Approach for the Design of Covalent Protein Kinase Inhibitors via Focused Deep Generative Modeling
- PMID: 35056884
- PMCID: PMC8778003
- DOI: 10.3390/molecules27020570
Approach for the Design of Covalent Protein Kinase Inhibitors via Focused Deep Generative Modeling
Abstract
Deep machine learning is expanding the conceptual framework and capacity of computational compound design, enabling new applications through generative modeling. We have explored the systematic design of covalent protein kinase inhibitors by learning from kinome-relevant chemical space, followed by focusing on an exemplary kinase of interest. Covalent inhibitors experience a renaissance in drug discovery, especially for targeting protein kinases. However, computational design of this class of inhibitors has thus far only been little investigated. To this end, we have devised a computational approach combining fragment-based design and deep generative modeling augmented by three-dimensional pharmacophore screening. This approach is thought to be particularly relevant for medicinal chemistry applications because it combines knowledge-based elements with deep learning and is chemically intuitive. As an exemplary application, we report for Bruton's tyrosine kinase (BTK), a major drug target for the treatment of inflammatory diseases and leukemia, the generation of novel candidate inhibitors with a specific chemically reactive group for covalent modification, requiring only little target-specific compound information to guide the design efforts. Newly generated compounds include known inhibitors and characteristic substructures and many novel candidates, thus lending credence to the computational approach, which is readily applicable to other targets.
Keywords: Bruton’s tyrosine kinase; covalent inhibitors; deep machine learning; generative modeling; kinase inhibitor design.
Conflict of interest statement
The authors declare no conflict of interest.
Figures
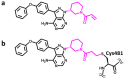
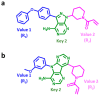
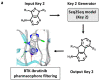
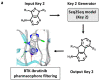
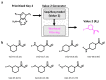
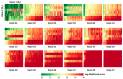
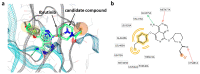
Similar articles
-
Pharmacophore modeling and virtual screening in search of novel Bruton's tyrosine kinase inhibitors.J Mol Model. 2019 Jun 6;25(7):179. doi: 10.1007/s00894-019-4047-y. J Mol Model. 2019. PMID: 31172362
-
Discovery of potent, highly selective covalent irreversible BTK inhibitors from a fragment hit.Bioorg Med Chem Lett. 2018 Sep 15;28(17):2939-2944. doi: 10.1016/j.bmcl.2018.07.008. Epub 2018 Jul 5. Bioorg Med Chem Lett. 2018. PMID: 30122225
-
Discovery of a Novel Class of Covalent Dual Inhibitors Targeting the Protein Kinases BMX and BTK.Int J Mol Sci. 2020 Dec 4;21(23):9269. doi: 10.3390/ijms21239269. Int J Mol Sci. 2020. PMID: 33291717 Free PMC article.
-
Emerging small-molecule inhibitors of the Bruton's tyrosine kinase (BTK): Current development.Eur J Med Chem. 2021 May 5;217:113329. doi: 10.1016/j.ejmech.2021.113329. Epub 2021 Mar 12. Eur J Med Chem. 2021. PMID: 33740548 Review.
-
The development of Bruton's tyrosine kinase (BTK) inhibitors from 2012 to 2017: A mini-review.Eur J Med Chem. 2018 May 10;151:315-326. doi: 10.1016/j.ejmech.2018.03.062. Epub 2018 Mar 23. Eur J Med Chem. 2018. PMID: 29631132 Review.
Cited by
-
Reactivity of Covalent Fragments and Their Role in Fragment Based Drug Discovery.Pharmaceuticals (Basel). 2022 Nov 8;15(11):1366. doi: 10.3390/ph15111366. Pharmaceuticals (Basel). 2022. PMID: 36355538 Free PMC article. Review.
-
Interpretable Machine Learning Models for Molecular Design of Tyrosine Kinase Inhibitors Using Variational Autoencoders and Perturbation-Based Approach of Chemical Space Exploration.Int J Mol Sci. 2022 Sep 24;23(19):11262. doi: 10.3390/ijms231911262. Int J Mol Sci. 2022. PMID: 36232566 Free PMC article.
-
Designing Next-Generation Drug-like Molecules for Medicinal Applications.Molecules. 2023 Feb 16;28(4):1860. doi: 10.3390/molecules28041860. Molecules. 2023. PMID: 36838848 Free PMC article.
-
Chemical Multiverse: An Expanded View of Chemical Space.Mol Inform. 2022 Nov;41(11):e2200116. doi: 10.1002/minf.202200116. Epub 2022 Aug 23. Mol Inform. 2022. PMID: 35916110 Free PMC article. Review.
-
Streamlining Computational Fragment-Based Drug Discovery through Evolutionary Optimization Informed by Ligand-Based Virtual Prescreening.J Chem Inf Model. 2024 May 13;64(9):3826-3840. doi: 10.1021/acs.jcim.4c00234. Epub 2024 May 2. J Chem Inf Model. 2024. PMID: 38696451 Free PMC article.
References
MeSH terms
Substances
LinkOut - more resources
Full Text Sources
Research Materials