Identification of genetic elements in metabolism by high-throughput mouse phenotyping
- PMID: 29348434
- PMCID: PMC5773596
- DOI: 10.1038/s41467-017-01995-2
Identification of genetic elements in metabolism by high-throughput mouse phenotyping
Abstract
Metabolic diseases are a worldwide problem but the underlying genetic factors and their relevance to metabolic disease remain incompletely understood. Genome-wide research is needed to characterize so-far unannotated mammalian metabolic genes. Here, we generate and analyze metabolic phenotypic data of 2016 knockout mouse strains under the aegis of the International Mouse Phenotyping Consortium (IMPC) and find 974 gene knockouts with strong metabolic phenotypes. 429 of those had no previous link to metabolism and 51 genes remain functionally completely unannotated. We compared human orthologues of these uncharacterized genes in five GWAS consortia and indeed 23 candidate genes are associated with metabolic disease. We further identify common regulatory elements in promoters of candidate genes. As each regulatory element is composed of several transcription factor binding sites, our data reveal an extensive metabolic phenotype-associated network of co-regulated genes. Our systematic mouse phenotype analysis thus paves the way for full functional annotation of the genome.
Conflict of interest statement
The authors declare no competing financial interests.
Figures
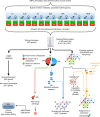
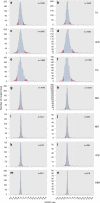
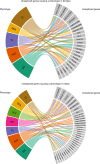
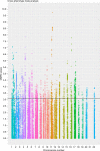
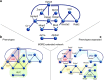
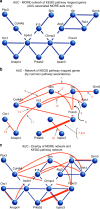
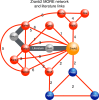
Similar articles
-
Defining the optimum strategy for identifying adults and children with coeliac disease: systematic review and economic modelling.Health Technol Assess. 2022 Oct;26(44):1-310. doi: 10.3310/ZUCE8371. Health Technol Assess. 2022. PMID: 36321689 Free PMC article.
-
Depressing time: Waiting, melancholia, and the psychoanalytic practice of care.In: Kirtsoglou E, Simpson B, editors. The Time of Anthropology: Studies of Contemporary Chronopolitics. Abingdon: Routledge; 2020. Chapter 5. In: Kirtsoglou E, Simpson B, editors. The Time of Anthropology: Studies of Contemporary Chronopolitics. Abingdon: Routledge; 2020. Chapter 5. PMID: 36137063 Free Books & Documents. Review.
-
Hybrid closed-loop systems for managing blood glucose levels in type 1 diabetes: a systematic review and economic modelling.Health Technol Assess. 2024 Dec;28(80):1-190. doi: 10.3310/JYPL3536. Health Technol Assess. 2024. PMID: 39673446 Free PMC article. Review.
-
Discarded sequencing reads uncover natural variation in pest resistance in Thlaspi arvense.Elife. 2024 Dec 19;13:RP95510. doi: 10.7554/eLife.95510. Elife. 2024. PMID: 39699583 Free PMC article.
-
The effectiveness of abstinence-based and harm reduction-based interventions in reducing problematic substance use in adults who are experiencing homelessness in high income countries: A systematic review and meta-analysis: A systematic review.Campbell Syst Rev. 2024 Apr 21;20(2):e1396. doi: 10.1002/cl2.1396. eCollection 2024 Jun. Campbell Syst Rev. 2024. PMID: 38645303 Free PMC article. Review.
Cited by
-
Metabolic effects of the schizophrenia-associated 3q29 deletion.Transl Psychiatry. 2022 Feb 17;12(1):66. doi: 10.1038/s41398-022-01824-1. Transl Psychiatry. 2022. PMID: 35177588 Free PMC article.
-
The role of genetic variation of human metabolism for BMI, mental traits and mental disorders.Mol Metab. 2018 Jun;12:1-11. doi: 10.1016/j.molmet.2018.03.015. Epub 2018 Apr 3. Mol Metab. 2018. PMID: 29673576 Free PMC article.
-
Insights into energy balance dysregulation from a mouse model of methylmalonic aciduria.Hum Mol Genet. 2023 Aug 26;32(17):2717-2734. doi: 10.1093/hmg/ddad100. Hum Mol Genet. 2023. PMID: 37369025 Free PMC article.
-
Advances in mouse genetics for the study of human disease.Hum Mol Genet. 2021 Oct 1;30(R2):R274-R284. doi: 10.1093/hmg/ddab153. Hum Mol Genet. 2021. PMID: 34089057 Free PMC article. Review.
-
The International Mouse Phenotyping Consortium: comprehensive knockout phenotyping underpinning the study of human disease.Nucleic Acids Res. 2023 Jan 6;51(D1):D1038-D1045. doi: 10.1093/nar/gkac972. Nucleic Acids Res. 2023. PMID: 36305825 Free PMC article.
References
-
- Boehme MW, et al. Prevalence, incidence and concomitant co-morbidities of type 2 diabetes mellitus in South Western Germany–a retrospective cohort and case control study in claims data of a large statutory health insurance. BMC Public Health. 2015;15:855. doi: 10.1186/s12889-015-2188-1. - DOI - PMC - PubMed
Publication types
MeSH terms
Substances
Grants and funding
- U54 HG006332/HG/NHGRI NIH HHS/United States
- UM1 OD023221/OD/NIH HHS/United States
- MC_U142684171/MRC_/Medical Research Council/United Kingdom
- R24 OD011883/OD/NIH HHS/United States
- U42 OD012210/OD/NIH HHS/United States
- UM1 HG006370/HG/NHGRI NIH HHS/United States
- U54 HG006348/HG/NHGRI NIH HHS/United States
- U54 HG006370/HG/NHGRI NIH HHS/United States
- MC_UP_1502/3/MRC_/Medical Research Council/United Kingdom
- G0300212/MRC_/Medical Research Council/United Kingdom
- K08 EY027463/EY/NEI NIH HHS/United States
- U42 RR024244/RR/NCRR NIH HHS/United States
- U24 DK092993/DK/NIDDK NIH HHS/United States
- MC_QA137918/MRC_/Medical Research Council/United Kingdom
- U42 RR033193/RR/NCRR NIH HHS/United States
- UM1 OD023222/OD/NIH HHS/United States
- MR/N012119/1/MRC_/Medical Research Council/United Kingdom
- U54 HG006364/HG/NHGRI NIH HHS/United States
- MC_U142684172/MRC_/Medical Research Council/United Kingdom
- UM1 HG006348/HG/NHGRI NIH HHS/United States
- U42 OD011174/OD/NIH HHS/United States
- U42 OD011175/OD/NIH HHS/United States
- WT_/Wellcome Trust/United Kingdom
- U42 OD011185/OD/NIH HHS/United States
- U2C DK092993/DK/NIDDK NIH HHS/United States
LinkOut - more resources
Full Text Sources
Other Literature Sources
Medical
Molecular Biology Databases
Research Materials