Enhanced detection of circulating tumor DNA by fragment size analysis
- PMID: 30404863
- PMCID: PMC6483061
- DOI: 10.1126/scitranslmed.aat4921
Enhanced detection of circulating tumor DNA by fragment size analysis
Abstract
Existing methods to improve detection of circulating tumor DNA (ctDNA) have focused on genomic alterations but have rarely considered the biological properties of plasma cell-free DNA (cfDNA). We hypothesized that differences in fragment lengths of circulating DNA could be exploited to enhance sensitivity for detecting the presence of ctDNA and for noninvasive genomic analysis of cancer. We surveyed ctDNA fragment sizes in 344 plasma samples from 200 patients with cancer using low-pass whole-genome sequencing (0.4×). To establish the size distribution of mutant ctDNA, tumor-guided personalized deep sequencing was performed in 19 patients. We detected enrichment of ctDNA in fragment sizes between 90 and 150 bp and developed methods for in vitro and in silico size selection of these fragments. Selecting fragments between 90 and 150 bp improved detection of tumor DNA, with more than twofold median enrichment in >95% of cases and more than fourfold enrichment in >10% of cases. Analysis of size-selected cfDNA identified clinically actionable mutations and copy number alterations that were otherwise not detected. Identification of plasma samples from patients with advanced cancer was improved by predictive models integrating fragment length and copy number analysis of cfDNA, with area under the curve (AUC) >0.99 compared to AUC <0.80 without fragmentation features. Increased identification of cfDNA from patients with glioma, renal, and pancreatic cancer was achieved with AUC > 0.91 compared to AUC < 0.5 without fragmentation features. Fragment size analysis and selective sequencing of specific fragment sizes can boost ctDNA detection and could complement or provide an alternative to deeper sequencing of cfDNA.
Copyright © 2018 The Authors, some rights reserved; exclusive licensee American Association for the Advancement of Science. No claim to original U.S. Government Works.
Conflict of interest statement
Figures
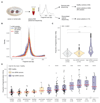
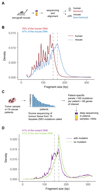
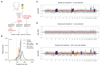
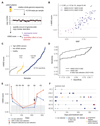
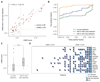
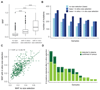
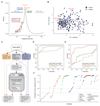
Comment in
-
One size does not fit all: Size-based plasma DNA diagnostics.Sci Transl Med. 2018 Nov 7;10(466):eaav3873. doi: 10.1126/scitranslmed.aav3873. Sci Transl Med. 2018. PMID: 30404860 Review.
Similar articles
-
Refined characterization of circulating tumor DNA through biological feature integration.Sci Rep. 2022 Feb 4;12(1):1928. doi: 10.1038/s41598-022-05606-z. Sci Rep. 2022. PMID: 35121756 Free PMC article.
-
Clinical significance of the mutational landscape and fragmentation of circulating tumor DNA in renal cell carcinoma.Cancer Sci. 2019 Feb;110(2):617-628. doi: 10.1111/cas.13906. Epub 2019 Jan 25. Cancer Sci. 2019. PMID: 30536551 Free PMC article.
-
Diverse fragment lengths dismiss size selection for serum cell-free DNA: a comparative study of serum and plasma samples.Clin Chem Lab Med. 2020 Mar 28;58(9):1451-1459. doi: 10.1515/cclm-2020-0078. Print 2020 Aug 27. Clin Chem Lab Med. 2020. PMID: 32229658
-
Circulating tumor DNA and liquid biopsy: opportunities, challenges, and recent advances in detection technologies.Lab Chip. 2018 Apr 17;18(8):1174-1196. doi: 10.1039/C8LC00100F. Lab Chip. 2018. PMID: 29569666 Review.
-
Using circulating cell-free DNA to monitor personalized cancer therapy.Crit Rev Clin Lab Sci. 2017 May;54(3):205-218. doi: 10.1080/10408363.2017.1299683. Epub 2017 Apr 10. Crit Rev Clin Lab Sci. 2017. PMID: 28393575 Review.
Cited by
-
Cell-Free DNA Fragmentomics: A Promising Biomarker for Diagnosis, Prognosis and Prediction of Response in Breast Cancer.Int J Mol Sci. 2022 Nov 17;23(22):14197. doi: 10.3390/ijms232214197. Int J Mol Sci. 2022. PMID: 36430675 Free PMC article. Review.
-
Liquid biopsy in lymphoma: Molecular methods and clinical applications.Cancer Treat Rev. 2020 Dec;91:102106. doi: 10.1016/j.ctrv.2020.102106. Epub 2020 Sep 22. Cancer Treat Rev. 2020. PMID: 33049623 Free PMC article. Review.
-
Ultradeep targeted sequencing of circulating tumor DNA in plasma of early and advanced breast cancer.Cancer Sci. 2021 Jan;112(1):454-464. doi: 10.1111/cas.14697. Epub 2020 Nov 4. Cancer Sci. 2021. PMID: 33075187 Free PMC article.
-
Longitudinal monitoring of cell-free DNA methylation in ALK-positive non-small cell lung cancer patients.Clin Epigenetics. 2022 Dec 2;14(1):163. doi: 10.1186/s13148-022-01387-4. Clin Epigenetics. 2022. PMID: 36461127 Free PMC article.
-
Liquid biopsies to predict CDK4/6 inhibitor efficacy and resistance in breast cancer.Cancer Drug Resist. 2022 Jun 22;5(3):727-748. doi: 10.20517/cdr.2022.37. eCollection 2022. Cancer Drug Resist. 2022. PMID: 36176758 Free PMC article. Review.
References
-
- Wan JCM, Massie C, Garcia-Corbacho J, Mouliere F, Brenton JD, Caldas C, Pacey S, Baird R, Rosenfeld N. Liquid biopsies come of age: towards implementation of circulating tumour DNA. Nat Rev Cancer. 2017;17:223–238. - PubMed
-
- Murtaza M, Dawson S-J, Tsui DWY, Gale D, Forshew T, Piskorz AM, Parkinson C, Chin S-F, Kingsbury Z, Wong ASC, Marass F, et al. Non-invasive analysis of acquired resistance to cancer therapy by sequencing of plasma DNA. Nature. 2013;497:108–112. - PubMed
-
- Heitzer E, Ulz P, Belic J, Gutschi S, Quehenberger F, Fischereder K, Benezeder T, Auer M, Pischler C, Mannweiler S, Pichler M, et al. Tumor-associated copy number changes in the circulation of patients with prostate cancer identified through whole-genome sequencing. Genome Med. 2013;5:30. - PMC - PubMed
Publication types
MeSH terms
Substances
Grants and funding
LinkOut - more resources
Full Text Sources
Other Literature Sources