Computational tools for analyzing single-cell data in pluripotent cell differentiation studies
- PMID: 35474899
- PMCID: PMC9017169
- DOI: 10.1016/j.crmeth.2021.100087
Computational tools for analyzing single-cell data in pluripotent cell differentiation studies
Abstract
Single-cell technologies are revolutionizing the ability of researchers to infer the causes and results of biological processes. Although several studies of pluripotent cell differentiation have recently utilized single-cell sequencing data, other aspects related to the optimization of differentiation protocols, their validation, robustness, and usage are still not taking full advantage of single-cell technologies. In this review, we focus on computational approaches for the analysis of single-cell omics and imaging data and discuss their use to address many of the major challenges involved in the development, validation, and use of cells obtained from pluripotent cell differentiation.
Keywords: computational approaches; pluripotent cell differentiation; protocol optimization; single cell; stem cell applications.
© 2021 The Authors.
Conflict of interest statement
The authors declare no competing interests.
Figures
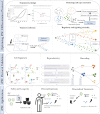
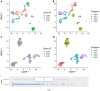
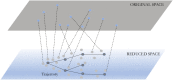
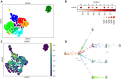
Similar articles
-
In Vitro Differentiation of Pluripotent Stem Cells into Functional β Islets Under 2D and 3D Culture Conditions and In Vivo Preclinical Validation of 3D Islets.Methods Mol Biol. 2016;1341:257-84. doi: 10.1007/7651_2015_230. Methods Mol Biol. 2016. PMID: 25783769
-
Proteomic and Glyco(proteo)mic tools in the profiling of cardiac progenitors and pluripotent stem cell derived cardiomyocytes: Accelerating translation into therapy.Biotechnol Adv. 2021 Jul-Aug;49:107755. doi: 10.1016/j.biotechadv.2021.107755. Epub 2021 Apr 22. Biotechnol Adv. 2021. PMID: 33895330 Review.
-
A data analysis framework for biomedical big data: Application on mesoderm differentiation of human pluripotent stem cells.PLoS One. 2017 Jun 27;12(6):e0179613. doi: 10.1371/journal.pone.0179613. eCollection 2017. PLoS One. 2017. PMID: 28654683 Free PMC article.
-
Pluripotent stem cells for skeletal tissue engineering.Crit Rev Biotechnol. 2022 Aug;42(5):774-793. doi: 10.1080/07388551.2021.1968785. Epub 2021 Sep 6. Crit Rev Biotechnol. 2022. PMID: 34488516 Review.
-
Convergence of human pluripotent stem cell, organoid, and genome editing technologies.Exp Biol Med (Maywood). 2021 Apr;246(7):861-875. doi: 10.1177/1535370220985808. Epub 2021 Jan 19. Exp Biol Med (Maywood). 2021. PMID: 33467883 Free PMC article. Review.
Cited by
-
Therapeutic Cell Repopulation of the Liver: From Fetal Rat Cells to Synthetic Human Tissues.Cells. 2023 Feb 6;12(4):529. doi: 10.3390/cells12040529. Cells. 2023. PMID: 36831196 Free PMC article. Review.
-
New Insights into Cerebral Vessel Disease Landscapes at Single-Cell Resolution: Pathogenetic and Therapeutic Perspectives.Biomedicines. 2022 Jul 13;10(7):1693. doi: 10.3390/biomedicines10071693. Biomedicines. 2022. PMID: 35884997 Free PMC article. Review.
References
Publication types
MeSH terms
Grants and funding
LinkOut - more resources
Full Text Sources