Deep reinforcement learning for de novo drug design
- PMID: 30050984
- PMCID: PMC6059760
- DOI: 10.1126/sciadv.aap7885
Deep reinforcement learning for de novo drug design
Abstract
We have devised and implemented a novel computational strategy for de novo design of molecules with desired properties termed ReLeaSE (Reinforcement Learning for Structural Evolution). On the basis of deep and reinforcement learning (RL) approaches, ReLeaSE integrates two deep neural networks-generative and predictive-that are trained separately but are used jointly to generate novel targeted chemical libraries. ReLeaSE uses simple representation of molecules by their simplified molecular-input line-entry system (SMILES) strings only. Generative models are trained with a stack-augmented memory network to produce chemically feasible SMILES strings, and predictive models are derived to forecast the desired properties of the de novo-generated compounds. In the first phase of the method, generative and predictive models are trained separately with a supervised learning algorithm. In the second phase, both models are trained jointly with the RL approach to bias the generation of new chemical structures toward those with the desired physical and/or biological properties. In the proof-of-concept study, we have used the ReLeaSE method to design chemical libraries with a bias toward structural complexity or toward compounds with maximal, minimal, or specific range of physical properties, such as melting point or hydrophobicity, or toward compounds with inhibitory activity against Janus protein kinase 2. The approach proposed herein can find a general use for generating targeted chemical libraries of novel compounds optimized for either a single desired property or multiple properties.
Figures
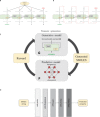
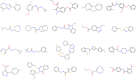
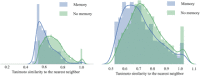
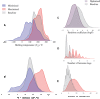
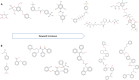
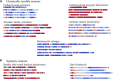
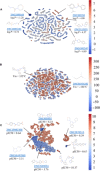
Similar articles
-
Reinforced Adversarial Neural Computer for de Novo Molecular Design.J Chem Inf Model. 2018 Jun 25;58(6):1194-1204. doi: 10.1021/acs.jcim.7b00690. Epub 2018 Jun 12. J Chem Inf Model. 2018. PMID: 29762023
-
De Novo Molecular Design by Combining Deep Autoencoder Recurrent Neural Networks with Generative Topographic Mapping.J Chem Inf Model. 2019 Mar 25;59(3):1182-1196. doi: 10.1021/acs.jcim.8b00751. Epub 2019 Mar 5. J Chem Inf Model. 2019. PMID: 30785751
-
Generative Adversarial Networks for De Novo Molecular Design.Mol Inform. 2021 Oct;40(10):e2100045. doi: 10.1002/minf.202100045. Epub 2021 Jul 6. Mol Inform. 2021. PMID: 34622551
-
Generative Deep Learning for Targeted Compound Design.J Chem Inf Model. 2021 Nov 22;61(11):5343-5361. doi: 10.1021/acs.jcim.0c01496. Epub 2021 Oct 26. J Chem Inf Model. 2021. PMID: 34699719 Review.
-
Deep learning for molecular generation.Future Med Chem. 2019 Mar;11(6):567-597. doi: 10.4155/fmc-2018-0358. Epub 2019 Jan 30. Future Med Chem. 2019. PMID: 30698019 Review.
Cited by
-
Network-principled deep generative models for designing drug combinations as graph sets.Bioinformatics. 2020 Jul 1;36(Suppl_1):i445-i454. doi: 10.1093/bioinformatics/btaa317. Bioinformatics. 2020. PMID: 32657357 Free PMC article.
-
Accelerated antimicrobial discovery via deep generative models and molecular dynamics simulations.Nat Biomed Eng. 2021 Jun;5(6):613-623. doi: 10.1038/s41551-021-00689-x. Epub 2021 Mar 11. Nat Biomed Eng. 2021. PMID: 33707779
-
Predicting chemical structure using reinforcement learning with a stack-augmented conditional variational autoencoder.J Cheminform. 2022 Dec 9;14(1):83. doi: 10.1186/s13321-022-00666-9. J Cheminform. 2022. PMID: 36494855 Free PMC article.
-
Deep learning of pharmacogenomics resources: moving towards precision oncology.Brief Bioinform. 2020 Dec 1;21(6):2066-2083. doi: 10.1093/bib/bbz144. Brief Bioinform. 2020. PMID: 31813953 Free PMC article.
-
Critical assessment of computational tools for prokaryotic and eukaryotic promoter prediction.Brief Bioinform. 2022 Mar 10;23(2):bbab551. doi: 10.1093/bib/bbab551. Brief Bioinform. 2022. PMID: 35021193 Free PMC article.
References
-
- Gil Y., Greaves M., Hendler J., Hirsh H., Amplify scientific discovery with artificial intelligence. Science 346, 171–172 (2014). - PubMed
-
- Krittanawong C., Zhang H., Wang Z., Aydar M., Kitai T., Artificial intelligence in precision cardiovascular medicine. J. Am. Coll. Cardiol. 69, 2657–2664 (2017). - PubMed
-
- Chockley K., Emanuel E., The end of radiology? Three threats to the future practice of radiology. J. Am. Coll. Radiol. 13, 1415–1420 (2016). - PubMed
-
- Gawehn E., Hiss J. A., Schneider G., Deep learning in drug discovery. Mol. Inform. 35, 3–14 (2016). - PubMed
Publication types
MeSH terms
Substances
Grants and funding
LinkOut - more resources
Full Text Sources
Other Literature Sources