A Machine Learning Approach to Simulate Gene Expression and Infer Gene Regulatory Networks
- PMID: 37628244
- PMCID: PMC10453511
- DOI: 10.3390/e25081214
A Machine Learning Approach to Simulate Gene Expression and Infer Gene Regulatory Networks
Abstract
The ability to simulate gene expression and infer gene regulatory networks has vast potential applications in various fields, including medicine, agriculture, and environmental science. In recent years, machine learning approaches to simulate gene expression and infer gene regulatory networks have gained significant attention as a promising area of research. By simulating gene expression, we can gain insights into the complex mechanisms that control gene expression and how they are affected by various environmental factors. This knowledge can be used to develop new treatments for genetic diseases, improve crop yields, and better understand the evolution of species. In this article, we address this issue by focusing on a novel method capable of simulating the gene expression regulation of a group of genes and their mutual interactions. Our framework enables us to simulate the regulation of gene expression in response to alterations or perturbations that can affect the expression of a gene. We use both artificial and real benchmarks to empirically evaluate the effectiveness of our methodology. Furthermore, we compare our method with existing ones to understand its advantages and disadvantages. We also present future ideas for improvement to enhance the effectiveness of our method. Overall, our approach has the potential to greatly improve the field of gene expression simulation and gene regulatory network inference, possibly leading to significant advancements in genetics.
Keywords: complex network; gene regulatory network; machine learning; metaheuristic; reverse engineering; time-series forecasting.
Conflict of interest statement
The authors declare no conflict of interest.
Figures
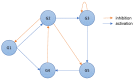
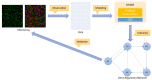
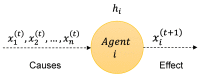
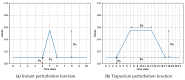
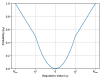
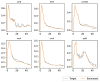
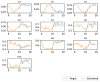
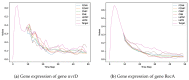
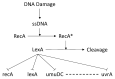
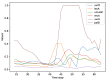
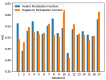
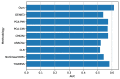
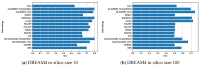
Similar articles
-
MICRAT: a novel algorithm for inferring gene regulatory networks using time series gene expression data.BMC Syst Biol. 2018 Dec 14;12(Suppl 7):115. doi: 10.1186/s12918-018-0635-1. BMC Syst Biol. 2018. PMID: 30547796 Free PMC article.
-
Periodic synchronization of isolated network elements facilitates simulating and inferring gene regulatory networks including stochastic molecular kinetics.BMC Bioinformatics. 2022 Jan 5;23(1):13. doi: 10.1186/s12859-021-04541-6. BMC Bioinformatics. 2022. PMID: 34986805 Free PMC article.
-
Reverse engineering highlights potential principles of large gene regulatory network design and learning.NPJ Syst Biol Appl. 2017 Jun 22;3:17. doi: 10.1038/s41540-017-0019-y. eCollection 2017. NPJ Syst Biol Appl. 2017. PMID: 28649444 Free PMC article.
-
Review and assessment of Boolean approaches for inference of gene regulatory networks.Heliyon. 2022 Aug 9;8(8):e10222. doi: 10.1016/j.heliyon.2022.e10222. eCollection 2022 Aug. Heliyon. 2022. PMID: 36033302 Free PMC article. Review.
-
Learning from Co-expression Networks: Possibilities and Challenges.Front Plant Sci. 2016 Apr 8;7:444. doi: 10.3389/fpls.2016.00444. eCollection 2016. Front Plant Sci. 2016. PMID: 27092161 Free PMC article. Review.
Cited by
-
Hybrid whale algorithm with evolutionary strategies and filtering for high-dimensional optimization: Application to microarray cancer data.PLoS One. 2024 Mar 11;19(3):e0295643. doi: 10.1371/journal.pone.0295643. eCollection 2024. PLoS One. 2024. PMID: 38466740 Free PMC article.
References
Grants and funding
LinkOut - more resources
Full Text Sources