Comprehensive Analysis of Alternative Splicing Across Tumors from 8,705 Patients
- PMID: 30078747
- PMCID: PMC9844097
- DOI: 10.1016/j.ccell.2018.07.001
Comprehensive Analysis of Alternative Splicing Across Tumors from 8,705 Patients
Abstract
Our comprehensive analysis of alternative splicing across 32 The Cancer Genome Atlas cancer types from 8,705 patients detects alternative splicing events and tumor variants by reanalyzing RNA and whole-exome sequencing data. Tumors have up to 30% more alternative splicing events than normal samples. Association analysis of somatic variants with alternative splicing events confirmed known trans associations with variants in SF3B1 and U2AF1 and identified additional trans-acting variants (e.g., TADA1, PPP2R1A). Many tumors have thousands of alternative splicing events not detectable in normal samples; on average, we identified ≈930 exon-exon junctions ("neojunctions") in tumors not typically found in GTEx normals. From Clinical Proteomic Tumor Analysis Consortium data available for breast and ovarian tumor samples, we confirmed ≈1.7 neojunction- and ≈0.6 single nucleotide variant-derived peptides per tumor sample that are also predicted major histocompatibility complex-I binders ("putative neoantigens").
Keywords: CPTAC; GTEx; MS proteomics; RNA-seq; TCGA; TCGA Pan-Cancer Atlas; alternative splicing; cancer; exome; immunoediting; immunotherapy; neoantigens; splicing QTL; tumor-specific splicing.
Copyright © 2018 Elsevier Inc. All rights reserved.
Figures
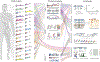
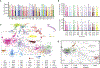
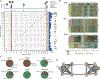
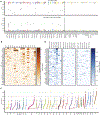
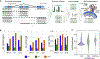
Comment in
-
Cancer-Specific Splicing Changes and the Potential for Splicing-Derived Neoantigens.Cancer Cell. 2018 Aug 13;34(2):181-183. doi: 10.1016/j.ccell.2018.07.008. Cancer Cell. 2018. PMID: 30107172 Free PMC article.
Similar articles
-
Integrative genome-wide analysis of the determinants of RNA splicing in kidney renal clear cell carcinoma.Pac Symp Biocomput. 2015;20:44-55. Pac Symp Biocomput. 2015. PMID: 25592567 Free PMC article.
-
Comprehensive profiling of cancer neoantigens from aberrant RNA splicing.J Immunother Cancer. 2024 May 15;12(5):e008988. doi: 10.1136/jitc-2024-008988. J Immunother Cancer. 2024. PMID: 38754917 Free PMC article.
-
Differentially expressed alternatively spliced genes in malignant pleural mesothelioma identified using massively parallel transcriptome sequencing.BMC Med Genet. 2009 Dec 31;10:149. doi: 10.1186/1471-2350-10-149. BMC Med Genet. 2009. PMID: 20043850 Free PMC article.
-
Understanding human DNA variants affecting pre-mRNA splicing in the NGS era.Adv Genet. 2019;103:39-90. doi: 10.1016/bs.adgen.2018.09.002. Epub 2019 Jan 17. Adv Genet. 2019. PMID: 30904096 Review.
-
Alternative RNA splicing and gastric cancer.Mutat Res Rev Mutat Res. 2017 Jul;773:263-273. doi: 10.1016/j.mrrev.2016.07.011. Epub 2016 Jul 29. Mutat Res Rev Mutat Res. 2017. PMID: 28927534 Review.
Cited by
-
Splicing factor BUD31 promotes ovarian cancer progression through sustaining the expression of anti-apoptotic BCL2L12.Nat Commun. 2022 Oct 21;13(1):6246. doi: 10.1038/s41467-022-34042-w. Nat Commun. 2022. PMID: 36271053 Free PMC article.
-
Comparative RNA-Seq Analysis Revealed Tissue-Specific Splicing Variations during the Generation of the PDX Model.Int J Mol Sci. 2023 Nov 30;24(23):17001. doi: 10.3390/ijms242317001. Int J Mol Sci. 2023. PMID: 38069324 Free PMC article.
-
RPL22 is a tumor suppressor in MSI-high cancers and a key splicing regulator of MDM4.bioRxiv [Preprint]. 2023 Dec 10:2023.12.10.570873. doi: 10.1101/2023.12.10.570873. bioRxiv. 2023. Update in: Cell Rep. 2024 Aug 27;43(8):114622. doi: 10.1016/j.celrep.2024.114622. PMID: 38106152 Free PMC article. Updated. Preprint.
-
Role of epithelial splicing regulatory protein 1 in cancer progression.Cancer Cell Int. 2023 Dec 18;23(1):331. doi: 10.1186/s12935-023-03180-6. Cancer Cell Int. 2023. PMID: 38110955 Free PMC article. Review.
-
Identification of Prognostic Signatures of Alternative Splicing in Glioma.J Mol Neurosci. 2020 Oct;70(10):1484-1492. doi: 10.1007/s12031-020-01581-0. Epub 2020 Jun 29. J Mol Neurosci. 2020. PMID: 32602029
References
-
- Agrawal S, and Eng C (2006). Differential expression of novel naturally occurring splice variants of PTEN and their functional consequences in Cowden syndrome and sporadic breast cancer. Hum. Mol. Genet. 15, 777–787. - PubMed
-
- Bassani-Sternberg M, Bräunlein E, Klar R, Engleitner T, Sinitcyn P, Audehm S, Straub M, Weber J, Slotta-Huspenina J, Specht K, et al. (2016). Direct identification of clinically relevant neoepitopes presented on native human melanoma tissue by mass spectrometry. Nat. Commun. 7, 13404. - PMC - PubMed
Publication types
MeSH terms
Grants and funding
LinkOut - more resources
Full Text Sources
Other Literature Sources
Miscellaneous