Framework for identifying drug repurposing candidates from observational healthcare data
- PMID: 33623890
- PMCID: PMC7886555
- DOI: 10.1093/jamiaopen/ooaa048
Framework for identifying drug repurposing candidates from observational healthcare data
Abstract
Objective: Observational medical databases, such as electronic health records and insurance claims, track the healthcare trajectory of millions of individuals. These databases provide real-world longitudinal information on large cohorts of patients and their medication prescription history. We present an easy-to-customize framework that systematically analyzes such databases to identify new indications for on-market prescription drugs.
Materials and methods: Our framework provides an interface for defining study design parameters and extracting patient cohorts, disease-related outcomes, and potential confounders in observational databases. It then applies causal inference methodology to emulate hundreds of randomized controlled trials (RCTs) for prescribed drugs, while adjusting for confounding and selection biases. After correcting for multiple testing, it outputs the estimated effects and their statistical significance in each database.
Results: We demonstrate the utility of the framework in a case study of Parkinson's disease (PD) and evaluate the effect of 259 drugs on various PD progression measures in two observational medical databases, covering more than 150 million patients. The results of these emulated trials reveal remarkable agreement between the two databases for the most promising candidates.
Discussion: Estimating drug effects from observational data is challenging due to data biases and noise. To tackle this challenge, we integrate causal inference methodology with domain knowledge and compare the estimated effects in two separate databases.
Conclusion: Our framework enables systematic search for drug repurposing candidates by emulating RCTs using observational data. The high level of agreement between separate databases strongly supports the identified effects.
Keywords: Parkinson’s disease; causal inference; comparative effectiveness research; drug repositioning; electronic health records.
© The Author(s) 2020. Published by Oxford University Press on behalf of the American Medical Informatics Association.
Figures
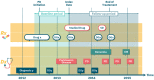
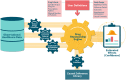
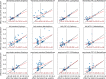
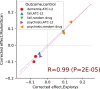
Similar articles
-
Folic acid supplementation and malaria susceptibility and severity among people taking antifolate antimalarial drugs in endemic areas.Cochrane Database Syst Rev. 2022 Feb 1;2(2022):CD014217. doi: 10.1002/14651858.CD014217. Cochrane Database Syst Rev. 2022. PMID: 36321557 Free PMC article.
-
Emulated Clinical Trials from Longitudinal Real-World Data Efficiently Identify Candidates for Neurological Disease Modification: Examples from Parkinson's Disease.Front Pharmacol. 2021 Apr 22;12:631584. doi: 10.3389/fphar.2021.631584. eCollection 2021. Front Pharmacol. 2021. PMID: 33967767 Free PMC article.
-
A deep learning framework for drug repurposing via emulating clinical trials on real-world patient data.Nat Mach Intell. 2021 Jan;3(1):68-75. doi: 10.1038/s42256-020-00276-w. Epub 2021 Jan 4. Nat Mach Intell. 2021. PMID: 35603127 Free PMC article.
-
Healthcare outcomes assessed with observational study designs compared with those assessed in randomized trials.Cochrane Database Syst Rev. 2014 Apr 29;2014(4):MR000034. doi: 10.1002/14651858.MR000034.pub2. Cochrane Database Syst Rev. 2014. Update in: Cochrane Database Syst Rev. 2024 Jan 4;1:MR000034. doi: 10.1002/14651858.MR000034.pub3 PMID: 24782322 Free PMC article. Updated. Review.
-
Implementation of the trial emulation approach in medical research: a scoping review.BMC Med Res Methodol. 2023 Aug 16;23(1):186. doi: 10.1186/s12874-023-02000-9. BMC Med Res Methodol. 2023. PMID: 37587484 Free PMC article. Review.
Cited by
-
Reporting of Observational Studies Explicitly Aiming to Emulate Randomized Trials: A Systematic Review.JAMA Netw Open. 2023 Sep 5;6(9):e2336023. doi: 10.1001/jamanetworkopen.2023.36023. JAMA Netw Open. 2023. PMID: 37755828 Free PMC article.
-
Computational drug repurposing based on electronic health records: a scoping review.NPJ Digit Med. 2022 Jun 14;5(1):77. doi: 10.1038/s41746-022-00617-6. NPJ Digit Med. 2022. PMID: 35701544 Free PMC article. Review.
-
Artificial intelligence for COVID-19: battling the pandemic with computational intelligence.Intell Med. 2022 Feb;2(1):13-29. doi: 10.1016/j.imed.2021.09.001. Epub 2021 Oct 21. Intell Med. 2022. PMID: 34697578 Free PMC article. Review.
-
High-throughput target trial emulation for Alzheimer's disease drug repurposing with real-world data.Nat Commun. 2023 Dec 11;14(1):8180. doi: 10.1038/s41467-023-43929-1. Nat Commun. 2023. PMID: 38081829 Free PMC article.
-
A network-based systems genetics framework identifies pathobiology and drug repurposing in Parkinson's disease.Res Sq [Preprint]. 2024 Oct 14:rs.3.rs-4869009. doi: 10.21203/rs.3.rs-4869009/v1. Res Sq. 2024. PMID: 39483867 Free PMC article. Preprint.
References
-
- Langedijk J, Mantel-Teeuwisse AK, Slijkerman DS, et al.Drug repositioning and repurposing: terminology and definitions in literature. Drug Discov Today 2015; 20 (8): 1027–34. - PubMed
-
- Ashburn TT, Thor KB.. Drug repositioning: identifying and developing new uses for existing drugs. Nat Rev Drug Discov 2004; 3 (8): 673–83. - PubMed
-
- Pushpakom S, Iorio F, Eyers PA, et al.Drug repurposing: progress, challenges and recommendations. Nat Rev Drug Discov October 2018; 18 (1): 41–58. - PubMed
-
- Hurle MR, Yang L, Xie Q, et al.Computational drug repositioning: from data to therapeutics. Clin Pharmacol Ther 2013; 93 (4): 335–41. - PubMed
LinkOut - more resources
Full Text Sources