A combination of circulating miRNAs for the early detection of ovarian cancer
- PMID: 29163790
- PMCID: PMC5685711
- DOI: 10.18632/oncotarget.20688
A combination of circulating miRNAs for the early detection of ovarian cancer
Abstract
Ovarian cancer is the leading cause of gynecologic cancer mortality, due to the difficulty of early detection. Current screening methods lack sufficient accuracy, and it is still challenging to propose a new early detection method that improves patient outcomes with less-invasiveness. Although many studies have suggested the utility of circulating microRNAs in cancer detection, their potential for early detection remains elusive. Here, we develop novel predictive models using a combination of 8 circulating serum miRNAs. This method was able to successfully distinguish ovarian cancer patients from healthy controls (area under the curve, 0.97; sensitivity, 0.92; and specificity, 0.91) and early-stage ovarian cancer from patients with benign tumors (0.91, 0.86 and 0.83, respectively). This method also enables subtype classification in 4 types of epithelial ovarian cancer. Furthermore, it is found that most of the 8 miRNAs were packaged in extracellular vesicles, including exosomes, derived from ovarian cancer cells, and they were circulating in murine blood stream. The circulating miRNAs described in this study may serve as biomarkers for ovarian cancer patients. Early detection and subtype determination prior to surgery are crucial for clinicians to design an effective treatment strategy for each patient, as is the goal of precision medicine.
Keywords: biomarkers; circulating microRNAs; exosomes; liquid biopsy; ovarian cancer.
Conflict of interest statement
CONFLICTS OF INTEREST The authors have declared that no conflicts of interest exists.
Figures
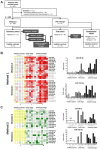
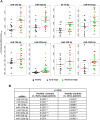
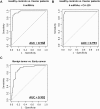
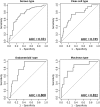
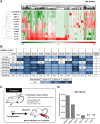
Similar articles
-
Clinical relevance of circulating cell-free microRNAs in ovarian cancer.Mol Cancer. 2016 Jun 24;15(1):48. doi: 10.1186/s12943-016-0536-0. Mol Cancer. 2016. PMID: 27343009 Free PMC article. Review.
-
Liquid biopsy in ovarian cancer: the potential of circulating miRNAs and exosomes.Transl Res. 2019 Mar;205:77-91. doi: 10.1016/j.trsl.2018.10.003. Epub 2018 Oct 12. Transl Res. 2019. PMID: 30391474 Review.
-
Exosomal miR-99a-5p is elevated in sera of ovarian cancer patients and promotes cancer cell invasion by increasing fibronectin and vitronectin expression in neighboring peritoneal mesothelial cells.BMC Cancer. 2018 Nov 5;18(1):1065. doi: 10.1186/s12885-018-4974-5. BMC Cancer. 2018. PMID: 30396333 Free PMC article.
-
Circulating Exosomal miRNAs as Biomarkers in Epithelial Ovarian Cancer.Biomedicines. 2021 Oct 10;9(10):1433. doi: 10.3390/biomedicines9101433. Biomedicines. 2021. PMID: 34680550 Free PMC article. Review.
-
The clinical impact of intra- and extracellular miRNAs in ovarian cancer.Cancer Sci. 2020 Oct;111(10):3435-3444. doi: 10.1111/cas.14599. Epub 2020 Aug 27. Cancer Sci. 2020. PMID: 32750177 Free PMC article. Review.
Cited by
-
High-Grade Serous Ovarian Cancer-A Risk Factor Puzzle and Screening Fugitive.Biomedicines. 2024 Jan 19;12(1):229. doi: 10.3390/biomedicines12010229. Biomedicines. 2024. PMID: 38275400 Free PMC article. Review.
-
Frontline Management of Epithelial Ovarian Cancer-Combining Clinical Expertise with Community Practice Collaboration and Cutting-Edge Research.J Clin Med. 2020 Sep 1;9(9):2830. doi: 10.3390/jcm9092830. J Clin Med. 2020. PMID: 32882942 Free PMC article. Review.
-
Circulating miRNA panels for specific and early detection in bladder cancer.Cancer Sci. 2019 Jan;110(1):408-419. doi: 10.1111/cas.13856. Epub 2018 Dec 12. Cancer Sci. 2019. PMID: 30382619 Free PMC article.
-
Personalized medicine: From diagnostic to adaptive.Biomed J. 2022 Feb;45(1):132-142. doi: 10.1016/j.bj.2019.05.004. Epub 2019 Jul 9. Biomed J. 2022. PMID: 35590431 Free PMC article. Review.
-
miR-150-5p represses TP53 tumor suppressor gene to promote proliferation of colon adenocarcinoma.Sci Rep. 2019 May 1;9(1):6740. doi: 10.1038/s41598-019-43231-5. Sci Rep. 2019. PMID: 31043658 Free PMC article.
References
-
- Webb PM, Jordan SJ. Epidemiology of epithelial ovarian cancer. Best Pract Res Clin Obstet Gynaecol. 2016 https://doi.org/10.1016/j.bpobgyn.2016.08.006. - DOI - PubMed
-
- Huttenhain R, Soste M, Selevsek N, Rost H, Sethi A, Carapito C, Farrah T, Deutsch EW, Kusebauch U, Moritz RL, Nimeus-Malmstrom E, Rinner O, Aebersold R. Reproducible quantification of cancer-associated proteins in body fluids using targeted proteomics. Sci Transl Med. 2012;4:142ra94. https://doi.org/10.1126/scitranslmed.3003989. - DOI - PMC - PubMed
-
- Nolen BM, Lokshin AE. Protein biomarkers of ovarian cancer: the forest and the trees. Future Oncol. 2012;8:55–71. https://doi.org/10.2217/fon.11.135. - DOI - PMC - PubMed
-
- Hausler SF, Keller A, Chandran PA, Ziegler K, Zipp K, Heuer S, Krockenberger M, Engel JB, Honig A, Scheffler M, Dietl J, Wischhusen J. Whole blood-derived miRNA profiles as potential new tools for ovarian cancer screening. Br J Cancer. 2010;103:693–700. https://doi.org/10.1038/sj.bjc.6605833. - DOI - PMC - PubMed
-
- Menon U, Skates SJ, Lewis S, Rosenthal AN, Rufford B, Sibley K, Macdonald N, Dawnay A, Jeyarajah A, Bast RC, Jr, Oram D, Jacobs IJ. Prospective study using the risk of ovarian cancer algorithm to screen for ovarian cancer. J Clin Oncol. 2005;23:7919–26. https://doi.org/10.1200/JCO.2005.01.6642. - DOI - PubMed
LinkOut - more resources
Full Text Sources
Other Literature Sources