Systems-Level Immunomonitoring from Acute to Recovery Phase of Severe COVID-19
- PMID: 32838342
- PMCID: PMC7405891
- DOI: 10.1016/j.xcrm.2020.100078
Systems-Level Immunomonitoring from Acute to Recovery Phase of Severe COVID-19
Abstract
Severe disease of SARS-CoV-2 is characterized by vigorous inflammatory responses in the lung, often with a sudden onset after 5-7 days of stable disease. Efforts to modulate this hyperinflammation and the associated acute respiratory distress syndrome rely on the unraveling of the immune cell interactions and cytokines that drive such responses. Given that every patient is captured at different stages of infection, longitudinal monitoring of the immune response is critical and systems-level analyses are required to capture cellular interactions. Here, we report on a systems-level blood immunomonitoring study of 37 adult patients diagnosed with COVID-19 and followed with up to 14 blood samples from acute to recovery phases of the disease. We describe an IFNγ-eosinophil axis activated before lung hyperinflammation and changes in cell-cell co-regulation during different stages of the disease. We also map an immune trajectory during recovery that is shared among patients with severe COVID-19.
Keywords: COVID-19; SARS-CoV-2; human immunology; mass cytometry; plasma proteins; systems immunology.
© 2020 The Author(s).
Conflict of interest statement
P.B., T.L., and J.M. are the founders of Cytodelics AB, a company that commercializes reagents for blood sample preservation as used in this study.
Figures
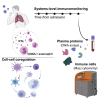
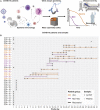
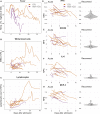
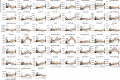
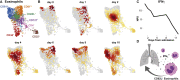
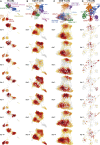
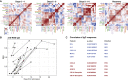
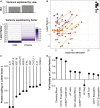
Similar articles
-
SARS-CoV-2-Specific Antibody and T Cell Response Kinetics According to Symptom Severity.Am J Trop Med Hyg. 2021 Jun 17;105(2):395-400. doi: 10.4269/ajtmh.20-1594. Am J Trop Med Hyg. 2021. PMID: 34143752 Free PMC article.
-
Chemokines and eicosanoids fuel the hyperinflammation within the lungs of patients with severe COVID-19.J Allergy Clin Immunol. 2021 Aug;148(2):368-380.e3. doi: 10.1016/j.jaci.2021.05.032. Epub 2021 Jun 7. J Allergy Clin Immunol. 2021. PMID: 34111453 Free PMC article.
-
Longitudinal assessment of anti-SARS-CoV-2 antibody dynamics and clinical features following convalescence from a COVID-19 infection.Int J Infect Dis. 2021 Jun;107:221-227. doi: 10.1016/j.ijid.2021.04.080. Epub 2021 Apr 28. Int J Infect Dis. 2021. PMID: 33932604 Free PMC article.
-
Basophils and Mast Cells in COVID-19 Pathogenesis.Cells. 2021 Oct 14;10(10):2754. doi: 10.3390/cells10102754. Cells. 2021. PMID: 34685733 Free PMC article. Review.
-
Insights into COVID-19 Vaccine Development Based on Immunogenic Structural Proteins of SARS-CoV-2, Host Immune Responses, and Herd Immunity.Cells. 2021 Oct 29;10(11):2949. doi: 10.3390/cells10112949. Cells. 2021. PMID: 34831172 Free PMC article. Review.
Cited by
-
Immunologic mediators profile in COVID-19 convalescence.Sci Rep. 2024 Sep 9;14(1):20930. doi: 10.1038/s41598-024-71419-x. Sci Rep. 2024. PMID: 39251702 Free PMC article.
-
Longitudinal analysis of the lung proteome reveals persistent repair months after mild to moderate COVID-19.Cell Rep Med. 2024 Jul 16;5(7):101642. doi: 10.1016/j.xcrm.2024.101642. Epub 2024 Jul 8. Cell Rep Med. 2024. PMID: 38981485 Free PMC article.
-
Innate Immunity in Protection and Pathogenesis During Coronavirus Infections and COVID-19.Annu Rev Immunol. 2024 Jun;42(1):615-645. doi: 10.1146/annurev-immunol-083122-043545. Annu Rev Immunol. 2024. PMID: 38941608 Review.
-
PD-L1- and IL-4-expressing basophils promote pathogenic accumulation of T follicular helper cells in lupus.Nat Commun. 2024 Apr 22;15(1):3389. doi: 10.1038/s41467-024-47691-w. Nat Commun. 2024. PMID: 38649353 Free PMC article.
-
Integrated Bioinformatics Exploration and Preliminary Clinical Verification for the Identification of Crucial Biomarkers in Severe Cases of COVID-19.J Inflamm Res. 2024 Mar 11;17:1561-1576. doi: 10.2147/JIR.S454284. eCollection 2024. J Inflamm Res. 2024. PMID: 38495341 Free PMC article.
References
-
- Medzhitov R., Janeway C.A., Jr. Decoding the patterns of self and nonself by the innate immune system. Science. 2002;296:298–300. - PubMed
Publication types
MeSH terms
Substances
LinkOut - more resources
Full Text Sources
Other Literature Sources
Medical
Miscellaneous