Accurate prediction of protein structures and interactions using a three-track neural network
- PMID: 34282049
- PMCID: PMC7612213
- DOI: 10.1126/science.abj8754
Accurate prediction of protein structures and interactions using a three-track neural network
Abstract
DeepMind presented notably accurate predictions at the recent 14th Critical Assessment of Structure Prediction (CASP14) conference. We explored network architectures that incorporate related ideas and obtained the best performance with a three-track network in which information at the one-dimensional (1D) sequence level, the 2D distance map level, and the 3D coordinate level is successively transformed and integrated. The three-track network produces structure predictions with accuracies approaching those of DeepMind in CASP14, enables the rapid solution of challenging x-ray crystallography and cryo-electron microscopy structure modeling problems, and provides insights into the functions of proteins of currently unknown structure. The network also enables rapid generation of accurate protein-protein complex models from sequence information alone, short-circuiting traditional approaches that require modeling of individual subunits followed by docking. We make the method available to the scientific community to speed biological research.
Copyright © 2021 The Authors, some rights reserved; exclusive licensee American Association for the Advancement of Science. No claim to original U.S. Government Works.
Conflict of interest statement
Figures
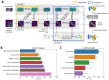
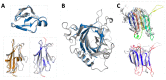
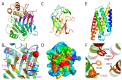
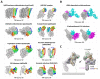
Comment in
-
Solution of the protein structure prediction problem at last: crucial innovations and next frontiers.Fac Rev. 2022 Dec 14;11:38. doi: 10.12703/r-01-0000020. eCollection 2022. Fac Rev. 2022. PMID: 36644294 Free PMC article.
Similar articles
-
Applying and improving AlphaFold at CASP14.Proteins. 2021 Dec;89(12):1711-1721. doi: 10.1002/prot.26257. Proteins. 2021. PMID: 34599769 Free PMC article.
-
Deep Learning-Based Advances in Protein Structure Prediction.Int J Mol Sci. 2021 May 24;22(11):5553. doi: 10.3390/ijms22115553. Int J Mol Sci. 2021. PMID: 34074028 Free PMC article. Review.
-
Evaluation of Deep Neural Network ProSPr for Accurate Protein Distance Predictions on CASP14 Targets.Int J Mol Sci. 2021 Nov 27;22(23):12835. doi: 10.3390/ijms222312835. Int J Mol Sci. 2021. PMID: 34884640 Free PMC article.
-
Hybridized distance- and contact-based hierarchical structure modeling for folding soluble and membrane proteins.PLoS Comput Biol. 2021 Feb 23;17(2):e1008753. doi: 10.1371/journal.pcbi.1008753. eCollection 2021 Feb. PLoS Comput Biol. 2021. PMID: 33621244 Free PMC article.
-
Assessment of Protein-Protein Docking Models Using Deep Learning.Methods Mol Biol. 2024;2780:149-162. doi: 10.1007/978-1-0716-3985-6_10. Methods Mol Biol. 2024. PMID: 38987469 Review.
Cited by
-
Fit-for-purpose heterodivalent single-domain antibody for gastrointestinal targeting of toxin B from Clostridium difficile.Protein Sci. 2024 Jul;33(7):e5035. doi: 10.1002/pro.5035. Protein Sci. 2024. PMID: 38923049 Free PMC article.
-
Structural and Evolutionary Analysis of Proteins Endowed with a Nucleotidyltransferase, or Non-canonical Palm, Catalytic Domain.J Mol Evol. 2024 Dec;92(6):799-814. doi: 10.1007/s00239-024-10207-7. Epub 2024 Sep 19. J Mol Evol. 2024. PMID: 39297932 Free PMC article.
-
The Effects of Codon Usage on Protein Structure and Folding.Annu Rev Biophys. 2024 Jul;53(1):87-108. doi: 10.1146/annurev-biophys-030722-020555. Epub 2024 Jun 28. Annu Rev Biophys. 2024. PMID: 38134335 Review.
-
Molecular dynamics simulations reveal differences in the conformational stability of FtsZs derived from Staphylococcus aureus and Bacillus subtilis.Sci Rep. 2024 Jul 11;14(1):16043. doi: 10.1038/s41598-024-66763-x. Sci Rep. 2024. PMID: 38992051 Free PMC article.
-
Assessing and enhancing foldability in designed proteins.Protein Sci. 2022 Sep;31(9):e4400. doi: 10.1002/pro.4400. Protein Sci. 2022. PMID: 36040259 Free PMC article.
References
-
- Senior AW, Evans R, Jumper J, Kirkpatrick J, Sifre L, Green T, Qin C, Žídek A, Nelson AWR, Bridgland A, Penedones H, et al. Improved protein structure prediction using potentials from deep learning. Nature. 2020;577:706–710. - PubMed
-
- Jumper John, Evans Richard, Pritzel Alexander, Green Tim, Figurnov Michael, Tunyasuvunakool Kathryn, Ronneberger Olaf, Bates Russ, Augustin Žídek Alex, Bridgland Clemens, Meyer, et al. Fourteenth Critical Assessment of Techniques for Protein Structure Prediction
Publication types
MeSH terms
Substances
Grants and funding
LinkOut - more resources
Full Text Sources
Other Literature Sources