A long-context language model for deciphering and generating bacteriophage genomes
- PMID: 39477977
- PMCID: PMC11525655
- DOI: 10.1038/s41467-024-53759-4
A long-context language model for deciphering and generating bacteriophage genomes
Abstract
Inspired by the success of large language models (LLMs), we develop a long-context generative model for genomes. Our multiscale transformer model, megaDNA, is pre-trained on unannotated bacteriophage genomes with nucleotide-level tokenization. We demonstrate the foundational capabilities of our model including the prediction of essential genes, genetic variant effects, regulatory element activity and taxonomy of unannotated sequences. Furthermore, it generates de novo sequences up to 96 K base pairs, which contain potential regulatory elements and annotated proteins with phage-related functions.
© 2024. The Author(s).
Conflict of interest statement
The authors declare no competing interests.
Figures
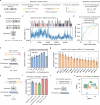
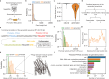
Similar articles
-
Transformer model generated bacteriophage genomes are compositionally distinct from natural sequences.NAR Genom Bioinform. 2024 Sep 18;6(3):lqae129. doi: 10.1093/nargab/lqae129. eCollection 2024 Sep. NAR Genom Bioinform. 2024. PMID: 39296932 Free PMC article.
-
In silico characterization of DNA motifs with particular reference to promoters and terminators.Methods Mol Biol. 2009;502:113-29. doi: 10.1007/978-1-60327-565-1_8. Methods Mol Biol. 2009. PMID: 19082554
-
DeepPL: A deep-learning-based tool for the prediction of bacteriophage lifecycle.PLoS Comput Biol. 2024 Oct 17;20(10):e1012525. doi: 10.1371/journal.pcbi.1012525. eCollection 2024 Oct. PLoS Comput Biol. 2024. PMID: 39418300 Free PMC article.
-
A Bioinformatic Ecosystem for Bacteriophage Genomics: PhaMMSeqs, Phamerator, pdm_utils, PhagesDB, DEPhT, and PhamClust.Viruses. 2024 Aug 10;16(8):1278. doi: 10.3390/v16081278. Viruses. 2024. PMID: 39205252 Free PMC article. Review.
-
Analysis of the phage sequence space: the benefit of structured information.Virology. 2007 Sep 1;365(2):241-9. doi: 10.1016/j.virol.2007.03.047. Epub 2007 May 7. Virology. 2007. PMID: 17482656 Review.
Cited by
-
The design and engineering of synthetic genomes.Nat Rev Genet. 2024 Nov 6. doi: 10.1038/s41576-024-00786-y. Online ahead of print. Nat Rev Genet. 2024. PMID: 39506144 Review.
-
Protein Set Transformer: A protein-based genome language model to power high diversity viromics.Res Sq [Preprint]. 2024 Sep 23:rs.3.rs-4844047. doi: 10.21203/rs.3.rs-4844047/v1. Res Sq. 2024. PMID: 39399683 Free PMC article. Preprint.
-
Synthetic genomes unveil the effects of synonymous recoding.bioRxiv [Preprint]. 2024 Jun 16:2024.06.16.599206. doi: 10.1101/2024.06.16.599206. bioRxiv. 2024. PMID: 38915524 Free PMC article. Preprint.
-
Protein Set Transformer: A protein-based genome language model to power high diversity viromics.bioRxiv [Preprint]. 2024 Jul 29:2024.07.26.605391. doi: 10.1101/2024.07.26.605391. bioRxiv. 2024. PMID: 39131363 Free PMC article. Preprint.
-
Evaluating the representational power of pre-trained DNA language models for regulatory genomics.bioRxiv [Preprint]. 2024 Sep 25:2024.02.29.582810. doi: 10.1101/2024.02.29.582810. bioRxiv. 2024. PMID: 38464101 Free PMC article. Preprint.
References
-
- Devlin, J., Chang, M.-W., Lee, K. & Toutanova, K. Bert: pre-training of deep bidirectional transformers for language understanding. Preprint at arXiv10.48550/arXiv.1810.04805 (2018).
-
- Brown, T. et al. Language models are few-shot learners. Adv. Neural Inf. Process. Syst.33, 1877–1901 (2020).
-
- Benegas, G., Ye, C., Albors, C., Li, J. C. & Song, Y. S. Genomic language models: opportunities and challenges. Preprint at arXiv10.48550/arXiv.2407.11435 (2024).
-
- Dalla-Torre, H. et al. The nucleotide transformer: building and evaluating robust foundation models for human genomics. bioRxiv10.1101/2023.01.11.523679 (2023).
MeSH terms
LinkOut - more resources
Full Text Sources