Regulatory networks driving expression of genes critical for glioblastoma are controlled by the transcription factor c-Jun and the pre-existing epigenetic modifications
- PMID: 36850002
- PMCID: PMC9972689
- DOI: 10.1186/s13148-023-01446-4
Regulatory networks driving expression of genes critical for glioblastoma are controlled by the transcription factor c-Jun and the pre-existing epigenetic modifications
Abstract
Background: Glioblastoma (GBM, WHO grade IV) is an aggressive, primary brain tumor. Despite extensive tumor resection followed by radio- and chemotherapy, life expectancy of GBM patients did not improve over decades. Several studies reported transcription deregulation in GBMs, but regulatory mechanisms driving overexpression of GBM-specific genes remain largely unknown. Transcription in open chromatin regions is directed by transcription factors (TFs) that bind to specific motifs, recruit co-activators/repressors and the transcriptional machinery. Identification of GBM-related TFs-gene regulatory networks may reveal new and targetable mechanisms of gliomagenesis.
Results: We predicted TFs-regulated networks in GBMs in silico and intersected them with putative TF binding sites identified in the accessible chromatin in human glioma cells and GBM patient samples. The Cancer Genome Atlas and Glioma Atlas datasets (DNA methylation, H3K27 acetylation, transcriptomic profiles) were explored to elucidate TFs-gene regulatory networks and effects of the epigenetic background. In contrast to the majority of tumors, c-Jun expression was higher in GBMs than in normal brain and c-Jun binding sites were found in multiple genes overexpressed in GBMs, including VIM, FOSL2 or UPP1. Binding of c-Jun to the VIM gene promoter was stronger in GBM-derived cells than in cells derived from benign glioma as evidenced by gel shift and supershift assays. Regulatory regions of the majority of c-Jun targets have distinct DNA methylation patterns in GBMs as compared to benign gliomas, suggesting the contribution of DNA methylation to the c-Jun-dependent gene expression.
Conclusions: GBM-specific TFs-gene networks identified in GBMs differ from regulatory pathways attributed to benign brain tumors and imply a decisive role of c-Jun in controlling genes that drive glioma growth and invasion as well as a modulatory role of DNA methylation.
Keywords: Chromatin accessibility; DNA binding; DNA methylation; Gene expression; Glioblastoma; Transcription factors; Transcriptional deregulation.
© 2023. The Author(s).
Conflict of interest statement
The authors declare that they have no competing interests.
Figures
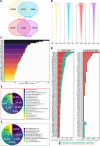
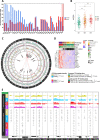
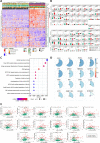
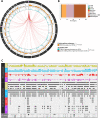
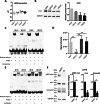
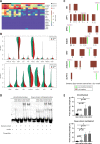
Similar articles
-
Genome-wide methylomic and transcriptomic analyses identify subtype-specific epigenetic signatures commonly dysregulated in glioma stem cells and glioblastoma.Epigenetics. 2018;13(4):432-448. doi: 10.1080/15592294.2018.1469892. Epub 2018 Aug 6. Epigenetics. 2018. PMID: 29927689 Free PMC article.
-
Overexpression of alpha4 chain-containing laminins in human glial tumors identified by gene microarray analysis.Cancer Res. 2001 Jul 15;61(14):5601-10. Cancer Res. 2001. PMID: 11454714
-
Mapping chromatin accessibility and active regulatory elements reveals pathological mechanisms in human gliomas.Nat Commun. 2021 Jun 15;12(1):3621. doi: 10.1038/s41467-021-23922-2. Nat Commun. 2021. PMID: 34131149 Free PMC article.
-
The Impact of Epigenetic Modifications on Adaptive Resistance Evolution in Glioblastoma.Int J Mol Sci. 2021 Aug 3;22(15):8324. doi: 10.3390/ijms22158324. Int J Mol Sci. 2021. PMID: 34361090 Free PMC article. Review.
-
Epigenetics of glioblastoma multiforme: From molecular mechanisms to therapeutic approaches.Semin Cancer Biol. 2022 Aug;83:100-120. doi: 10.1016/j.semcancer.2020.12.015. Epub 2020 Dec 25. Semin Cancer Biol. 2022. PMID: 33370605 Review.
Cited by
-
Eslicarbazepine induces apoptosis and cell cycle arrest in C6 glioma cells in vitro and suppresses tumor growth in an intracranial rat model.BMC Cancer. 2024 Sep 4;24(1):1099. doi: 10.1186/s12885-024-12840-3. BMC Cancer. 2024. PMID: 39232721 Free PMC article.
-
FOSL2-mediated transcription of ISG20 induces M2 polarization of macrophages and enhances tumorigenic ability of glioblastoma cells.J Neurooncol. 2024 Sep;169(3):659-670. doi: 10.1007/s11060-024-04771-7. Epub 2024 Jul 29. J Neurooncol. 2024. PMID: 39073688
-
Navigating the Gene Co-Expression Network and Drug Repurposing Opportunities for Brain Disorders Associated with Neurocognitive Impairment.Brain Sci. 2023 Nov 7;13(11):1564. doi: 10.3390/brainsci13111564. Brain Sci. 2023. PMID: 38002524 Free PMC article.
References
Publication types
MeSH terms
Substances
LinkOut - more resources
Full Text Sources
Medical
Miscellaneous