Extensive impact of non-antibiotic drugs on human gut bacteria
- PMID: 29555994
- PMCID: PMC6108420
- DOI: 10.1038/nature25979
Extensive impact of non-antibiotic drugs on human gut bacteria
Abstract
A few commonly used non-antibiotic drugs have recently been associated with changes in gut microbiome composition, but the extent of this phenomenon is unknown. Here, we screened more than 1,000 marketed drugs against 40 representative gut bacterial strains, and found that 24% of the drugs with human targets, including members of all therapeutic classes, inhibited the growth of at least one strain in vitro. Particular classes, such as the chemically diverse antipsychotics, were overrepresented in this group. The effects of human-targeted drugs on gut bacteria are reflected on their antibiotic-like side effects in humans and are concordant with existing human cohort studies. Susceptibility to antibiotics and human-targeted drugs correlates across bacterial species, suggesting common resistance mechanisms, which we verified for some drugs. The potential risk of non-antibiotics promoting antibiotic resistance warrants further exploration. Our results provide a resource for future research on drug-microbiome interactions, opening new paths for side effect control and drug repurposing, and broadening our view of antibiotic resistance.
Conflict of interest statement
The authors declare no competing financial interests.
Figures
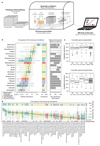
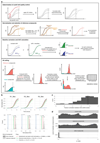
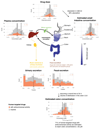
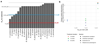
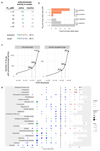
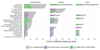
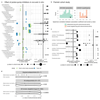
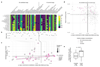
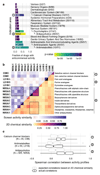
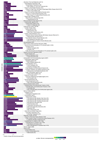
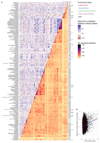
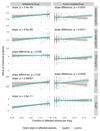
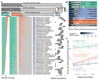
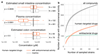
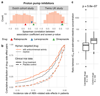
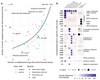
Comment in
-
To grow, or not to grow.Nat Rev Microbiol. 2018 Jun;16(6):329. doi: 10.1038/s41579-018-0006-3. Nat Rev Microbiol. 2018. PMID: 29599456 No abstract available.
-
Broad collateral damage of drugs against the gut microbiome.Nat Rev Gastroenterol Hepatol. 2018 Aug;15(8):457-458. doi: 10.1038/s41575-018-0028-3. Nat Rev Gastroenterol Hepatol. 2018. PMID: 29752457 No abstract available.
-
Cutting the Gordian Knot of the Microbiota.Mol Cell. 2018 Jun 7;70(5):765-767. doi: 10.1016/j.molcel.2018.05.034. Epub 2018 Jun 7. Mol Cell. 2018. PMID: 29883604 Free PMC article.
-
Environmental shaping of the microbiome.Nat Med. 2018 Dec;24(12):1782. doi: 10.1038/s41591-018-0286-1. Nat Med. 2018. PMID: 30523320 No abstract available.
Similar articles
-
Droplet-based high-throughput cultivation for accurate screening of antibiotic resistant gut microbes.Elife. 2020 Jun 17;9:e56998. doi: 10.7554/eLife.56998. Elife. 2020. PMID: 32553109 Free PMC article.
-
Unravelling the collateral damage of antibiotics on gut bacteria.Nature. 2021 Nov;599(7883):120-124. doi: 10.1038/s41586-021-03986-2. Epub 2021 Oct 13. Nature. 2021. PMID: 34646011 Free PMC article.
-
Probiotics impact the antibiotic resistance gene reservoir along the human GI tract in a person-specific and antibiotic-dependent manner.Nat Microbiol. 2021 Aug;6(8):1043-1054. doi: 10.1038/s41564-021-00920-0. Epub 2021 Jul 5. Nat Microbiol. 2021. PMID: 34226711 Free PMC article.
-
Horizontal transfer of antibiotic resistance genes in the human gut microbiome.Curr Opin Microbiol. 2020 Feb;53:35-43. doi: 10.1016/j.mib.2020.02.002. Epub 2020 Mar 3. Curr Opin Microbiol. 2020. PMID: 32143027 Review.
-
Response, resistance, and recovery of gut bacteria to human-targeted drug exposure.Cell Host Microbe. 2024 Jun 12;32(6):786-793. doi: 10.1016/j.chom.2024.05.009. Cell Host Microbe. 2024. PMID: 38870896 Review.
Cited by
-
Role of microbiota and microbiota-derived short-chain fatty acids in PDAC.Cancer Med. 2023 Mar;12(5):5661-5675. doi: 10.1002/cam4.5323. Epub 2022 Oct 7. Cancer Med. 2023. PMID: 36205023 Free PMC article. Review.
-
Differential responses of the gut microbiome and resistome to antibiotic exposures in infants and adults.Nat Commun. 2023 Dec 22;14(1):8526. doi: 10.1038/s41467-023-44289-6. Nat Commun. 2023. PMID: 38135681 Free PMC article.
-
A systematic framework for understanding the microbiome in human health and disease: from basic principles to clinical translation.Signal Transduct Target Ther. 2024 Sep 23;9(1):237. doi: 10.1038/s41392-024-01946-6. Signal Transduct Target Ther. 2024. PMID: 39307902 Free PMC article. Review.
-
Gut microbiota variations in patients diagnosed with major depressive disorder-A systematic review.Brain Behav. 2021 Jul;11(7):e02177. doi: 10.1002/brb3.2177. Epub 2021 May 28. Brain Behav. 2021. PMID: 34047485 Free PMC article. Review.
-
Highly parallel lab evolution reveals that epistasis can curb the evolution of antibiotic resistance.Nat Commun. 2020 Jun 19;11(1):3105. doi: 10.1038/s41467-020-16932-z. Nat Commun. 2020. PMID: 32561723 Free PMC article.
References
Publication types
MeSH terms
Substances
Grants and funding
LinkOut - more resources
Full Text Sources
Other Literature Sources
Medical