Chemistry-First Approach for Nomination of Personalized Treatment in Lung Cancer
- PMID: 29681454
- PMCID: PMC5935540
- DOI: 10.1016/j.cell.2018.03.028
Chemistry-First Approach for Nomination of Personalized Treatment in Lung Cancer
Abstract
Diversity in the genetic lesions that cause cancer is extreme. In consequence, a pressing challenge is the development of drugs that target patient-specific disease mechanisms. To address this challenge, we employed a chemistry-first discovery paradigm for de novo identification of druggable targets linked to robust patient selection hypotheses. In particular, a 200,000 compound diversity-oriented chemical library was profiled across a heavily annotated test-bed of >100 cellular models representative of the diverse and characteristic somatic lesions for lung cancer. This approach led to the delineation of 171 chemical-genetic associations, shedding light on the targetability of mechanistic vulnerabilities corresponding to a range of oncogenotypes present in patient populations lacking effective therapy. Chemically addressable addictions to ciliogenesis in TTC21B mutants and GLUT8-dependent serine biosynthesis in KRAS/KEAP1 double mutants are prominent examples. These observations indicate a wealth of actionable opportunities within the complex molecular etiology of cancer.
Keywords: KRAS mutant; NRF2 signaling; cancer target identification; chemical biology; ciliogenesis; glucocorticoid therapies; lung cancer; serine biosynthesis.
Copyright © 2018 Elsevier Inc. All rights reserved.
Conflict of interest statement
The authors have no conflicts of interest to report related to this work. Michael White is currently an employee of Pfizer Inc. and Takashi Motoyaji was an employee of Takeda Oncology.
Figures
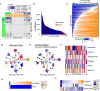
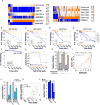
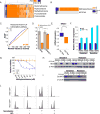
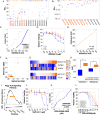
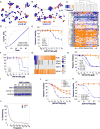
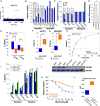
Comment in
-
Chemistry Takes Center Stage for Identifying Cancer Targetability.Cell. 2018 May 3;173(4):815-817. doi: 10.1016/j.cell.2018.04.020. Cell. 2018. PMID: 29727668
-
Identifying therapeutic vulnerabilities in lung cancer: application of a chemistry-first approach.Transl Lung Cancer Res. 2018 Sep;7(Suppl 3):S265-S269. doi: 10.21037/tlcr.2018.09.10. Transl Lung Cancer Res. 2018. PMID: 30393619 Free PMC article. No abstract available.
Similar articles
-
LKB1 and KEAP1/NRF2 Pathways Cooperatively Promote Metabolic Reprogramming with Enhanced Glutamine Dependence in KRAS-Mutant Lung Adenocarcinoma.Cancer Res. 2019 Jul 1;79(13):3251-3267. doi: 10.1158/0008-5472.CAN-18-3527. Epub 2019 Apr 30. Cancer Res. 2019. PMID: 31040157 Free PMC article.
-
ERBB3 and IGF1R Signaling Are Required for Nrf2-Dependent Growth in KEAP1-Mutant Lung Cancer.Cancer Res. 2019 Oct 1;79(19):4828-4839. doi: 10.1158/0008-5472.CAN-18-2086. Epub 2019 Aug 15. Cancer Res. 2019. PMID: 31416841
-
Distinct initiating events underpin the immune and metabolic heterogeneity of KRAS-mutant lung adenocarcinoma.Nat Commun. 2019 Sep 13;10(1):4190. doi: 10.1038/s41467-019-12164-y. Nat Commun. 2019. PMID: 31519898 Free PMC article.
-
Discovery and Development of Kelch-like ECH-Associated Protein 1. Nuclear Factor Erythroid 2-Related Factor 2 (KEAP1:NRF2) Protein-Protein Interaction Inhibitors: Achievements, Challenges, and Future Directions.J Med Chem. 2016 Dec 22;59(24):10837-10858. doi: 10.1021/acs.jmedchem.6b00586. Epub 2016 Oct 12. J Med Chem. 2016. PMID: 27690435 Review.
-
Nuclear factor (erythroid-derived 2)-like 2 (NRF2) drug discovery: Biochemical toolbox to develop NRF2 activators by reversible binding of Kelch-like ECH-associated protein 1 (KEAP1).Arch Biochem Biophys. 2017 Oct 1;631:31-41. doi: 10.1016/j.abb.2017.08.003. Epub 2017 Aug 8. Arch Biochem Biophys. 2017. PMID: 28801166 Review.
Cited by
-
Multigene Panel Sequencing Reveals Cancer-Specific and Common Somatic Mutations in Colorectal Cancer Patients: An Egyptian Experience.Curr Issues Mol Biol. 2022 Mar 18;44(3):1332-1352. doi: 10.3390/cimb44030090. Curr Issues Mol Biol. 2022. PMID: 35723313 Free PMC article.
-
FRA1 contributes to MEK-ERK pathway-dependent PD-L1 upregulation by KRAS mutation in premalignant human bronchial epithelial cells.Am J Transl Res. 2020 Feb 15;12(2):409-427. eCollection 2020. Am J Transl Res. 2020. PMID: 32194893 Free PMC article.
-
Dissecting the Crosstalk between NRF2 Signaling and Metabolic Processes in Cancer.Cancers (Basel). 2020 Oct 17;12(10):3023. doi: 10.3390/cancers12103023. Cancers (Basel). 2020. PMID: 33080927 Free PMC article. Review.
-
Current Status and Perspectives of Patient-Derived Models for Ewing's Sarcoma.Cancers (Basel). 2020 Sep 4;12(9):2520. doi: 10.3390/cancers12092520. Cancers (Basel). 2020. PMID: 32899796 Free PMC article.
-
Microenvironmental regulation of cancer cell metabolism: implications for experimental design and translational studies.Dis Model Mech. 2018 Aug 7;11(8):dmm035758. doi: 10.1242/dmm.035758. Dis Model Mech. 2018. PMID: 30104199 Free PMC article. Review.
References
-
- Baumgart A, Mazur PK, Anton M, Rudelius M, Schwamborn K, Feuchtinger A, Behnke K, Walch A, Braren R, Peschel C, et al. Opposing role of Notch1 and Notch2 in a Kras(G12D)-driven murine non-small cell lung cancer model. Oncogene. 2015;34:578–588. - PubMed
-
- Chu D, Zhang Z, Zhou Y, Wang W, Li Y, Zhang H, Dong G, Zhao Q, Ji G. Notch1 and Notch2 have opposite prognostic effects on patients with colorectal cancer. Ann Oncol. 2011;22:2440–2447. - PubMed
Publication types
MeSH terms
Substances
Grants and funding
LinkOut - more resources
Full Text Sources
Other Literature Sources
Medical
Molecular Biology Databases
Research Materials
Miscellaneous