TreeTime: Maximum-likelihood phylodynamic analysis
- PMID: 29340210
- PMCID: PMC5758920
- DOI: 10.1093/ve/vex042
TreeTime: Maximum-likelihood phylodynamic analysis
Abstract
Mutations that accumulate in the genome of cells or viruses can be used to infer their evolutionary history. In the case of rapidly evolving organisms, genomes can reveal their detailed spatiotemporal spread. Such phylodynamic analyses are particularly useful to understand the epidemiology of rapidly evolving viral pathogens. As the number of genome sequences available for different pathogens has increased dramatically over the last years, phylodynamic analysis with traditional methods becomes challenging as these methods scale poorly with growing datasets. Here, we present TreeTime, a Python-based framework for phylodynamic analysis using an approximate Maximum Likelihood approach. TreeTime can estimate ancestral states, infer evolution models, reroot trees to maximize temporal signals, estimate molecular clock phylogenies and population size histories. The runtime of TreeTime scales linearly with dataset size.
Keywords: molecular clock phylogenies; phylodynamics; python.
Figures
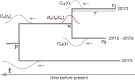
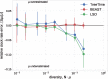
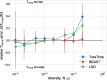
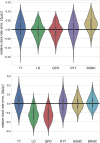
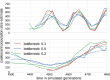
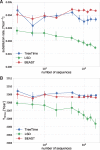
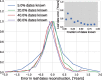
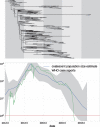
Similar articles
-
Applications of Bayesian Phylodynamic Methods in a Recent U.S. Porcine Reproductive and Respiratory Syndrome Virus Outbreak.Front Microbiol. 2016 Feb 2;7:67. doi: 10.3389/fmicb.2016.00067. eCollection 2016. Front Microbiol. 2016. PMID: 26870024 Free PMC article.
-
Phylodynamic applications in 21st century global infectious disease research.Glob Health Res Policy. 2017 May 8;2:13. doi: 10.1186/s41256-017-0034-y. eCollection 2017. Glob Health Res Policy. 2017. PMID: 29202081 Free PMC article.
-
TreeTime: an extensible C++ software package for Bayesian phylogeny reconstruction with time-calibration.Bioinformatics. 2009 Sep 15;25(18):2440-1. doi: 10.1093/bioinformatics/btp417. Epub 2009 Jul 3. Bioinformatics. 2009. PMID: 19578040
-
Towards a new paradigm linking virus molecular evolution and pathogenesis: experimental design and phylodynamic inference.New Microbiol. 2012 Apr;35(2):101-11. Epub 2012 Mar 31. New Microbiol. 2012. PMID: 22707126 Review.
-
Advances in Visualization Tools for Phylogenomic and Phylodynamic Studies of Viral Diseases.Front Public Health. 2019 Aug 2;7:208. doi: 10.3389/fpubh.2019.00208. eCollection 2019. Front Public Health. 2019. PMID: 31428595 Free PMC article. Review.
Cited by
-
Molecular epidemiology of mumps viruses in the Netherlands, 2017-2019.PLoS One. 2020 Sep 14;15(9):e0233143. doi: 10.1371/journal.pone.0233143. eCollection 2020. PLoS One. 2020. PMID: 32925979 Free PMC article.
-
Integrating genotypes and phenotypes improves long-term forecasts of seasonal influenza A/H3N2 evolution.Elife. 2020 Sep 2;9:e60067. doi: 10.7554/eLife.60067. Elife. 2020. PMID: 32876050 Free PMC article.
-
Population genomics provides insights into the evolution and adaptation to humans of the waterborne pathogen Mycobacterium kansasii.Nat Commun. 2021 May 3;12(1):2491. doi: 10.1038/s41467-021-22760-6. Nat Commun. 2021. PMID: 33941780 Free PMC article.
-
Longitudinal immune profiling of a SARS-CoV-2 reinfection in a solid organ transplant recipient.Res Sq [Preprint]. 2021 May 5:rs.3.rs-405958. doi: 10.21203/rs.3.rs-405958/v1. Res Sq. 2021. Update in: J Infect Dis. 2022 Feb 1;225(3):374-384. doi: 10.1093/infdis/jiab553 PMID: 34013255 Free PMC article. Updated. Preprint.
-
Incipient functional SARS-CoV-2 diversification identified through neural network haplotype maps.Proc Natl Acad Sci U S A. 2024 Mar 5;121(10):e2317851121. doi: 10.1073/pnas.2317851121. Epub 2024 Feb 28. Proc Natl Acad Sci U S A. 2024. PMID: 38416684 Free PMC article.
References
-
- Aris-Brosou S., Yang Z., Huelsenbeck J. (2002) ‘Effects of Models of Rate Evolution on Estimation of Divergence Dates With Special Reference to the Metazoan 18s Ribosomal RNA Phylogeny, Systematic Biology, 51: 703. - PubMed
-
- Britton T., Anderson C. L., Jacquet D.. et al. (2007) ‘Estimating Divergence Times in Large Phylogenetic Trees’, Systematic Biology, 56: 741. - PubMed
LinkOut - more resources
Full Text Sources
Other Literature Sources
Research Materials