Can Machine Learning from Real-World Data Support Drug Treatment Decisions? A Prediction Modeling Case for Direct Oral Anticoagulants
- PMID: 34911402
- PMCID: PMC9189725
- DOI: 10.1177/0272989X211064604
Can Machine Learning from Real-World Data Support Drug Treatment Decisions? A Prediction Modeling Case for Direct Oral Anticoagulants
Abstract
Background: Decision making for the "best" treatment is particularly challenging in situations in which individual patient response to drugs can largely differ from average treatment effects. By estimating individual treatment effects (ITEs), we aimed to demonstrate how strokes, major bleeding events, and a composite of both could be reduced by model-assisted recommendations for a particular direct oral anticoagulant (DOAC).
Methods: In German claims data for the calendar years 2014-2018, we selected 29 901 new users of the DOACs rivaroxaban and apixaban. Random forests considered binary events within 1 y to estimate ITEs under each DOAC according to the X-learner algorithm with 29 potential effect modifiers; treatment recommendations were based on these estimated ITEs. Model performance was evaluated by the c-for-benefit statistics, absolute risk reduction (ARR), and absolute risk difference (ARD) by trial emulation.
Results: A significant proportion of patients would be recommended a different treatment option than they actually received. The stroke model significantly discriminated patients for higher benefit and thus indicated improved decisions by reduced outcomes (c-for-benefit: 0.56; 95% confidence interval [0.52; 0.60]). In the group with apixaban recommendation, the model also improved the composite endpoint (ARR: 1.69 % [0.39; 2.97]). In trial emulations, model-assisted recommendations significantly reduced the composite event rate (ARD: -0.78 % [-1.40; -0.03]).
Conclusions: If prescribers are undecided about the potential benefits of different treatment options, ITEs can support decision making, especially if evidence is inconclusive, risk-benefit profiles of therapeutic alternatives differ significantly, and the patients' complexity deviates from "typical" study populations. In the exemplary case for DOACs and potentially in other situations, the significant impact could also become practically relevant if recommendations were available in an automated way as part of decision making.HighlightsIt was possible to calculate individual treatment effects (ITEs) from routine claims data for rivaroxaban and apixaban, and the characteristics between the groups with recommendation for one or the other option differed significantly.ITEs resulted in recommendations that were significantly superior to usual (observed) treatment allocations in terms of absolute risk reduction, both separately for stroke and in the composite endpoint of stroke and major bleeding.When similar patients from routine data were selected (precision cohorts) for patients with a strong recommendation for one option or the other, those similar patients under the respective recommendation showed a significantly better prognosis compared with the alternative option.Many steps may still be needed on the way to clinical practice, but the principle of decision support developed from routine data may point the way toward future decision-making processes.
Keywords: claims data; clinical decision support system; direct oral anticoagulants; heterogeneous treatment effects; machine-learning; personalized medicine.
Conflict of interest statement
The authors declared no potential conflicts of interest with respect to the research, authorship, and/or publication of this article.
The authors disclosed receipt of the following financial support for the research, authorship, and/or publication of this article: Financial support for this study was provided in part by a contract with the statutory health insurance fund
Figures
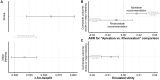
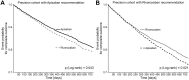
Similar articles
-
Dose specific effectiveness and safety of DOACs in patients with non-valvular atrial fibrillation: A Canadian retrospective cohort study.Thromb Res. 2021 Jul;203:121-130. doi: 10.1016/j.thromres.2021.05.005. Epub 2021 May 11. Thromb Res. 2021. PMID: 34000493
-
Comparison of the Safety and Effectiveness of Four Direct Oral Anticoagulants in Japanese Patients with Nonvalvular Atrial Fibrillation Using Real-World Data.Biol Pharm Bull. 2021;44(9):1294-1302. doi: 10.1248/bpb.b21-00230. Biol Pharm Bull. 2021. PMID: 34471057
-
An early evaluation of bleeding-related hospital readmissions among hospitalized patients with nonvalvular atrial fibrillation treated with direct oral anticoagulants.Curr Med Res Opin. 2016;32(3):573-82. doi: 10.1185/03007995.2015.1131676. Epub 2016 Jan 1. Curr Med Res Opin. 2016. PMID: 26652179
-
Recommendations for the emergency management of complications associated with the new direct oral anticoagulants (DOACs), apixaban, dabigatran and rivaroxaban.Clin Res Cardiol. 2013 Jun;102(6):399-412. doi: 10.1007/s00392-013-0560-7. Epub 2013 May 14. Clin Res Cardiol. 2013. PMID: 23669868 Review.
-
Systematic review and adjusted indirect comparison meta-analysis of oral anticoagulants in atrial fibrillation.Circ Cardiovasc Qual Outcomes. 2012 Sep 1;5(5):711-9. doi: 10.1161/CIRCOUTCOMES.112.966572. Epub 2012 Aug 21. Circ Cardiovasc Qual Outcomes. 2012. PMID: 22912382 Review.
Cited by
-
Reporting of Observational Studies Explicitly Aiming to Emulate Randomized Trials: A Systematic Review.JAMA Netw Open. 2023 Sep 5;6(9):e2336023. doi: 10.1001/jamanetworkopen.2023.36023. JAMA Netw Open. 2023. PMID: 37755828 Free PMC article.
-
Methodological concerns about "concordance-statistic for benefit" as a measure of discrimination in predicting treatment benefit.Diagn Progn Res. 2023 May 16;7(1):10. doi: 10.1186/s41512-023-00147-z. Diagn Progn Res. 2023. PMID: 37189162 Free PMC article.
-
The role of INTERCheckWEB digital innovation in supporting polytherapy management.Sci Rep. 2023 Apr 4;13(1):5544. doi: 10.1038/s41598-023-32844-6. Sci Rep. 2023. PMID: 37016155 Free PMC article.
-
Potential Applications of Artificial Intelligence (AI) in Managing Polypharmacy in Saudi Arabia: A Narrative Review.Healthcare (Basel). 2024 Apr 5;12(7):788. doi: 10.3390/healthcare12070788. Healthcare (Basel). 2024. PMID: 38610210 Free PMC article. Review.
References
-
- Armstrong KA, Metlay JP. Annals clinical decision making: communicating risk and engaging patients in shared decision making. Ann Intern Med. 2020;172(10):688–92. - PubMed
-
- Kent DM, Hayward RA. Limitations of applying summary results of clinical trials to individual patients: the need for risk stratification. JAMA. 2007;298(10):1209–12. - PubMed
-
- Armstrong KA, Metlay JP. Annals clinical decision making: translating population evidence to individual patients. Ann Intern Med. 2020;172(9):610–6. - PubMed
Publication types
MeSH terms
Substances
LinkOut - more resources
Full Text Sources
Medical