Single-cell ATAC-Seq in human pancreatic islets and deep learning upscaling of rare cells reveals cell-specific type 2 diabetes regulatory signatures
- PMID: 32029221
- PMCID: PMC6961712
- DOI: 10.1016/j.molmet.2019.12.006
Single-cell ATAC-Seq in human pancreatic islets and deep learning upscaling of rare cells reveals cell-specific type 2 diabetes regulatory signatures
Abstract
Objective: Type 2 diabetes (T2D) is a complex disease characterized by pancreatic islet dysfunction, insulin resistance, and disruption of blood glucose levels. Genome-wide association studies (GWAS) have identified > 400 independent signals that encode genetic predisposition. More than 90% of associated single-nucleotide polymorphisms (SNPs) localize to non-coding regions and are enriched in chromatin-defined islet enhancer elements, indicating a strong transcriptional regulatory component to disease susceptibility. Pancreatic islets are a mixture of cell types that express distinct hormonal programs, so each cell type may contribute differentially to the underlying regulatory processes that modulate T2D-associated transcriptional circuits. Existing chromatin profiling methods such as ATAC-seq and DNase-seq, applied to islets in bulk, produce aggregate profiles that mask important cellular and regulatory heterogeneity.
Methods: We present genome-wide single-cell chromatin accessibility profiles in >1,600 cells derived from a human pancreatic islet sample using single-cell combinatorial indexing ATAC-seq (sci-ATAC-seq). We also developed a deep learning model based on U-Net architecture to accurately predict open chromatin peak calls in rare cell populations.
Results: We show that sci-ATAC-seq profiles allow us to deconvolve alpha, beta, and delta cell populations and identify cell-type-specific regulatory signatures underlying T2D. Particularly, T2D GWAS SNPs are significantly enriched in beta cell-specific and across cell-type shared islet open chromatin, but not in alpha or delta cell-specific open chromatin. We also demonstrate, using less abundant delta cells, that deep learning models can improve signal recovery and feature reconstruction of rarer cell populations. Finally, we use co-accessibility measures to nominate the cell-specific target genes at 104 non-coding T2D GWAS signals.
Conclusions: Collectively, we identify the islet cell type of action across genetic signals of T2D predisposition and provide higher-resolution mechanistic insights into genetically encoded risk pathways.
Keywords: Chromatin; Deep learning; Epigenomics; Islet; Single cell; Type 2 diabetes.
Published by Elsevier GmbH.
Figures
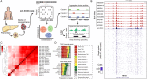
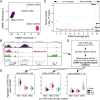
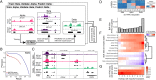
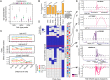
Similar articles
-
Genetic regulatory signatures underlying islet gene expression and type 2 diabetes.Proc Natl Acad Sci U S A. 2017 Feb 28;114(9):2301-2306. doi: 10.1073/pnas.1621192114. Epub 2017 Feb 13. Proc Natl Acad Sci U S A. 2017. PMID: 28193859 Free PMC article.
-
ATAC-seq reveals alterations in open chromatin in pancreatic islets from subjects with type 2 diabetes.Sci Rep. 2019 May 23;9(1):7785. doi: 10.1038/s41598-019-44076-8. Sci Rep. 2019. PMID: 31123324 Free PMC article.
-
Single-cell chromatin accessibility identifies pancreatic islet cell type- and state-specific regulatory programs of diabetes risk.Nat Genet. 2021 Apr;53(4):455-466. doi: 10.1038/s41588-021-00823-0. Epub 2021 Apr 1. Nat Genet. 2021. PMID: 33795864 Free PMC article.
-
(Epi)genomic heterogeneity of pancreatic islet function and failure in type 2 diabetes.Mol Metab. 2019 Sep;27S(Suppl):S15-S24. doi: 10.1016/j.molmet.2019.06.002. Mol Metab. 2019. PMID: 31500827 Free PMC article. Review.
-
Genomics of Islet (Dys)function and Type 2 Diabetes.Trends Genet. 2017 Apr;33(4):244-255. doi: 10.1016/j.tig.2017.01.010. Epub 2017 Feb 27. Trends Genet. 2017. PMID: 28245910 Free PMC article. Review.
Cited by
-
A genetic map of the mouse dorsal vagal complex and its role in obesity.Nat Metab. 2021 Apr;3(4):530-545. doi: 10.1038/s42255-021-00363-1. Epub 2021 Mar 25. Nat Metab. 2021. PMID: 33767443
-
The missing link between genetic association and regulatory function.Elife. 2022 Dec 14;11:e74970. doi: 10.7554/eLife.74970. Elife. 2022. PMID: 36515579 Free PMC article.
-
Fundamental and practical approaches for single-cell ATAC-seq analysis.aBIOTECH. 2022 Sep 27;3(3):212-223. doi: 10.1007/s42994-022-00082-5. eCollection 2022 Sep. aBIOTECH. 2022. PMID: 36313930 Free PMC article. Review.
-
Single-cell multi-omics analysis of human pancreatic islets reveals novel cellular states in type 1 diabetes.Nat Metab. 2022 Feb;4(2):284-299. doi: 10.1038/s42255-022-00531-x. Epub 2022 Feb 28. Nat Metab. 2022. PMID: 35228745 Free PMC article.
-
The Human Islet: Mini-Organ With Mega-Impact.Endocr Rev. 2021 Sep 28;42(5):605-657. doi: 10.1210/endrev/bnab010. Endocr Rev. 2021. PMID: 33844836 Free PMC article. Review.
References
-
- DeFronzo R.A., Ferrannini E., Groop L., Henry R.R., Herman W.H., Holst J.J. Type 2 diabetes mellitus. Nature reviews Disease primers. 2015;1:15019. - PubMed
-
- Spellman C.W. Pathophysiology of type 2 diabetes: targeting islet cell dysfunction. Journal of the American Osteopathic Association. 2010;110:S2–S7. - PubMed
-
- Viñuela A., Varshney A., van de Bunt M., Prasad R.B., Asplund O.B., Bennett A. Influence of genetic variants on gene expression in human pancreatic islets – implications for type 2 diabetes. BioRxiv. 2019:655670.
Publication types
MeSH terms
Grants and funding
LinkOut - more resources
Full Text Sources
Other Literature Sources
Medical