Compromised Humoral Functional Evolution Tracks with SARS-CoV-2 Mortality
- PMID: 33207184
- PMCID: PMC7608014
- DOI: 10.1016/j.cell.2020.10.052
Compromised Humoral Functional Evolution Tracks with SARS-CoV-2 Mortality
Abstract
The urgent need for an effective SARS-CoV-2 vaccine has forced development to progress in the absence of well-defined correlates of immunity. While neutralization has been linked to protection against other pathogens, whether neutralization alone will be sufficient to drive protection against SARS-CoV-2 in the broader population remains unclear. Therefore, to fully define protective humoral immunity, we dissected the early evolution of the humoral response in 193 hospitalized individuals ranging from moderate to severe. Although robust IgM and IgA responses evolved in both survivors and non-survivors with severe disease, non-survivors showed attenuated IgG responses, accompanied by compromised Fcɣ receptor binding and Fc effector activity, pointing to deficient humoral development rather than disease-enhancing humoral immunity. In contrast, individuals with moderate disease exhibited delayed responses that ultimately matured. These data highlight distinct humoral trajectories associated with resolution of SARS-CoV-2 infection and the need for early functional humoral immunity.
Keywords: COVID-19; Fc receptors; SARS-CoV-2; antibodies; dynamics; innate immunity.
Copyright © 2020 Elsevier Inc. All rights reserved.
Conflict of interest statement
Declaration of Interests G.A. is a founder of SeromYx Systems, Inc. The Systems Serology platform is pending as a patent to G.A. No other authors have interests to declare.
Figures
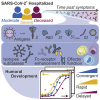
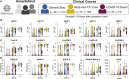
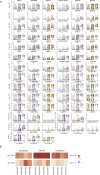
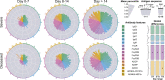
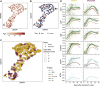
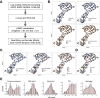
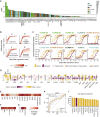
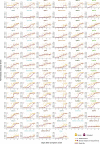
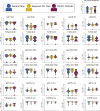
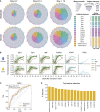
Similar articles
-
Comparative analyses of SARS-CoV-2 binding (IgG, IgM, IgA) and neutralizing antibodies from human serum samples.J Immunol Methods. 2021 Feb;489:112937. doi: 10.1016/j.jim.2020.112937. Epub 2020 Nov 28. J Immunol Methods. 2021. PMID: 33253698 Free PMC article.
-
Evolution of antibody immunity to SARS-CoV-2.Nature. 2021 Mar;591(7851):639-644. doi: 10.1038/s41586-021-03207-w. Epub 2021 Jan 18. Nature. 2021. PMID: 33461210 Free PMC article.
-
Systems serology detects functionally distinct coronavirus antibody features in children and elderly.Nat Commun. 2021 Apr 1;12(1):2037. doi: 10.1038/s41467-021-22236-7. Nat Commun. 2021. PMID: 33795692 Free PMC article.
-
Humoral immune responses and neutralizing antibodies against SARS-CoV-2; implications in pathogenesis and protective immunity.Biochem Biophys Res Commun. 2021 Jan 29;538:187-191. doi: 10.1016/j.bbrc.2020.10.108. Epub 2020 Nov 7. Biochem Biophys Res Commun. 2021. PMID: 33187644 Free PMC article. Review.
-
Therapeutic antibodies for COVID-19: is a new age of IgM, IgA and bispecific antibodies coming?MAbs. 2022 Jan-Dec;14(1):2031483. doi: 10.1080/19420862.2022.2031483. MAbs. 2022. PMID: 35220888 Free PMC article. Review.
Cited by
-
Low quantity and quality of anti-spike humoral response is linked to CD4 T-cell apoptosis in COVID-19 patients.Cell Death Dis. 2022 Aug 27;13(8):741. doi: 10.1038/s41419-022-05190-0. Cell Death Dis. 2022. PMID: 36030261 Free PMC article.
-
Resistance of SARS-CoV-2 variants to neutralization by monoclonal and serum-derived polyclonal antibodies.Nat Med. 2021 Apr;27(4):717-726. doi: 10.1038/s41591-021-01294-w. Epub 2021 Mar 4. Nat Med. 2021. PMID: 33664494 Free PMC article.
-
Fc-mediated functions and the treatment of severe respiratory viral infections with passive immunotherapy - a balancing act.Front Immunol. 2023 Nov 22;14:1307398. doi: 10.3389/fimmu.2023.1307398. eCollection 2023. Front Immunol. 2023. PMID: 38077353 Free PMC article.
-
Evolution of functional antibodies following acute Epstein-Barr virus infection.PLoS Pathog. 2022 Sep 6;18(9):e1010738. doi: 10.1371/journal.ppat.1010738. eCollection 2022 Sep. PLoS Pathog. 2022. PMID: 36067220 Free PMC article.
-
SARS-CoV-2 BA.4/5 infection triggers more cross-reactive FcγRIIIa signaling and neutralization than BA.1, in the context of hybrid immunity.J Virol. 2024 Jul 23;98(7):e0067824. doi: 10.1128/jvi.00678-24. Epub 2024 Jul 2. J Virol. 2024. PMID: 38953380 Free PMC article.
References
-
- Akaike H. In: Second International Symposium on Information Theory. Petrov B.N., Caski F., editors. Akadémiai Kiadó; 1973. Information theory and an extension of the maximum likelihood principle; pp. 267–281.
-
- Bergqvist P., Stensson A., Lycke N.Y., Bemark M. T cell-independent IgA class switch recombination is restricted to the GALT and occurs prior to manifest germinal center formation. J. Immunol. 2010;184:3545–3553. - PubMed
Publication types
MeSH terms
Substances
Grants and funding
LinkOut - more resources
Full Text Sources
Other Literature Sources
Medical
Research Materials
Miscellaneous