early embryo and its stem cells.Adv Exp Med Biol. 2015;843:77-128. doi: 10.1007/978-1-4939-2480-6_4. Adv Exp Med Biol. 2015. PMID: 25956296 Review.
Cited by
-
DNA methylation-activated full-length EMX1 facilitates metastasis through EMX1-EGFR-ERK axis in hepatocellular carcinoma.Cell Death Dis. 2023 Nov 25;14(11):769. doi: 10.1038/s41419-023-06293-y. Cell Death Dis. 2023. PMID: 38007497 Free PMC article.
-
Allosteric regulation by c-di-AMP modulates a complete N-acetylglucosamine signaling cascade in Saccharopolyspora erythraea.Nat Commun. 2024 May 7;15(1):3825. doi: 10.1038/s41467-024-48063-0. Nat Commun. 2024. PMID: 38714645 Free PMC article.
-
Single-cell multiomics guided mechanistic understanding of Fontan-associated liver disease.Sci Transl Med. 2024 Apr 24;16(744):eadk6213. doi: 10.1126/scitranslmed.adk6213. Epub 2024 Apr 24. Sci Transl Med. 2024. PMID: 38657025 Free PMC article.
-
Decoding biology with massively parallel reporter assays and machine learning.Genes Dev. 2024 Oct 16;38(17-20):843-865. doi: 10.1101/gad.351800.124. Genes Dev. 2024. PMID: 39362779 Free PMC article. Review.
-
Hold out the genome: a roadmap to solving the cis-regulatory code.Nature. 2024 Jan;625(7993):41-50. doi: 10.1038/s41586-023-06661-w. Epub 2023 Dec 13. Nature. 2024. PMID: 38093018 Review.
References
Publication types
MeSH terms
Substances
Grants and funding
LinkOut - more resources
Full Text Sources
Other Literature Sources
Molecular Biology Databases
Research Materials
Miscellaneous