Deep learning of gene relationships from single cell time-course expression data
- PMID: 33876191
- PMCID: PMC8425306
- DOI: 10.1093/bib/bbab142
Deep learning of gene relationships from single cell time-course expression data
Abstract
Time-course gene-expression data have been widely used to infer regulatory and signaling relationships between genes. Most of the widely used methods for such analysis were developed for bulk expression data. Single cell RNA-Seq (scRNA-Seq) data offer several advantages including the large number of expression profiles available and the ability to focus on individual cells rather than averages. However, the data also raise new computational challenges. Using a novel encoding for scRNA-Seq expression data, we develop deep learning methods for interaction prediction from time-course data. Our methods use a supervised framework which represents the data as 3D tensor and train convolutional and recurrent neural networks for predicting interactions. We tested our time-course deep learning (TDL) models on five different time-series scRNA-Seq datasets. As we show, TDL can accurately identify causal and regulatory gene-gene interactions and can also be used to assign new function to genes. TDL improves on prior methods for the above tasks and can be generally applied to new time-series scRNA-Seq data.
Keywords: deep learning; single cell RNA-Seq; time-course data.
© The Author(s) 2021. Published by Oxford University Press. All rights reserved. For Permissions, please email: journals.permissions@oup.com.
Figures
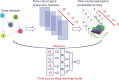
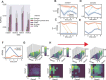
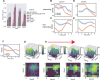
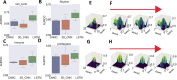
Similar articles
-
DeepGRNCS: deep learning-based framework for jointly inferring gene regulatory networks across cell subpopulations.Brief Bioinform. 2024 May 23;25(4):bbae334. doi: 10.1093/bib/bbae334. Brief Bioinform. 2024. PMID: 38980373 Free PMC article.
-
Deep embedded clustering with multiple objectives on scRNA-seq data.Brief Bioinform. 2021 Sep 2;22(5):bbab090. doi: 10.1093/bib/bbab090. Brief Bioinform. 2021. PMID: 33822877
-
SFINN: inferring gene regulatory network from single-cell and spatial transcriptomic data with shared factor neighborhood and integrated neural network.Bioinformatics. 2024 Jul 1;40(7):btae433. doi: 10.1093/bioinformatics/btae433. Bioinformatics. 2024. PMID: 38950180 Free PMC article.
-
Biological and Medical Importance of Cellular Heterogeneity Deciphered by Single-Cell RNA Sequencing.Cells. 2020 Jul 22;9(8):1751. doi: 10.3390/cells9081751. Cells. 2020. PMID: 32707839 Free PMC article. Review.
-
Application of Deep Learning on Single-cell RNA Sequencing Data Analysis: A Review.Genomics Proteomics Bioinformatics. 2022 Oct;20(5):814-835. doi: 10.1016/j.gpb.2022.11.011. Epub 2022 Dec 14. Genomics Proteomics Bioinformatics. 2022. PMID: 36528240 Free PMC article. Review.
Cited by
-
CRISPR-GEM: A Novel Machine Learning Model for CRISPR Genetic Target Discovery and Evaluation.ACS Synth Biol. 2024 Oct 18;13(10):3413-3429. doi: 10.1021/acssynbio.4c00473. Epub 2024 Oct 7. ACS Synth Biol. 2024. PMID: 39375864 Free PMC article.
-
CVGAE: A Self-Supervised Generative Method for Gene Regulatory Network Inference Using Single-Cell RNA Sequencing Data.Interdiscip Sci. 2024 Dec;16(4):990-1004. doi: 10.1007/s12539-024-00633-y. Epub 2024 May 23. Interdiscip Sci. 2024. PMID: 38778003
-
GMFGRN: a matrix factorization and graph neural network approach for gene regulatory network inference.Brief Bioinform. 2024 Jan 22;25(2):bbad529. doi: 10.1093/bib/bbad529. Brief Bioinform. 2024. PMID: 38261340
-
Depicting pseudotime-lagged causality across single-cell trajectories for accurate gene-regulatory inference.PNAS Nexus. 2023 Mar 30;2(4):pgad113. doi: 10.1093/pnasnexus/pgad113. eCollection 2023 Apr. PNAS Nexus. 2023. PMID: 37113980 Free PMC article.
-
STGRNS: an interpretable transformer-based method for inferring gene regulatory networks from single-cell transcriptomic data.Bioinformatics. 2023 Apr 3;39(4):btad165. doi: 10.1093/bioinformatics/btad165. Bioinformatics. 2023. PMID: 37004161 Free PMC article.
References
-
- Stuart JM, Segal E, Koller D, et al. . A gene-coexpression network for global discovery of conserved genetic modules. Science 2003;302:249–55. - PubMed
Publication types
MeSH terms
Grants and funding
LinkOut - more resources
Full Text Sources
Other Literature Sources
Research Materials