Cell lineage inference from SNP and scRNA-Seq data
- PMID: 30820578
- PMCID: PMC6547431
- DOI: 10.1093/nar/gkz146
Cell lineage inference from SNP and scRNA-Seq data
Abstract
Several recent studies focus on the inference of developmental and response trajectories from single cell RNA-Seq (scRNA-Seq) data. A number of computational methods, often referred to as pseudo-time ordering, have been developed for this task. Recently, CRISPR has also been used to reconstruct lineage trees by inserting random mutations. However, both approaches suffer from drawbacks that limit their use. Here, we develop a method to detect significant, cell type specific, sequence mutations from scRNA-Seq data. We show that only a few mutations are enough for reconstructing good branching models. Integrating these mutations with expression data further improves the accuracy of the reconstructed models. As we show, the majority of mutations we identify are likely RNA editing events indicating that such information can be used to distinguish cell types.
© The Author(s) 2019. Published by Oxford University Press on behalf of Nucleic Acids Research.
Figures
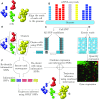
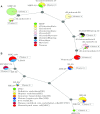
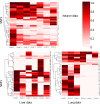
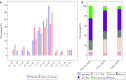
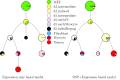
Similar articles
-
LINEAGE: Label-free identification of endogenous informative single-cell mitochondrial RNA mutation for lineage analysis.Proc Natl Acad Sci U S A. 2022 Feb 1;119(5):e2119767119. doi: 10.1073/pnas.2119767119. Proc Natl Acad Sci U S A. 2022. PMID: 35086932 Free PMC article.
-
Data Analysis in Single-Cell Transcriptome Sequencing.Methods Mol Biol. 2018;1754:311-326. doi: 10.1007/978-1-4939-7717-8_18. Methods Mol Biol. 2018. PMID: 29536451
-
An accessible, interactive GenePattern Notebook for analysis and exploration of single-cell transcriptomic data.F1000Res. 2018 Aug 16;7:1306. doi: 10.12688/f1000research.15830.2. eCollection 2018. F1000Res. 2018. PMID: 31316748 Free PMC article.
-
Computational approaches for interpreting scRNA-seq data.FEBS Lett. 2017 Aug;591(15):2213-2225. doi: 10.1002/1873-3468.12684. Epub 2017 Jun 12. FEBS Lett. 2017. PMID: 28524227 Free PMC article. Review.
-
Design and computational analysis of single-cell RNA-sequencing experiments.Genome Biol. 2016 Apr 7;17:63. doi: 10.1186/s13059-016-0927-y. Genome Biol. 2016. PMID: 27052890 Free PMC article. Review.
Cited by
-
Benchmarking bulk and single-cell variant-calling approaches on Chromium scRNA-seq and scATAC-seq libraries.Genome Res. 2024 Sep 20;34(8):1196-1210. doi: 10.1101/gr.277066.122. Genome Res. 2024. PMID: 39147582
-
Critical transition and reversion of tumorigenesis.Exp Mol Med. 2023 Apr;55(4):692-705. doi: 10.1038/s12276-023-00969-3. Epub 2023 Apr 3. Exp Mol Med. 2023. PMID: 37009794 Free PMC article. Review.
-
Recurrent RNA edits in human preimplantation potentially enhance maternal mRNA clearance.Commun Biol. 2022 Dec 21;5(1):1400. doi: 10.1038/s42003-022-04338-0. Commun Biol. 2022. PMID: 36543858 Free PMC article.
-
Simultaneous brain cell type and lineage determined by scRNA-seq reveals stereotyped cortical development.Cell Syst. 2022 Jun 15;13(6):438-453.e5. doi: 10.1016/j.cels.2022.03.006. Epub 2022 Apr 21. Cell Syst. 2022. PMID: 35452605 Free PMC article.
-
LINEAGE: Label-free identification of endogenous informative single-cell mitochondrial RNA mutation for lineage analysis.Proc Natl Acad Sci U S A. 2022 Feb 1;119(5):e2119767119. doi: 10.1073/pnas.2119767119. Proc Natl Acad Sci U S A. 2022. PMID: 35086932 Free PMC article.
References
Publication types
MeSH terms
Grants and funding
LinkOut - more resources
Full Text Sources
Research Materials