P2Rank: machine learning based tool for rapid and accurate prediction of ligand binding sites from protein structure
- PMID: 30109435
- PMCID: PMC6091426
- DOI: 10.1186/s13321-018-0285-8
P2Rank: machine learning based tool for rapid and accurate prediction of ligand binding sites from protein structure
Abstract
Background: Ligand binding site prediction from protein structure has many applications related to elucidation of protein function and structure based drug discovery. It often represents only one step of many in complex computational drug design efforts. Although many methods have been published to date, only few of them are suitable for use in automated pipelines or for processing large datasets. These use cases require stability and speed, which disqualifies many of the recently introduced tools that are either template based or available only as web servers.
Results: We present P2Rank, a stand-alone template-free tool for prediction of ligand binding sites based on machine learning. It is based on prediction of ligandability of local chemical neighbourhoods that are centered on points placed on the solvent accessible surface of a protein. We show that P2Rank outperforms several existing tools, which include two widely used stand-alone tools (Fpocket, SiteHound), a comprehensive consensus based tool (MetaPocket 2.0), and a recent deep learning based method (DeepSite). P2Rank belongs to the fastest available tools (requires under 1 s for prediction on one protein), with additional advantage of multi-threaded implementation.
Conclusions: P2Rank is a new open source software package for ligand binding site prediction from protein structure. It is available as a user-friendly stand-alone command line program and a Java library. P2Rank has a lightweight installation and does not depend on other bioinformatics tools or large structural or sequence databases. Thanks to its speed and ability to make fully automated predictions, it is particularly well suited for processing large datasets or as a component of scalable structural bioinformatics pipelines.
Keywords: Binding site prediction; Ligand binding sites; Machine learning; Protein pockets; Protein surface descriptors; Random forests.
Figures
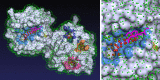
Similar articles
-
PrankWeb: a web server for ligand binding site prediction and visualization.Nucleic Acids Res. 2019 Jul 2;47(W1):W345-W349. doi: 10.1093/nar/gkz424. Nucleic Acids Res. 2019. PMID: 31114880 Free PMC article.
-
Improving protein-ligand binding site prediction accuracy by classification of inner pocket points using local features.J Cheminform. 2015 Apr 1;7:12. doi: 10.1186/s13321-015-0059-5. eCollection 2015. J Cheminform. 2015. PMID: 25932051 Free PMC article.
-
Comparative evaluation of methods for the prediction of protein-ligand binding sites.J Cheminform. 2024 Nov 11;16(1):126. doi: 10.1186/s13321-024-00923-z. J Cheminform. 2024. PMID: 39529176 Free PMC article.
-
Exploring the computational methods for protein-ligand binding site prediction.Comput Struct Biotechnol J. 2020 Feb 17;18:417-426. doi: 10.1016/j.csbj.2020.02.008. eCollection 2020. Comput Struct Biotechnol J. 2020. PMID: 32140203 Free PMC article. Review.
-
Towards more accurate prediction of protein folding rates: a review of the existing Web-based bioinformatics approaches.Brief Bioinform. 2015 Mar;16(2):314-24. doi: 10.1093/bib/bbu007. Epub 2014 Mar 11. Brief Bioinform. 2015. PMID: 24621527 Review.
Cited by
-
MEF-AlloSite: an accurate and robust Multimodel Ensemble Feature selection for the Allosteric Site identification model.J Cheminform. 2024 Oct 23;16(1):116. doi: 10.1186/s13321-024-00882-5. J Cheminform. 2024. PMID: 39444016 Free PMC article.
-
Comprehensive detection and characterization of human druggable pockets through binding site descriptors.Nat Commun. 2024 Sep 10;15(1):7917. doi: 10.1038/s41467-024-52146-3. Nat Commun. 2024. PMID: 39256431 Free PMC article.
-
Novel Noninvasive Serum Biomarkers for Prompt Diagnosis of Breast Carcinoma.ACS Omega. 2023 Dec 21;9(1):1174-1182. doi: 10.1021/acsomega.3c07431. eCollection 2024 Jan 9. ACS Omega. 2023. PMID: 38222620 Free PMC article.
-
Unraveling the Behavior of Intrinsically Disordered Protein c-Myc: A Study Utilizing Gaussian-Accelerated Molecular Dynamics.ACS Omega. 2023 Dec 1;9(2):2250-2262. doi: 10.1021/acsomega.3c05822. eCollection 2024 Jan 16. ACS Omega. 2023. PMID: 38250404 Free PMC article.
-
Exploring Conformational Landscapes and Cryptic Binding Pockets in Distinct Functional States of the SARS-CoV-2 Omicron BA.1 and BA.2 Trimers: Mutation-Induced Modulation of Protein Dynamics and Network-Guided Prediction of Variant-Specific Allosteric Binding Sites.Viruses. 2023 Sep 27;15(10):2009. doi: 10.3390/v15102009. Viruses. 2023. PMID: 37896786 Free PMC article.
References
Grants and funding
LinkOut - more resources
Full Text Sources
Other Literature Sources