Generative chemistry: drug discovery with deep learning generative models
- PMID: 33543405
- PMCID: PMC10984615
- DOI: 10.1007/s00894-021-04674-8
Generative chemistry: drug discovery with deep learning generative models
Abstract
The de novo design of molecular structures using deep learning generative models introduces an encouraging solution to drug discovery in the face of the continuously increased cost of new drug development. From the generation of original texts, images, and videos, to the scratching of novel molecular structures the creativity of deep learning generative models exhibits the height machine intelligence can achieve. The purpose of this paper is to review the latest advances in generative chemistry which relies on generative modeling to expedite the drug discovery process. This review starts with a brief history of artificial intelligence in drug discovery to outline this emerging paradigm. Commonly used chemical databases, molecular representations, and tools in cheminformatics and machine learning are covered as the infrastructure for generative chemistry. The detailed discussions on utilizing cutting-edge generative architectures, including recurrent neural network, variational autoencoder, adversarial autoencoder, and generative adversarial network for compound generation are focused. Challenges and future perspectives follow.
Keywords: Adversarial autoencoder; Deep learning; Drug discovery; Generative adversarial network; Generative model; Recurrent neural network; Variational autoencoder.
Conflict of interest statement
Figures
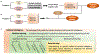
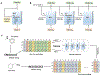
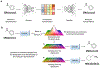
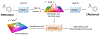
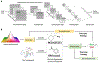
Similar articles
-
druGAN: An Advanced Generative Adversarial Autoencoder Model for de Novo Generation of New Molecules with Desired Molecular Properties in Silico.Mol Pharm. 2017 Sep 5;14(9):3098-3104. doi: 10.1021/acs.molpharmaceut.7b00346. Epub 2017 Aug 4. Mol Pharm. 2017. PMID: 28703000
-
Data Integration Using Advances in Machine Learning in Drug Discovery and Molecular Biology.Methods Mol Biol. 2021;2190:167-184. doi: 10.1007/978-1-0716-0826-5_7. Methods Mol Biol. 2021. PMID: 32804365 Review.
-
Navigating the frontier of drug-like chemical space with cutting-edge generative AI models.Drug Discov Today. 2024 Sep;29(9):104133. doi: 10.1016/j.drudis.2024.104133. Epub 2024 Aug 3. Drug Discov Today. 2024. PMID: 39103144 Review.
-
De Novo Peptide and Protein Design Using Generative Adversarial Networks: An Update.J Chem Inf Model. 2022 Feb 28;62(4):761-774. doi: 10.1021/acs.jcim.1c01361. Epub 2022 Feb 7. J Chem Inf Model. 2022. PMID: 35128926 Review.
-
The power of deep learning to ligand-based novel drug discovery.Expert Opin Drug Discov. 2020 Jul;15(7):755-764. doi: 10.1080/17460441.2020.1745183. Epub 2020 Mar 31. Expert Opin Drug Discov. 2020. PMID: 32228116 Review.
Cited by
-
Machine Learning Guided Discovery of Non-Hemolytic Membrane Disruptive Anticancer Peptides.ChemMedChem. 2022 Sep 5;17(17):e202200291. doi: 10.1002/cmdc.202200291. Epub 2022 Aug 5. ChemMedChem. 2022. PMID: 35880810 Free PMC article.
-
Machine learning approaches for biomolecular, biophysical, and biomaterials research.Biophys Rev (Melville). 2022 Jun 3;3(2):021306. doi: 10.1063/5.0082179. eCollection 2022 Jun. Biophys Rev (Melville). 2022. PMID: 38505413 Free PMC article. Review.
-
Target-driven machine learning-enabled virtual screening (TAME-VS) platform for early-stage hit identification.Front Mol Biosci. 2023 Mar 13;10:1163536. doi: 10.3389/fmolb.2023.1163536. eCollection 2023. Front Mol Biosci. 2023. PMID: 36994428 Free PMC article.
-
Formulation Graphs for Mapping Structure-Composition of Battery Electrolytes to Device Performance.J Chem Inf Model. 2023 Nov 27;63(22):6998-7010. doi: 10.1021/acs.jcim.3c01030. Epub 2023 Nov 10. J Chem Inf Model. 2023. PMID: 37948621 Free PMC article.
-
Deep learning for studying drawing behavior: A review.Front Psychol. 2023 Feb 8;14:992541. doi: 10.3389/fpsyg.2023.992541. eCollection 2023. Front Psychol. 2023. PMID: 36844320 Free PMC article. Review.
References
-
- DiMasi JA, Grabowski HG, Hansen RW (2016) Innovation in the pharmaceutical industry: new estimates of R&D costs. J Health Econ 47:20–33 - PubMed
-
- Blay V, Tolani B, Ho SP, Arkin MR (2020) High-Throughput Screening: today’s biochemical and cell-based approaches. Drug Discov Today 25:1807–1821 - PubMed
-
- Kroemer RT (2007) Structure-based drug design: docking and scoring. Curr Protein Pept Sci 8:312–328 - PubMed
Publication types
MeSH terms
Grants and funding
LinkOut - more resources
Full Text Sources
Other Literature Sources