TRANSPIRE: A Computational Pipeline to Elucidate Intracellular Protein Movements from Spatial Proteomics Data Sets
- PMID: 32401031
- PMCID: PMC7737664
- DOI: 10.1021/jasms.0c00033
TRANSPIRE: A Computational Pipeline to Elucidate Intracellular Protein Movements from Spatial Proteomics Data Sets
Abstract
Protein localization is paramount to protein function, and the intracellular movement of proteins underlies the regulation of numerous cellular processes. Given advances in spatial proteomics, the investigation of protein localization at a global scale has become attainable. Also becoming apparent is the need for dedicated analytical frameworks that allow the discovery of global intracellular protein movement events. Here, we describe TRANSPIRE, a computational pipeline that facilitates TRanslocation ANalysis of SPatIal pRotEomics data sets. TRANSPIRE leverages synthetic translocation profiles generated from organelle marker proteins to train a probabilistic Gaussian process classifier that predicts changes in protein distribution. This output is then integrated with information regarding co-translocating proteins and complexes and enriched gene ontology associations to discern the putative regulation and function of movement. We validate TRANSPIRE performance for predicting nuclear-cytoplasmic shuttling events. Analyzing an existing data set of nuclear and cytoplasmic proteomes during Kaposi Sarcoma-associated herpesvirus (KSHV)-induced cellular mRNA decay, we confirm that TRANSPIRE readily discerns expected translocations of RNA binding proteins. We next investigate protein translocations during infection with human cytomegalovirus (HCMV), a β-herpesvirus known to induce global organelle remodeling. We find that HCMV infection induces broad changes in protein localization, with over 800 proteins predicted to translocate during virus replication. Evident are protein movements related to HCMV modulation of host defense, metabolism, cellular trafficking, and Wnt signaling. For example, the low-density lipoprotein receptor (LDLR) translocates to the lysosome early in infection in conjunction with its degradation, which we validate by targeted mass spectrometry. Using microscopy, we also validate the translocation of the multifunctional kinase DAPK3, a movement that may contribute to HCMV activation of Wnt signaling.
Keywords: machine learning; protein translocation; spatial proteomics; subcellular organelles; viral infection.
Conflict of interest statement
The authors declare no competing financial interest.
Figures
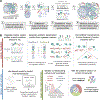
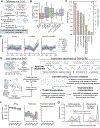
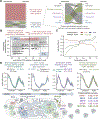
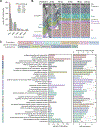
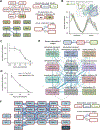
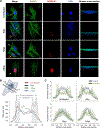
Similar articles
-
Location is everything: protein translocations as a viral infection strategy.Curr Opin Chem Biol. 2019 Feb;48:34-43. doi: 10.1016/j.cbpa.2018.09.021. Epub 2018 Oct 16. Curr Opin Chem Biol. 2019. PMID: 30339987 Free PMC article. Review.
-
Dynamic Organellar Maps for Spatial Proteomics.Curr Protoc Cell Biol. 2019 Jun;83(1):e81. doi: 10.1002/cpcb.81. Epub 2018 Nov 29. Curr Protoc Cell Biol. 2019. PMID: 30489039
-
A Portrait of the Human Organelle Proteome In Space and Time during Cytomegalovirus Infection.Cell Syst. 2016 Oct 26;3(4):361-373.e6. doi: 10.1016/j.cels.2016.08.012. Epub 2016 Sep 15. Cell Syst. 2016. PMID: 27641956 Free PMC article.
-
Human cytomegalovirus pUL97 kinase induces global changes in the infected cell phosphoproteome.Proteomics. 2015 Jun;15(12):2006-22. doi: 10.1002/pmic.201400607. Epub 2015 May 12. Proteomics. 2015. PMID: 25867546 Free PMC article.
-
Spatial proteomics: a powerful discovery tool for cell biology.Nat Rev Mol Cell Biol. 2019 May;20(5):285-302. doi: 10.1038/s41580-018-0094-y. Nat Rev Mol Cell Biol. 2019. PMID: 30659282 Review.
Cited by
-
Inferring differential subcellular localisation in comparative spatial proteomics using BANDLE.Nat Commun. 2022 Oct 10;13(1):5948. doi: 10.1038/s41467-022-33570-9. Nat Commun. 2022. PMID: 36216816 Free PMC article.
-
Spatial proteomics reveals subcellular reorganization in human keratinocytes exposed to UVA light.iScience. 2022 Mar 16;25(4):104093. doi: 10.1016/j.isci.2022.104093. eCollection 2022 Apr 15. iScience. 2022. PMID: 35372811 Free PMC article.
-
Comparative Analysis of T-Cell Spatial Proteomics and the Influence of HIV Expression.Mol Cell Proteomics. 2022 Mar;21(3):100194. doi: 10.1016/j.mcpro.2022.100194. Epub 2022 Jan 8. Mol Cell Proteomics. 2022. PMID: 35017099 Free PMC article.
-
Integrative multi-omics and systems bioinformatics in translational neuroscience: A data mining perspective.J Pharm Anal. 2023 Aug;13(8):836-850. doi: 10.1016/j.jpha.2023.06.011. Epub 2023 Jun 30. J Pharm Anal. 2023. PMID: 37719197 Free PMC article. Review.
-
Subcellular Transcriptomics and Proteomics: A Comparative Methods Review.Mol Cell Proteomics. 2022 Feb;21(2):100186. doi: 10.1016/j.mcpro.2021.100186. Epub 2021 Dec 16. Mol Cell Proteomics. 2022. PMID: 34922010 Free PMC article. Review.
References
-
- Hetz C; Papa FR The Unfolded Protein Response and Cell Fate Control. Mol. Cell 2018, 69, 169–181. - PubMed
MeSH terms
Substances
Grants and funding
LinkOut - more resources
Full Text Sources