Intermittent Hypoxia and Hypercapnia Reproducibly Change the Gut Microbiome and Metabolome across Rodent Model Systems
- PMID: 31058230
- PMCID: PMC6495231
- DOI: 10.1128/mSystems.00058-19
Intermittent Hypoxia and Hypercapnia Reproducibly Change the Gut Microbiome and Metabolome across Rodent Model Systems
Abstract
Studying perturbations in the gut ecosystem using animal models of disease continues to provide valuable insights into the role of the microbiome in various pathological conditions. However, understanding whether these changes are consistent across animal models of different genetic backgrounds, and hence potentially translatable to human populations, remains a major unmet challenge in the field. Nonetheless, in relatively limited cases have the same interventions been studied in two animal models in the same laboratory. Moreover, such studies typically examine a single data layer and time point. Here, we show the power of utilizing time series microbiome (16S rRNA amplicon profiling) and metabolome (untargeted liquid chromatography-tandem mass spectrometry [LC-MS/MS]) data to relate two different mouse models of atherosclerosis-ApoE-/- (n = 24) and Ldlr-/- (n = 16)-that are exposed to intermittent hypoxia and hypercapnia (IHH) longitudinally (for 10 and 6 weeks, respectively) to model chronic obstructive sleep apnea. Using random forest classifiers trained on each data layer, we show excellent accuracy in predicting IHH exposure within ApoE-/- and Ldlr-/- knockout models and in cross-applying predictive features found in one animal model to the other. The key microbes and metabolites that reproducibly predicted IHH exposure included bacterial species from the families Mogibacteriaceae, Clostridiaceae, bile acids, and fatty acids, providing a refined set of biomarkers associated with IHH. The results highlight that time series multiomics data can be used to relate different animal models of disease using supervised machine learning techniques and can provide a pathway toward identifying robust microbiome and metabolome features that underpin translation from animal models to human disease. IMPORTANCE Reproducibility of microbiome research is a major topic of contemporary interest. Although it is often possible to distinguish individuals with specific diseases within a study, the differences are often inconsistent across cohorts, often due to systematic variation in analytical conditions. Here we study the same intervention in two different mouse models of cardiovascular disease (atherosclerosis) by profiling the microbiome and metabolome in stool specimens over time. We demonstrate that shared microbial and metabolic changes are involved in both models with the intervention. We then introduce a pipeline for finding similar results in other studies. This work will help find common features identified across different model systems that are most likely to apply in humans.
Keywords: cardiovascular; machine learning; metabolism; microbiome; sleep apnea.
Figures
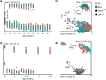
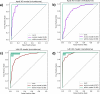
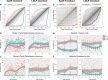
Similar articles
-
Intermittent Hypoxia and Hypercapnia, a Hallmark of Obstructive Sleep Apnea, Alters the Gut Microbiome and Metabolome.mSystems. 2018 Jun 5;3(3):e00020-18. doi: 10.1128/mSystems.00020-18. eCollection 2018 May-Jun. mSystems. 2018. PMID: 29896566 Free PMC article.
-
Intermittent Hypoxia and Hypercapnia Alter Diurnal Rhythms of Luminal Gut Microbiome and Metabolome.mSystems. 2021 Jun 29;6(3):e0011621. doi: 10.1128/mSystems.00116-21. Online ahead of print. mSystems. 2021. PMID: 34184915 Free PMC article.
-
Influence of Intermittent Hypoxia/Hypercapnia on Atherosclerosis, Gut Microbiome, and Metabolome.Front Physiol. 2021 Apr 8;12:663950. doi: 10.3389/fphys.2021.663950. eCollection 2021. Front Physiol. 2021. PMID: 33897472 Free PMC article.
