The landscape of viral associations in human cancers
- PMID: 32025001
- PMCID: PMC8076016
- DOI: 10.1038/s41588-019-0558-9
The landscape of viral associations in human cancers
Erratum in
-
Author Correction: The landscape of viral associations in human cancers.Nat Genet. 2023 Jun;55(6):1077. doi: 10.1038/s41588-023-01316-y. Nat Genet. 2023. PMID: 36944734 Free PMC article. No abstract available.
Abstract
Here, as part of the Pan-Cancer Analysis of Whole Genomes (PCAWG) Consortium, for which whole-genome and-for a subset-whole-transcriptome sequencing data from 2,658 cancers across 38 tumor types was aggregated, we systematically investigated potential viral pathogens using a consensus approach that integrated three independent pipelines. Viruses were detected in 382 genome and 68 transcriptome datasets. We found a high prevalence of known tumor-associated viruses such as Epstein-Barr virus (EBV), hepatitis B virus (HBV) and human papilloma virus (HPV; for example, HPV16 or HPV18). The study revealed significant exclusivity of HPV and driver mutations in head-and-neck cancer and the association of HPV with APOBEC mutational signatures, which suggests that impaired antiviral defense is a driving force in cervical, bladder and head-and-neck carcinoma. For HBV, HPV16, HPV18 and adeno-associated virus-2 (AAV2), viral integration was associated with local variations in genomic copy numbers. Integrations at the TERT promoter were associated with high telomerase expression evidently activating this tumor-driving process. High levels of endogenous retrovirus (ERV1) expression were linked to a worse survival outcome in patients with kidney cancer.
Conflict of interest statement
The authors declare no competing interests.
Figures
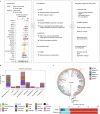
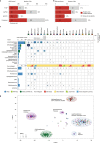
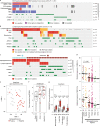
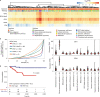
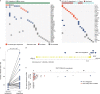
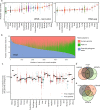
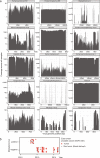
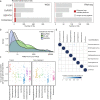
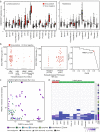
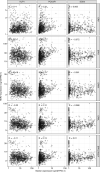
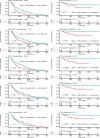
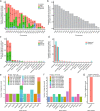
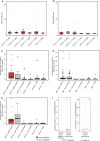
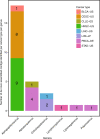
Similar articles
-
The viral expression and immune status in human cancers and insights into novel biomarkers of immunotherapy.BMC Cancer. 2021 Nov 5;21(1):1183. doi: 10.1186/s12885-021-08871-9. BMC Cancer. 2021. PMID: 34740324 Free PMC article.
-
A virome-wide clonal integration analysis platform for discovering cancer viral etiology.Genome Res. 2019 May;29(5):819-830. doi: 10.1101/gr.242529.118. Epub 2019 Mar 14. Genome Res. 2019. PMID: 30872350 Free PMC article.
-
Integrations of the hepatitis B virus (HBV) and human papillomavirus (HPV) into the human telomerase reverse transcriptase (hTERT) gene in liver and cervical cancers.Oncogene. 2003 Jun 12;22(24):3813-20. doi: 10.1038/sj.onc.1206528. Oncogene. 2003. PMID: 12802289
-
Human tumor-associated viruses and new insights into the molecular mechanisms of cancer.Oncogene. 2008 Dec;27 Suppl 2:S31-42. doi: 10.1038/onc.2009.351. Oncogene. 2008. PMID: 19956178 Review.
-
Regulation of telomerase and telomeres: human tumor viruses take control.J Natl Cancer Inst. 2008 Jan 16;100(2):98-108. doi: 10.1093/jnci/djm269. Epub 2008 Jan 8. J Natl Cancer Inst. 2008. PMID: 18182620 Review.
Cited by
-
When Do Tumours Develop? Neoplastic Processes Across Different Timescales: Age, Season and Round the Circadian Clock.Evol Appl. 2024 Oct 22;17(10):e70024. doi: 10.1111/eva.70024. eCollection 2024 Oct. Evol Appl. 2024. PMID: 39444444 Free PMC article. Review.
-
Clinical implications of cytomegalovirus in glioblastoma progression and therapy.NPJ Precis Oncol. 2024 Sep 29;8(1):213. doi: 10.1038/s41698-024-00709-4. NPJ Precis Oncol. 2024. PMID: 39343770 Free PMC article. Review.
-
Uncovering the link between human endogenous retroviruses, inflammatory pathways, and gastric cancer development.Cancer Biomark. 2024;41(2):103-113. doi: 10.3233/CBM-230417. Cancer Biomark. 2024. PMID: 39331091 Free PMC article.
-
Virology-The next fifty years.Cell. 2024 Sep 19;187(19):5128-5145. doi: 10.1016/j.cell.2024.07.025. Cell. 2024. PMID: 39303682
-
Beyond the Gut: The intratumoral microbiome's influence on tumorigenesis and treatment response.Cancer Commun (Lond). 2024 Oct;44(10):1130-1167. doi: 10.1002/cac2.12597. Epub 2024 Aug 1. Cancer Commun (Lond). 2024. PMID: 39087354 Free PMC article. Review.
References
Publication types
MeSH terms
Substances
Grants and funding
LinkOut - more resources
Full Text Sources
Other Literature Sources