TranSPHIRE: automated and feedback-optimized on-the-fly processing for cryo-EM
- PMID: 33177513
- PMCID: PMC7658977
- DOI: 10.1038/s41467-020-19513-2
TranSPHIRE: automated and feedback-optimized on-the-fly processing for cryo-EM
Abstract
Single particle cryo-EM requires full automation to allow high-throughput structure determination. Although software packages exist where parts of the cryo-EM pipeline are automated, a complete solution that offers reliable on-the-fly processing, resulting in high-resolution structures, does not exist. Here we present TranSPHIRE: A software package for fully-automated processing of cryo-EM datasets during data acquisition. TranSPHIRE transfers data from the microscope, automatically applies the common pre-processing steps, picks particles, performs 2D clustering, and 3D refinement parallel to image recording. Importantly, TranSPHIRE introduces a machine learning-based feedback loop to re-train its picking model to adapt to any given data set live during processing. This elegant approach enables TranSPHIRE to process data more effectively, producing high-quality particle stacks. TranSPHIRE collects and displays all metrics and microscope settings to allow users to quickly evaluate data during acquisition. TranSPHIRE can run on a single work station and also includes the automated processing of filaments.
Conflict of interest statement
The authors declare no competing interests.
Figures
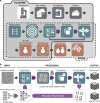
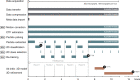
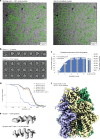
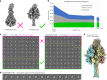
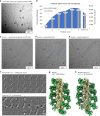
Similar articles
-
AutoCryoPicker: an unsupervised learning approach for fully automated single particle picking in Cryo-EM images.BMC Bioinformatics. 2019 Jun 13;20(1):326. doi: 10.1186/s12859-019-2926-y. BMC Bioinformatics. 2019. PMID: 31195977 Free PMC article.
-
A Super-Clustering Approach for Fully Automated Single Particle Picking in Cryo-EM.Genes (Basel). 2019 Aug 30;10(9):666. doi: 10.3390/genes10090666. Genes (Basel). 2019. PMID: 31480377 Free PMC article.
-
DeepCryoPicker: fully automated deep neural network for single protein particle picking in cryo-EM.BMC Bioinformatics. 2020 Nov 9;21(1):509. doi: 10.1186/s12859-020-03809-7. BMC Bioinformatics. 2020. PMID: 33167860 Free PMC article.
-
Routine Collection of High-Resolution cryo-EM Datasets Using 200 KV Transmission Electron Microscope.J Vis Exp. 2022 Mar 16;(181). doi: 10.3791/63519. J Vis Exp. 2022. PMID: 35377368 Review.
-
Automated pipelines for rapid evaluation during cryoEM data acquisition.Curr Opin Struct Biol. 2023 Dec;83:102729. doi: 10.1016/j.sbi.2023.102729. Epub 2023 Nov 21. Curr Opin Struct Biol. 2023. PMID: 37988815 Review.
Cited by
-
Automated vitrification of cryo-EM samples with controllable sample thickness using suction and real-time optical inspection.Nat Commun. 2022 May 27;13(1):2985. doi: 10.1038/s41467-022-30562-7. Nat Commun. 2022. PMID: 35624105 Free PMC article.
-
Structure of the peroxisomal Pex1/Pex6 ATPase complex bound to a substrate.Nat Commun. 2023 Sep 23;14(1):5942. doi: 10.1038/s41467-023-41640-9. Nat Commun. 2023. PMID: 37741838 Free PMC article.
-
Structure of the human KMN complex and implications for regulation of its assembly.Nat Struct Mol Biol. 2024 Jun;31(6):861-873. doi: 10.1038/s41594-024-01230-9. Epub 2024 Mar 8. Nat Struct Mol Biol. 2024. PMID: 38459128 Free PMC article.
-
Structural basis of TRPC4 regulation by calmodulin and pharmacological agents.Elife. 2020 Nov 25;9:e60603. doi: 10.7554/eLife.60603. Elife. 2020. PMID: 33236980 Free PMC article.
-
Design of an ultrafast pulsed ponderomotive phase plate for cryo-electron tomography.Cell Rep Methods. 2023 Jan 12;3(1):100387. doi: 10.1016/j.crmeth.2022.100387. eCollection 2023 Jan 23. Cell Rep Methods. 2023. PMID: 36814846 Free PMC article.
References
-
- Method of the Year 2015. Nat Methods 13, 1 10.1038/nmeth.3730 (2016). - PubMed
-
- Merino, F. & Raunser, S. Cryo-EM as a tool for structure-based drug development. Angewandte Chemie.10.1002/ange.201608432 (2016). - PubMed
-
- Pospich, S., Merino, F. & Raunser, S. Structural effects and functional implications of phalloidin and jasplakinolide binding to actin filaments. Structure. 10.1016/j.str.2020.01.014 (2020) - PubMed
Publication types
LinkOut - more resources
Full Text Sources