Spatial profiling of chromatin accessibility in mouse and human tissues
- PMID: 35978191
- PMCID: PMC9452302
- DOI: 10.1038/s41586-022-05094-1
Spatial profiling of chromatin accessibility in mouse and human tissues
Abstract
Cellular function in tissue is dependent on the local environment, requiring new methods for spatial mapping of biomolecules and cells in the tissue context1. The emergence of spatial transcriptomics has enabled genome-scale gene expression mapping2-5, but the ability to capture spatial epigenetic information of tissue at the cellular level and genome scale is lacking. Here we describe a method for spatially resolved chromatin accessibility profiling of tissue sections using next-generation sequencing (spatial-ATAC-seq) by combining in situ Tn5 transposition chemistry6 and microfluidic deterministic barcoding5. Profiling mouse embryos using spatial-ATAC-seq delineated tissue-region-specific epigenetic landscapes and identified gene regulators involved in the development of the central nervous system. Mapping the accessible genome in the mouse and human brain revealed the intricate arealization of brain regions. Applying spatial-ATAC-seq to tonsil tissue resolved the spatially distinct organization of immune cell types and states in lymphoid follicles and extrafollicular zones. This technology progresses spatial biology by enabling spatially resolved chromatin accessibility profiling to improve our understanding of cell identity, cell state and cell fate decision in relation to epigenetic underpinnings in development and disease.
© 2022. The Author(s).
Conflict of interest statement
R.F. and Y.D. are inventors of a patent application related to this work (PCT Patent Application No. PCT/US2021/065669). R.F. is scientific founder and advisor of IsoPlexis, Singleron Biotechnologies and AtlasXomics. The interests of R.F. were reviewed and managed by Yale University Provost’s Office in accordance with the University’s conflict of interest policies. The other authors declare no competing interests.
Figures
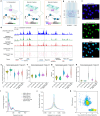
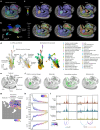
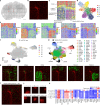
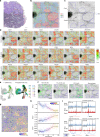
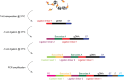
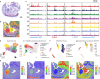
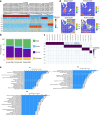
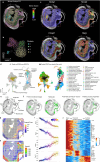
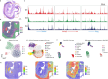
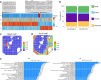
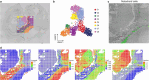
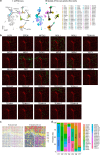
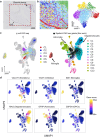
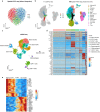
Similar articles
-
Spatially resolved epigenome sequencing via Tn5 transposition and deterministic DNA barcoding in tissue.Nat Protoc. 2024 Jun 28. doi: 10.1038/s41596-024-01013-y. Online ahead of print. Nat Protoc. 2024. PMID: 38943021 Review.
-
Chromatin accessibility profiling of targeted cell populations with laser capture microdissection coupled to ATAC-seq.Cell Rep Methods. 2023 Oct 23;3(10):100598. doi: 10.1016/j.crmeth.2023.100598. Epub 2023 Sep 29. Cell Rep Methods. 2023. PMID: 37776856 Free PMC article.
-
Solid-phase capture and profiling of open chromatin by spatial ATAC.Nat Biotechnol. 2023 Aug;41(8):1085-1088. doi: 10.1038/s41587-022-01603-9. Epub 2023 Jan 5. Nat Biotechnol. 2023. PMID: 36604544 Free PMC article.
-
Spatial-CUT&Tag: Spatially resolved chromatin modification profiling at the cellular level.Science. 2022 Feb 11;375(6581):681-686. doi: 10.1126/science.abg7216. Epub 2022 Feb 10. Science. 2022. PMID: 35143307 Free PMC article.
-
[Advances in assay for transposase-accessible chromatin with high-throughput sequencing].Yi Chuan. 2020 Apr 20;42(4):333-346. doi: 10.16288/j.yczz.19-279. Yi Chuan. 2020. PMID: 32312702 Review. Chinese.
Cited by
-
Seq-Scope: repurposing Illumina sequencing flow cells for high-resolution spatial transcriptomics.Nat Protoc. 2024 Oct 31. doi: 10.1038/s41596-024-01065-0. Online ahead of print. Nat Protoc. 2024. PMID: 39482362 Review.
-
Translation of genome-wide association study: from genomic signals to biological insights.Front Genet. 2024 Oct 3;15:1375481. doi: 10.3389/fgene.2024.1375481. eCollection 2024. Front Genet. 2024. PMID: 39421299 Free PMC article. Review.
-
Epigenomic heterogeneity as a source of tumour evolution.Nat Rev Cancer. 2024 Oct 16. doi: 10.1038/s41568-024-00757-9. Online ahead of print. Nat Rev Cancer. 2024. PMID: 39414948 Review.
-
Improved cell-type identification and comprehensive mapping of regulatory features with spatial epigenomics 96-channel microfluidic platform.GEN Biotechnol. 2023 Dec;2(6):503-514. doi: 10.1089/genbio.2023.0044. Epub 2023 Dec 15. GEN Biotechnol. 2023. PMID: 39380764 Free PMC article.
-
Multiplexed spatial mapping of chromatin features, transcriptome, and proteins in tissues.bioRxiv [Preprint]. 2024 Sep 19:2024.09.13.612892. doi: 10.1101/2024.09.13.612892. bioRxiv. 2024. PMID: 39345645 Free PMC article. Preprint.
References
MeSH terms
Substances
Grants and funding
LinkOut - more resources
Full Text Sources
Other Literature Sources