Deep Visual Proteomics defines single-cell identity and heterogeneity
- PMID: 35590073
- PMCID: PMC9371970
- DOI: 10.1038/s41587-022-01302-5
Deep Visual Proteomics defines single-cell identity and heterogeneity
Abstract
Despite the availabilty of imaging-based and mass-spectrometry-based methods for spatial proteomics, a key challenge remains connecting images with single-cell-resolution protein abundance measurements. Here, we introduce Deep Visual Proteomics (DVP), which combines artificial-intelligence-driven image analysis of cellular phenotypes with automated single-cell or single-nucleus laser microdissection and ultra-high-sensitivity mass spectrometry. DVP links protein abundance to complex cellular or subcellular phenotypes while preserving spatial context. By individually excising nuclei from cell culture, we classified distinct cell states with proteomic profiles defined by known and uncharacterized proteins. In an archived primary melanoma tissue, DVP identified spatially resolved proteome changes as normal melanocytes transition to fully invasive melanoma, revealing pathways that change in a spatial manner as cancer progresses, such as mRNA splicing dysregulation in metastatic vertical growth that coincides with reduced interferon signaling and antigen presentation. The ability of DVP to retain precise spatial proteomic information in the tissue context has implications for the molecular profiling of clinical samples.
© 2022. The Author(s).
Conflict of interest statement
P.H. is the founder and a shareholder of Single-Cell Technologies Ltd., a biodata analysis company that owns and develops the BIAS software. The remaining authors declare no competing interests.
Figures
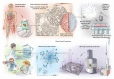
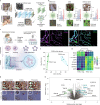
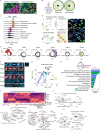
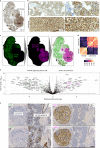
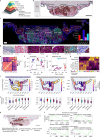
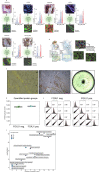
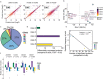
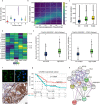
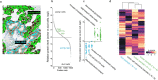
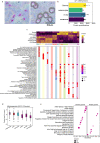
Comment in
-
Spatial proteomics with subcellular resolution.Nat Methods. 2022 Jul;19(7):780. doi: 10.1038/s41592-022-01554-8. Nat Methods. 2022. PMID: 35804240 No abstract available.
Similar articles
-
Spatial single-cell mass spectrometry defines zonation of the hepatocyte proteome.Nat Methods. 2023 Oct;20(10):1530-1536. doi: 10.1038/s41592-023-02007-6. Epub 2023 Oct 2. Nat Methods. 2023. PMID: 37783884 Free PMC article.
-
Utilization of Laser Capture Microdissection Coupled to Mass Spectrometry to Uncover the Proteome of Cellular Protrusions.Methods Mol Biol. 2021;2259:25-45. doi: 10.1007/978-1-0716-1178-4_3. Methods Mol Biol. 2021. PMID: 33687707 Free PMC article.
-
Development of a Sensitive, Scalable Method for Spatial, Cell-Type-Resolved Proteomics of the Human Brain.J Proteome Res. 2019 Apr 5;18(4):1787-1795. doi: 10.1021/acs.jproteome.8b00981. Epub 2019 Feb 25. J Proteome Res. 2019. PMID: 30768908 Free PMC article.
-
Unbiased spatial proteomics with single-cell resolution in tissues.Mol Cell. 2022 Jun 16;82(12):2335-2349. doi: 10.1016/j.molcel.2022.05.022. Mol Cell. 2022. PMID: 35714588 Review.
-
Chemical tools for temporally and spatially resolved mass spectrometry-based proteomics.Ann Biomed Eng. 2014 Feb;42(2):299-311. doi: 10.1007/s10439-013-0878-3. Epub 2013 Aug 14. Ann Biomed Eng. 2014. PMID: 23943069 Free PMC article. Review.
Cited by
-
Imputation of label-free quantitative mass spectrometry-based proteomics data using self-supervised deep learning.Nat Commun. 2024 Jun 26;15(1):5405. doi: 10.1038/s41467-024-48711-5. Nat Commun. 2024. PMID: 38926340 Free PMC article.
-
Recent advances in the field of single-cell proteomics.Transl Oncol. 2023 Jan;27:101556. doi: 10.1016/j.tranon.2022.101556. Epub 2022 Oct 19. Transl Oncol. 2023. PMID: 36270102 Free PMC article.
-
Spatial Proteomics for the Molecular Characterization of Breast Cancer.Proteomes. 2023 May 3;11(2):17. doi: 10.3390/proteomes11020017. Proteomes. 2023. PMID: 37218922 Free PMC article. Review.
-
Insights into protein post-translational modification landscapes of individual human cells by trapped ion mobility time-of-flight mass spectrometry.Nat Commun. 2022 Nov 25;13(1):7246. doi: 10.1038/s41467-022-34919-w. Nat Commun. 2022. PMID: 36433961 Free PMC article.
-
Time-of-Flight Fragmentation Spectra Generated by the Proteomic Analysis of Single Human Cells Do Not Exhibit Atypical Fragmentation Patterns.J Proteome Res. 2023 Mar 3;22(3):1003-1008. doi: 10.1021/acs.jproteome.2c00715. Epub 2023 Jan 26. J Proteome Res. 2023. PMID: 36700448 Free PMC article.
References
-
- Hériché J-K, Alexander S, Ellenberg J. Integrating imaging and omics: computational methods and challenges. Annu. Rev. Biomed. Data Sci. 2019;2:175–197. doi: 10.1146/annurev-biodatasci-080917-013328. - DOI
-
- Isola, P., Zhu, J.-Y., Zhou, T. & Efros, A. A. Image-to-image translation with conditional adversarial networks. Preprint at https://arxiv.org/abs/1611.07004 (2016).
Publication types
MeSH terms
Substances
Grants and funding
LinkOut - more resources
Full Text Sources
Other Literature Sources
Medical
Research Materials