-
Translational Metabolomics of Head Injury: Exploring Dysfunctional Cerebral Metabolism with Ex Vivo NMR Spectroscopy-Based Metabolite Quantification.In: Kobeissy FH, editor. Brain Neurotrauma: Molecular, Neuropsychological, and Rehabilitation Aspects. Boca Raton (FL): CRC Press/Taylor & Francis; 2015. Chapter 25. In: Kobeissy FH, editor. Brain Neurotrauma: Molecular, Neuropsychological, and Rehabilitation Aspects. Boca Raton (FL): CRC Press/Taylor & Francis; 2015. Chapter 25. PMID: 26269925 Free Books & Documents. Review.
-
Metabolic dysfunction in OSA: Is there something new under the sun?J Sleep Res. 2022 Feb;31(1):e13418. doi: 10.1111/jsr.13418. Epub 2021 Jun 21. J Sleep Res. 2022. PMID: 34152053 Review.
Cited by
-
Time of sample collection is critical for the replicability of microbiome analyses.Nat Metab. 2024 Jul;6(7):1282-1293. doi: 10.1038/s42255-024-01064-1. Epub 2024 Jul 1. Nat Metab. 2024. PMID: 38951660 Free PMC article.
-
Obstructive Sleep Apnea-Induced Hypertension Is Associated With Increased Gut and Neuroinflammation.J Am Heart Assoc. 2023 Jun 6;12(11):e029218. doi: 10.1161/JAHA.122.029218. Epub 2023 Jun 1. J Am Heart Assoc. 2023. PMID: 37260032 Free PMC article.
-
Fast cycling of intermittent hypoxia in a physiomimetic 3D environment: A novel tool for the study of the parenchymal effects of sleep apnea.Front Pharmacol. 2023 Jan 12;13:1081345. doi: 10.3389/fphar.2022.1081345. eCollection 2022. Front Pharmacol. 2023. PMID: 36712654 Free PMC article.
-
Experimental Models to Study End-Organ Morbidity in Sleep Apnea: Lessons Learned and Future Directions.Int J Mol Sci. 2022 Nov 20;23(22):14430. doi: 10.3390/ijms232214430. Int J Mol Sci. 2022. PMID: 36430904 Free PMC article. Review.
-
Interactive Effect of Combined Intermittent and Sustained Hypoxia and High-Fat Diet on the Colonic Mucosal Microbiome and Host Gene Expression in Mice.Nat Sci Sleep. 2022 Sep 9;14:1623-1639. doi: 10.2147/NSS.S370957. eCollection 2022. Nat Sci Sleep. 2022. PMID: 36111259 Free PMC article.
References
-
- Tripathi A, Melnik AV, Xue J, Poulsen O, Meehan MJ, Humphrey G, Jiang L, Ackermann G, McDonald D, Zhou D, Knight R, Dorrestein PC, Haddad GG. 2018. Intermittent hypoxia and hypercapnia, a hallmark of obstructive sleep apnea, alters the gut microbiome and metabolome. mSystems 3:e00020-18. doi:10.1128/mSystems.00020-18. - DOI - PMC - PubMed
-
- Douglas RM, Bowden K, Pattison J, Peterson AB, Juliano J, Dalton ND, Gu Y, Alvarez E, Imamura T, Peterson KL, Witztum JL, Haddad GG, Li AC. 2013. Intermittent hypoxia and hypercapnia induce pulmonary artery atherosclerosis and ventricular dysfunction in low density lipoprotein receptor deficient mice. J Appl Physiol 115:1694–1704. doi:10.1152/japplphysiol.00442.2013. - DOI - PMC - PubMed
-
- Xue J, Zhou D, Poulsen O, Imamura T, Hsiao Y-H, Smith TH, Malhotra A, Dorrestein P, Knight R, Haddad GG. 2017. Intermittent hypoxia and hypercapnia accelerate atherosclerosis, partially via trimethylamine-oxide. Am J Respir Cell Mol Biol 57:581–588. doi:10.1165/rcmb.2017-0086OC. - DOI - PMC - PubMed
Grants and funding
LinkOut - more resources
Full Text Sources
Miscellaneous