Discriminatory Power of Combinatorial Antigen Recognition in Cancer T Cell Therapies
- PMID: 32916097
- PMCID: PMC7814417
- DOI: 10.1016/j.cels.2020.08.002
Discriminatory Power of Combinatorial Antigen Recognition in Cancer T Cell Therapies
Abstract
Precise discrimination of tumor from normal tissues remains a major roadblock for therapeutic efficacy of chimeric antigen receptor (CAR) T cells. Here, we perform a comprehensive in silico screen to identify multi-antigen signatures that improve tumor discrimination by CAR T cells engineered to integrate multiple antigen inputs via Boolean logic, e.g., AND and NOT. We screen >2.5 million dual antigens and ∼60 million triple antigens across 33 tumor types and 34 normal tissues. We find that dual antigens significantly outperform the best single clinically investigated CAR targets and confirm key predictions experimentally. Further, we identify antigen triplets that are predicted to show close to ideal tumor-versus-normal tissue discrimination for several tumor types. This work demonstrates the potential of 2- to 3-antigen Boolean logic gates for improving tumor discrimination by CAR T cell therapies. Our predictions are available on an interactive web server resource (antigen.princeton.edu).
Keywords: AND gate; CAR T cell; NOT gate; T cell therapeutics; combinatorial antigen recognition; tumor antigens; tumor-versus-normal discrimination.
Copyright © 2020. Published by Elsevier Inc.
Conflict of interest statement
Declaration of Interests W.A.L. is on the Scientific Advisory Board for Allogene Therapeutics and O.G.T. is on the Scientific Advisory Board for Caris Life Sciences. W.A.L. and O.G.T. have filed patents related to this work.
Figures
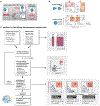
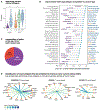
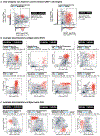
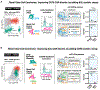
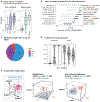
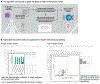
Comment in
-
The Most Logical Approach to Improve CAR T Cell Therapy.Cell Syst. 2020 Nov 18;11(5):421-423. doi: 10.1016/j.cels.2020.10.008. Cell Syst. 2020. PMID: 33212015
Similar articles
-
Co-expression patterns of chimeric antigen receptor (CAR)-T cell target antigens in primary and recurrent ovarian cancer.Gynecol Oncol. 2021 Feb;160(2):520-529. doi: 10.1016/j.ygyno.2020.12.005. Epub 2020 Dec 17. Gynecol Oncol. 2021. PMID: 33342620
-
Single-cell mapping of combinatorial target antigens for CAR switches using logic gates.Nat Biotechnol. 2023 Nov;41(11):1593-1605. doi: 10.1038/s41587-023-01686-y. Epub 2023 Feb 16. Nat Biotechnol. 2023. PMID: 36797491
-
Implementing Logic Gates for Safer Immunotherapy of Cancer.Front Immunol. 2021 Nov 4;12:780399. doi: 10.3389/fimmu.2021.780399. eCollection 2021. Front Immunol. 2021. PMID: 34804073 Free PMC article. Review.
-
Effective Targeting of TAG72+ Peritoneal Ovarian Tumors via Regional Delivery of CAR-Engineered T Cells.Front Immunol. 2018 Nov 19;9:2268. doi: 10.3389/fimmu.2018.02268. eCollection 2018. Front Immunol. 2018. PMID: 30510550 Free PMC article.
-
Chimeric antigen receptor-engineered T-cell therapy for liver cancer.Hepatobiliary Pancreat Dis Int. 2018 Aug;17(4):301-309. doi: 10.1016/j.hbpd.2018.05.005. Epub 2018 May 24. Hepatobiliary Pancreat Dis Int. 2018. PMID: 29861325 Review.
Cited by
-
How Do We Meet the Challenge of Chimeric Antigen Receptor T-Cell Therapy for Solid Tumors?Cancer J. 2021 Mar-Apr 01;27(2):134-142. doi: 10.1097/PPO.0000000000000516. Cancer J. 2021. PMID: 33750073 Free PMC article. Review.
-
The future of engineered immune cell therapies.Science. 2022 Nov 25;378(6622):853-858. doi: 10.1126/science.abq6990. Epub 2022 Nov 24. Science. 2022. PMID: 36423279 Free PMC article. Review.
-
CAR-T Cell Therapy for Acute Myeloid Leukemia: Preclinical Rationale, Current Clinical Progress, and Barriers to Success.BioDrugs. 2021 May;35(3):281-302. doi: 10.1007/s40259-021-00477-8. Epub 2021 Apr 7. BioDrugs. 2021. PMID: 33826079 Review.
-
Synthetic receptors for logic gated T cell recognition and function.Curr Opin Immunol. 2022 Feb;74:9-17. doi: 10.1016/j.coi.2021.09.003. Epub 2021 Sep 24. Curr Opin Immunol. 2022. PMID: 34571290 Free PMC article. Review.
-
Targeting solid tumor antigens with chimeric receptors: cancer biology meets synthetic immunology.Trends Cancer. 2024 Apr;10(4):312-331. doi: 10.1016/j.trecan.2024.01.003. Epub 2024 Feb 13. Trends Cancer. 2024. PMID: 38355356 Review.
References
Publication types
MeSH terms
Substances
Grants and funding
LinkOut - more resources
Full Text Sources
Other Literature Sources