Aligning SARS-CoV-2 indicators via an epidemic model: application to hospital admissions and RNA detection in sewage sludge
- PMID: 33111195
- PMCID: PMC7592141
- DOI: 10.1007/s10729-020-09525-1
Aligning SARS-CoV-2 indicators via an epidemic model: application to hospital admissions and RNA detection in sewage sludge
Abstract
Ascertaining the state of coronavirus outbreaks is crucial for public health decision-making. Absent repeated representative viral test samples in the population, public health officials and researchers alike have relied on lagging indicators of infection to make inferences about the direction of the outbreak and attendant policy decisions. Recently researchers have shown that SARS-CoV-2 RNA can be detected in municipal sewage sludge with measured RNA concentrations rising and falling suggestively in the shape of an epidemic curve while providing an earlier signal of infection than hospital admissions data. The present paper presents a SARS-CoV-2 epidemic model to serve as a basis for estimating the incidence of infection, and shows mathematically how modeled transmission dynamics translate into infection indicators by incorporating probability distributions for indicator-specific time lags from infection. Hospital admissions and SARS-CoV-2 RNA in municipal sewage sludge are simultaneously modeled via maximum likelihood scaling to the underlying transmission model. The results demonstrate that both data series plausibly follow from the transmission model specified and provide a 95% confidence interval estimate of the reproductive number R0 ≈ 2.4 ± 0.2. Sensitivity analysis accounting for alternative lag distributions from infection until hospitalization and sludge RNA concentration respectively suggests that the detection of viral RNA in sewage sludge leads hospital admissions by 3 to 5 days on average. The analysis suggests that stay-at-home restrictions plausibly removed 89% of the population from the risk of infection with the remaining 11% exposed to an unmitigated outbreak that infected 9.3% of the total population.
Keywords: COVID-19; COVID-19 hospital admissions; Epidemic indicators; Probability model; SARS-CoV-2; Sewage sludge viral RNA concentration; Wastewater epidemiology.
Figures
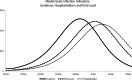
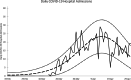
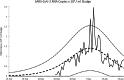
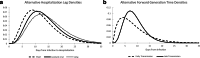
Similar articles
-
Measurement of SARS-CoV-2 RNA in wastewater tracks community infection dynamics.Nat Biotechnol. 2020 Oct;38(10):1164-1167. doi: 10.1038/s41587-020-0684-z. Epub 2020 Sep 18. Nat Biotechnol. 2020. PMID: 32948856 Free PMC article.
-
Municipal wastewater viral pollution in Saudi Arabia: effect of hot climate on COVID-19 disease spreading.Environ Sci Pollut Res Int. 2023 Feb;30(10):25050-25057. doi: 10.1007/s11356-021-14809-2. Epub 2021 Jun 17. Environ Sci Pollut Res Int. 2023. PMID: 34138435 Free PMC article.
-
Longitudinal wastewater surveillance of four key pathogens during an unprecedented large-scale COVID-19 outbreak in China facilitated a novel strategy for addressing public health priorities-A proof of concept study.Water Res. 2023 Dec 1;247:120751. doi: 10.1016/j.watres.2023.120751. Epub 2023 Oct 19. Water Res. 2023. PMID: 37918201
-
Occurrence of viruses in sewage sludge: A systematic review.Sci Total Environ. 2022 Jun 10;824:153886. doi: 10.1016/j.scitotenv.2022.153886. Epub 2022 Feb 16. Sci Total Environ. 2022. PMID: 35182626 Free PMC article. Review.
-
[Polio Environmental Surveillance and Its Application to SARS-CoV-2 Detection].Yakugaku Zasshi. 2022;142(1):11-15. doi: 10.1248/yakushi.21-00161-1. Yakugaku Zasshi. 2022. PMID: 34980746 Review. Japanese.
Cited by
-
Observations of Respiratory Syncytial Virus (RSV) Nucleic Acids in Wastewater Solids Across the United States in the 2022-2023 Season: Relationships with RSV Infection Positivity and Hospitalization Rates.ACS ES T Water. 2024 Feb 29;4(4):1657-1667. doi: 10.1021/acsestwater.3c00725. eCollection 2024 Apr 12. ACS ES T Water. 2024. PMID: 38633368 Free PMC article.
-
Trends in SARS-CoV-2 clinically confirmed cases and viral load in wastewater: A critical alignment for Padua city (NE Italy).Heliyon. 2023 Sep 29;9(10):e20571. doi: 10.1016/j.heliyon.2023.e20571. eCollection 2023 Oct. Heliyon. 2023. PMID: 37822618 Free PMC article.
-
Wastewater-based epidemiology predicts COVID-19-induced weekly new hospital admissions in over 150 USA counties.Nat Commun. 2023 Jul 28;14(1):4548. doi: 10.1038/s41467-023-40305-x. Nat Commun. 2023. PMID: 37507407 Free PMC article.
-
An exploration of the relationship between wastewater viral signals and COVID-19 hospitalizations in Ottawa, Canada.Infect Dis Model. 2023 Sep;8(3):617-631. doi: 10.1016/j.idm.2023.05.011. Epub 2023 Jun 7. Infect Dis Model. 2023. PMID: 37342365 Free PMC article.
-
Monitoring of COVID-19 in wastewater across the Eastern Upper Peninsula of Michigan.Environ Adv. 2023 Apr;11:100326. doi: 10.1016/j.envadv.2022.100326. Epub 2022 Dec 1. Environ Adv. 2023. PMID: 36471702 Free PMC article.
References
-
- CDC (2020) COVID-19 Pandemic Planning Scenarios. United States Centers for Disease Control and Prevention. https://www.cdc.gov/coronavirus/2019-ncov/hcp/planning-scenarios.html. Accessed 27 Sept 2020
-
- Cox DR, Hinkley DV. Theoretical statistics. Chapman and Hall: London; 1974.
-
- Ferguson N, Laydon D, Gemma N-G , et al. (2020) Impact of non-pharmaceutical interventions (NPIs) to reduce COVID-19 mortality and healthcare demand. Imperial College COVID-19 Response Team, March 16, 2020. https://tinyurl.com/tcdy42y. Accessed 27 Sept 2020
MeSH terms
Substances
LinkOut - more resources
Full Text Sources
Medical
Miscellaneous