scSTEM: clustering pseudotime ordered single-cell data
- PMID: 35799304
- PMCID: PMC9264648
- DOI: 10.1186/s13059-022-02716-9
scSTEM: clustering pseudotime ordered single-cell data
Abstract
We develop scSTEM, single-cell STEM, a method for clustering dynamic profiles of genes in trajectories inferred from pseudotime ordering of single-cell RNA-seq (scRNA-seq) data. scSTEM uses one of several metrics to summarize the expression of genes and assigns a p-value to clusters enabling the identification of significant profiles and comparison of profiles across different paths. Application of scSTEM to several scRNA-seq datasets demonstrates its usefulness and ability to improve downstream analysis of biological processes. scSTEM is available at https://github.com/alexQiSong/scSTEM .
Keywords: Gene clustering; Genomics; Single cell; Visualization.
© 2022. The Author(s).
Conflict of interest statement
The authors declare that they have no competing interests.
Figures
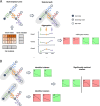
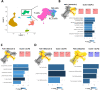
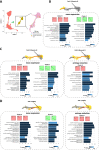
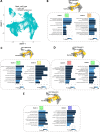
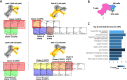
Similar articles
-
scBGEDA: deep single-cell clustering analysis via a dual denoising autoencoder with bipartite graph ensemble clustering.Bioinformatics. 2023 Feb 14;39(2):btad075. doi: 10.1093/bioinformatics/btad075. Bioinformatics. 2023. PMID: 36734596 Free PMC article.
-
A component overlapping attribute clustering (COAC) algorithm for single-cell RNA sequencing data analysis and potential pathobiological implications.PLoS Comput Biol. 2019 Feb 19;15(2):e1006772. doi: 10.1371/journal.pcbi.1006772. eCollection 2019 Feb. PLoS Comput Biol. 2019. PMID: 30779739 Free PMC article.
-
scHFC: a hybrid fuzzy clustering method for single-cell RNA-seq data optimized by natural computation.Brief Bioinform. 2022 Mar 10;23(2):bbab588. doi: 10.1093/bib/bbab588. Brief Bioinform. 2022. PMID: 35136924
-
Clustering scRNA-seq data with the cross-view collaborative information fusion strategy.Brief Bioinform. 2024 Sep 23;25(6):bbae511. doi: 10.1093/bib/bbae511. Brief Bioinform. 2024. PMID: 39402696 Free PMC article.
-
Machine learning and statistical methods for clustering single-cell RNA-sequencing data.Brief Bioinform. 2020 Jul 15;21(4):1209-1223. doi: 10.1093/bib/bbz063. Brief Bioinform. 2020. PMID: 31243426 Review.
Cited by
-
Single-cell genomics and regulatory networks for 388 human brains.Science. 2024 May 24;384(6698):eadi5199. doi: 10.1126/science.adi5199. Epub 2024 May 24. Science. 2024. PMID: 38781369 Free PMC article.
-
Single-cell genomics and regulatory networks for 388 human brains.bioRxiv [Preprint]. 2024 Mar 30:2024.03.18.585576. doi: 10.1101/2024.03.18.585576. bioRxiv. 2024. Update in: Science. 2024 May 24;384(6698):eadi5199. doi: 10.1126/science.adi5199. PMID: 38562822 Free PMC article. Updated. Preprint.
-
Spatial mapping of cellular senescence: emerging challenges and opportunities.Nat Aging. 2023 Jul;3(7):776-790. doi: 10.1038/s43587-023-00446-6. Epub 2023 Jul 3. Nat Aging. 2023. PMID: 37400722 Free PMC article. Review.
-
CellTracer: a comprehensive database to dissect the causative multilevel interplay contributing to cell development trajectories.Nucleic Acids Res. 2023 Jan 6;51(D1):D861-D869. doi: 10.1093/nar/gkac892. Nucleic Acids Res. 2023. PMID: 36243976 Free PMC article.
References
Publication types
MeSH terms
Grants and funding
LinkOut - more resources
Full Text Sources