Deep Learning-Based Multi-Omics Integration Robustly Predicts Survival in Liver Cancer
- PMID: 28982688
- PMCID: PMC6050171
- DOI: 10.1158/1078-0432.CCR-17-0853
Deep Learning-Based Multi-Omics Integration Robustly Predicts Survival in Liver Cancer
Abstract
Identifying robust survival subgroups of hepatocellular carcinoma (HCC) will significantly improve patient care. Currently, endeavor of integrating multi-omics data to explicitly predict HCC survival from multiple patient cohorts is lacking. To fill this gap, we present a deep learning (DL)-based model on HCC that robustly differentiates survival subpopulations of patients in six cohorts. We built the DL-based, survival-sensitive model on 360 HCC patients' data using RNA sequencing (RNA-Seq), miRNA sequencing (miRNA-Seq), and methylation data from The Cancer Genome Atlas (TCGA), which predicts prognosis as good as an alternative model where genomics and clinical data are both considered. This DL-based model provides two optimal subgroups of patients with significant survival differences (P = 7.13e-6) and good model fitness [concordance index (C-index) = 0.68]. More aggressive subtype is associated with frequent TP53 inactivation mutations, higher expression of stemness markers (KRT19 and EPCAM) and tumor marker BIRC5, and activated Wnt and Akt signaling pathways. We validated this multi-omics model on five external datasets of various omics types: LIRI-JP cohort (n = 230, C-index = 0.75), NCI cohort (n = 221, C-index = 0.67), Chinese cohort (n = 166, C-index = 0.69), E-TABM-36 cohort (n = 40, C-index = 0.77), and Hawaiian cohort (n = 27, C-index = 0.82). This is the first study to employ DL to identify multi-omics features linked to the differential survival of patients with HCC. Given its robustness over multiple cohorts, we expect this workflow to be useful at predicting HCC prognosis prediction. Clin Cancer Res; 24(6); 1248-59. ©2017 AACR.
©2017 American Association for Cancer Research.
Conflict of interest statement
The authors declared no conflict of interest.
Figures
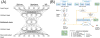
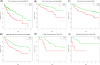
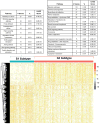
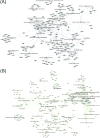
Similar articles
-
Bidirectional deep neural networks to integrate RNA and DNA data for predicting outcome for patients with hepatocellular carcinoma.Future Oncol. 2021 Nov;17(33):4481-4495. doi: 10.2217/fon-2021-0659. Epub 2021 Aug 10. Future Oncol. 2021. PMID: 34374301
-
Deep learning-based model for predicting progression in patients with head and neck squamous cell carcinoma.Cancer Biomark. 2020;27(1):19-28. doi: 10.3233/CBM-190380. Cancer Biomark. 2020. PMID: 31658045
-
Novel deep learning-based solution for identification of prognostic subgroups in liver cancer (Hepatocellular carcinoma).BMC Bioinformatics. 2021 Nov 24;22(1):563. doi: 10.1186/s12859-021-04454-4. BMC Bioinformatics. 2021. PMID: 34819028 Free PMC article.
-
Multiple "Omics" data-based biomarker screening for hepatocellular carcinoma diagnosis.World J Gastroenterol. 2019 Aug 14;25(30):4199-4212. doi: 10.3748/wjg.v25.i30.4199. World J Gastroenterol. 2019. PMID: 31435173 Free PMC article. Review.
-
High-dimensional biology to comprehend hepatocellular carcinoma.Expert Rev Proteomics. 2008 Feb;5(1):45-60. doi: 10.1586/14789450.5.1.45. Expert Rev Proteomics. 2008. PMID: 18282123 Review.
Cited by
-
Comprehensive review and updated analysis of DNA methylation in hepatocellular carcinoma: From basic research to clinical application.Clin Transl Med. 2024 Nov;14(11):e70066. doi: 10.1002/ctm2.70066. Clin Transl Med. 2024. PMID: 39462685 Free PMC article. Review.
-
Enhancing Non-Small Cell Lung Cancer Survival Prediction through Multi-Omics Integration Using Graph Attention Network.Diagnostics (Basel). 2024 Sep 29;14(19):2178. doi: 10.3390/diagnostics14192178. Diagnostics (Basel). 2024. PMID: 39410583 Free PMC article.
-
Improving Stroke Outcome Prediction Using Molecular and Machine Learning Approaches in Large Vessel Occlusion.J Clin Med. 2024 Oct 3;13(19):5917. doi: 10.3390/jcm13195917. J Clin Med. 2024. PMID: 39407977 Free PMC article.
-
Integrated analysis of single-cell RNA-seq and bulk RNA-seq revealed key genes for bone metastasis and chemoresistance in prostate cancer.Genes Genomics. 2024 Oct 12. doi: 10.1007/s13258-024-01575-x. Online ahead of print. Genes Genomics. 2024. PMID: 39395905
-
Deep learning-based approaches for multi-omics data integration and analysis.BioData Min. 2024 Oct 2;17(1):38. doi: 10.1186/s13040-024-00391-z. BioData Min. 2024. PMID: 39358793 Free PMC article. Review.
References
-
- Torre LA, Bray F, Siegel RL, Ferlay J, Lortet-Tieulent J, Jemal A. Global cancer statistics, 2012. CA Cancer J Clin. 2015;65(2):87–108. - PubMed
-
- Society AC. [Accessed 2017 May 24];Key Statistics About Liver Cancer. 2017 May 24; < https://www.cancer.org/cancer/liver-cancer/about/what-is-key-statistics....>.
-
- Siegel RL, Miller KD, Jemal A. Cancer statistics, 2016. CA Cancer J Clin. 2016;66(1):7–30. - PubMed
-
- Trevisani F, Magini G, Santi V, Morselli-Labate AM, Cantarini MC, Di Nolfo MA, et al. Impact of etiology of cirrhosis on the survival of patients diagnosed with hepatocellular carcinoma during surveillance. Am J Gastroenterol. 2007;102(5):1022–31. - PubMed
-
- Nguyen VT, Law MG, Dore GJ. Hepatitis B-related hepatocellular carcinoma: epidemiological characteristics and disease burden. J Viral Hepat. 2009;16(7):453–63. - PubMed
Publication types
MeSH terms
Substances
Grants and funding
LinkOut - more resources
Full Text Sources
Other Literature Sources
Medical
Research Materials
Miscellaneous