Deep learning and alignment of spatially resolved single-cell transcriptomes with Tangram
- PMID: 34711971
- PMCID: PMC8566243
- DOI: 10.1038/s41592-021-01264-7
Deep learning and alignment of spatially resolved single-cell transcriptomes with Tangram
Abstract
Charting an organs' biological atlas requires us to spatially resolve the entire single-cell transcriptome, and to relate such cellular features to the anatomical scale. Single-cell and single-nucleus RNA-seq (sc/snRNA-seq) can profile cells comprehensively, but lose spatial information. Spatial transcriptomics allows for spatial measurements, but at lower resolution and with limited sensitivity. Targeted in situ technologies solve both issues, but are limited in gene throughput. To overcome these limitations we present Tangram, a method that aligns sc/snRNA-seq data to various forms of spatial data collected from the same region, including MERFISH, STARmap, smFISH, Spatial Transcriptomics (Visium) and histological images. Tangram can map any type of sc/snRNA-seq data, including multimodal data such as those from SHARE-seq, which we used to reveal spatial patterns of chromatin accessibility. We demonstrate Tangram on healthy mouse brain tissue, by reconstructing a genome-wide anatomically integrated spatial map at single-cell resolution of the visual and somatomotor areas.
© 2021. The Author(s).
Conflict of interest statement
A.R. is a cofounder and an equity holder of Celsius Therapeutics and an equity holder of Immunitas, and was a scientific advisory board member of ThermoFisher Scientific, Syros Pharmaceuticals, Neogene Therapeutics and Asimov. From 1 August 2020, A.R. has been an employee of Genentech. From 1 January 2021, G.S. has been an employee of Roche. From 1 February 2021, T.B. has been an employee of Genentech. X.Z. is a cofounder of and consultant for Vizgen.
Figures
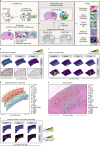
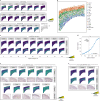
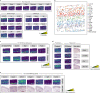
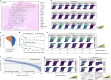
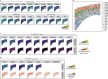
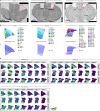
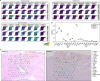
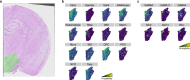
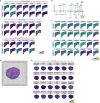
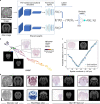
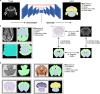
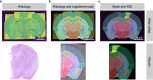
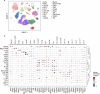
Comment in
-
Integrative analysis methods for spatial transcriptomics.Nat Methods. 2021 Nov;18(11):1282-1283. doi: 10.1038/s41592-021-01272-7. Nat Methods. 2021. PMID: 34711969 No abstract available.
Similar articles
-
Computational solutions for spatial transcriptomics.Comput Struct Biotechnol J. 2022 Sep 1;20:4870-4884. doi: 10.1016/j.csbj.2022.08.043. eCollection 2022. Comput Struct Biotechnol J. 2022. PMID: 36147664 Free PMC article. Review.
-
Concordance of MERFISH spatial transcriptomics with bulk and single-cell RNA sequencing.Life Sci Alliance. 2022 Dec 16;6(1):e202201701. doi: 10.26508/lsa.202201701. Print 2023 Jan. Life Sci Alliance. 2022. PMID: 36526371 Free PMC article.
-
Comparative Methods for Demystifying Spatial Transcriptomics.Methods Mol Biol. 2024;2802:515-546. doi: 10.1007/978-1-0716-3838-5_17. Methods Mol Biol. 2024. PMID: 38819570
-
Reference-based cell type matching of in situ image-based spatial transcriptomics data on primary visual cortex of mouse brain.Sci Rep. 2023 Jun 13;13(1):9567. doi: 10.1038/s41598-023-36638-8. Sci Rep. 2023. PMID: 37311768 Free PMC article.
-
Recent advances in spatially resolved transcriptomics: challenges and opportunities.BMB Rep. 2022 Mar;55(3):113-124. doi: 10.5483/BMBRep.2022.55.3.014. BMB Rep. 2022. PMID: 35168703 Free PMC article. Review.
Cited by
-
Integrative spatial and genomic analysis of tumor heterogeneity with Tumoroscope.Nat Commun. 2024 Oct 29;15(1):9343. doi: 10.1038/s41467-024-53374-3. Nat Commun. 2024. PMID: 39472583 Free PMC article.
-
STASCAN deciphers fine-resolution cell distribution maps in spatial transcriptomics by deep learning.Genome Biol. 2024 Oct 22;25(1):278. doi: 10.1186/s13059-024-03421-5. Genome Biol. 2024. PMID: 39439006 Free PMC article.
-
A spatiotemporal transcriptomic atlas of mouse placentation.Cell Discov. 2024 Oct 22;10(1):110. doi: 10.1038/s41421-024-00740-6. Cell Discov. 2024. PMID: 39438452 Free PMC article.
-
SELF-Former: multi-scale gene filtration transformer for single-cell spatial reconstruction.Brief Bioinform. 2024 Sep 23;25(6):bbae523. doi: 10.1093/bib/bbae523. Brief Bioinform. 2024. PMID: 39413798 Free PMC article.
-
SDePER: a hybrid machine learning and regression method for cell-type deconvolution of spatial barcoding-based transcriptomic data.Genome Biol. 2024 Oct 14;25(1):271. doi: 10.1186/s13059-024-03416-2. Genome Biol. 2024. PMID: 39402626 Free PMC article.
References
-
- Regev, A. et al. The Human Cell Atlas white paper. Preprint at https://arxiv.org/abs/1810.05192 (2018).
Publication types
MeSH terms
Substances
Grants and funding
LinkOut - more resources
Full Text Sources
Other Literature Sources