De novo protein design by deep network hallucination
- PMID: 34853475
- PMCID: PMC9293396
- DOI: 10.1038/s41586-021-04184-w
De novo protein design by deep network hallucination
Abstract
There has been considerable recent progress in protein structure prediction using deep neural networks to predict inter-residue distances from amino acid sequences1-3. Here we investigate whether the information captured by such networks is sufficiently rich to generate new folded proteins with sequences unrelated to those of the naturally occurring proteins used in training the models. We generate random amino acid sequences, and input them into the trRosetta structure prediction network to predict starting residue-residue distance maps, which, as expected, are quite featureless. We then carry out Monte Carlo sampling in amino acid sequence space, optimizing the contrast (Kullback-Leibler divergence) between the inter-residue distance distributions predicted by the network and background distributions averaged over all proteins. Optimization from different random starting points resulted in novel proteins spanning a wide range of sequences and predicted structures. We obtained synthetic genes encoding 129 of the network-'hallucinated' sequences, and expressed and purified the proteins in Escherichia coli; 27 of the proteins yielded monodisperse species with circular dichroism spectra consistent with the hallucinated structures. We determined the three-dimensional structures of three of the hallucinated proteins, two by X-ray crystallography and one by NMR, and these closely matched the hallucinated models. Thus, deep networks trained to predict native protein structures from their sequences can be inverted to design new proteins, and such networks and methods should contribute alongside traditional physics-based models to the de novo design of proteins with new functions.
© 2021. The Author(s), under exclusive licence to Springer Nature Limited.
Conflict of interest statement
Competing interests
G.T.M is a co-founder of Nexomics Biosciences, Inc.
Figures
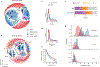
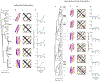
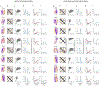
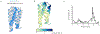
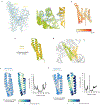
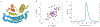
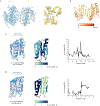
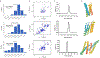
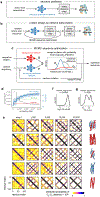
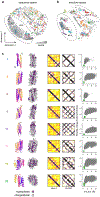
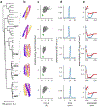
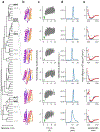
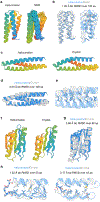
Comment in
-
Dreaming ideal protein structures.Nat Biotechnol. 2022 Feb;40(2):171-172. doi: 10.1038/s41587-021-01196-9. Nat Biotechnol. 2022. PMID: 35075248 No abstract available.
-
Scientists are using AI to dream up revolutionary new proteins.Nature. 2022 Sep;609(7928):661-662. doi: 10.1038/d41586-022-02947-7. Nature. 2022. PMID: 36109683 No abstract available.
Similar articles
-
CNNcon: improved protein contact maps prediction using cascaded neural networks.PLoS One. 2013 Apr 23;8(4):e61533. doi: 10.1371/journal.pone.0061533. Print 2013. PLoS One. 2013. PMID: 23626696 Free PMC article.
-
The trRosetta server for fast and accurate protein structure prediction.Nat Protoc. 2021 Dec;16(12):5634-5651. doi: 10.1038/s41596-021-00628-9. Epub 2021 Nov 10. Nat Protoc. 2021. PMID: 34759384 Review.
-
Protein sequence design by conformational landscape optimization.Proc Natl Acad Sci U S A. 2021 Mar 16;118(11):e2017228118. doi: 10.1073/pnas.2017228118. Proc Natl Acad Sci U S A. 2021. PMID: 33712545 Free PMC article.
-
SeqPredNN: a neural network that generates protein sequences that fold into specified tertiary structures.BMC Bioinformatics. 2023 Oct 3;24(1):373. doi: 10.1186/s12859-023-05498-4. BMC Bioinformatics. 2023. PMID: 37789284 Free PMC article.
-
De novo protein design-From new structures to programmable functions.Cell. 2024 Feb 1;187(3):526-544. doi: 10.1016/j.cell.2023.12.028. Cell. 2024. PMID: 38306980 Review.
Cited by
-
Exploring and Learning the Universe of Protein Allostery Using Artificial Intelligence Augmented Biophysical and Computational Approaches.J Chem Inf Model. 2023 Mar 13;63(5):1413-1428. doi: 10.1021/acs.jcim.2c01634. Epub 2023 Feb 24. J Chem Inf Model. 2023. PMID: 36827465 Free PMC article. Review.
-
AlphaFold2 models indicate that protein sequence determines both structure and dynamics.Sci Rep. 2022 Jun 23;12(1):10696. doi: 10.1038/s41598-022-14382-9. Sci Rep. 2022. PMID: 35739160 Free PMC article.
-
Ig-VAE: Generative modeling of protein structure by direct 3D coordinate generation.PLoS Comput Biol. 2022 Jun 27;18(6):e1010271. doi: 10.1371/journal.pcbi.1010271. eCollection 2022 Jun. PLoS Comput Biol. 2022. PMID: 35759518 Free PMC article.
-
Protein Design with Deep Learning.Int J Mol Sci. 2021 Oct 29;22(21):11741. doi: 10.3390/ijms222111741. Int J Mol Sci. 2021. PMID: 34769173 Free PMC article. Review.
-
RNA contact prediction by data efficient deep learning.Commun Biol. 2023 Sep 6;6(1):913. doi: 10.1038/s42003-023-05244-9. Commun Biol. 2023. PMID: 37674020 Free PMC article.
References
-
- Senior AW et al. Improved protein structure prediction using potentials from deep learning. Nature 577, 706–710 (2020). - PubMed
-
- Biswas S, Khimulya G, Alley EC, Esvelt KM & Church GM Low-N protein engineering with data-efficient deep learning. Nat. Methods 18, 389–396 (2021). - PubMed
-
- Madani A et al. ProGen: Language Modeling for Protein Generation. bioRxiv (2020) doi:10.1101/2020.03.07.982272. - DOI
Publication types
MeSH terms
Substances
Grants and funding
LinkOut - more resources
Full Text Sources