Finding the needle in the haystack: towards solving the protein-folding problem computationally
- PMID: 28976219
- PMCID: PMC6790072
- DOI: 10.1080/10409238.2017.1380596
Finding the needle in the haystack: towards solving the protein-folding problem computationally
Abstract
Prediction of protein tertiary structures from amino acid sequence and understanding the mechanisms of how proteins fold, collectively known as "the protein folding problem," has been a grand challenge in molecular biology for over half a century. Theories have been developed that provide us with an unprecedented understanding of protein folding mechanisms. However, computational simulation of protein folding is still difficult, and prediction of protein tertiary structure from amino acid sequence is an unsolved problem. Progress toward a satisfying solution has been slow due to challenges in sampling the vast conformational space and deriving sufficiently accurate energy functions. Nevertheless, several techniques and algorithms have been adopted to overcome these challenges, and the last two decades have seen exciting advances in enhanced sampling algorithms, computational power and tertiary structure prediction methodologies. This review aims at summarizing these computational techniques, specifically conformational sampling algorithms and energy approximations that have been frequently used to study protein-folding mechanisms or to de novo predict protein tertiary structures. We hope that this review can serve as an overview on how the protein-folding problem can be studied computationally and, in cases where experimental approaches are prohibitive, help the researcher choose the most relevant computational approach for the problem at hand. We conclude with a summary of current challenges faced and an outlook on potential future directions.
Keywords: Protein-folding problem; conformational sampling algorithms; protein energy approximations; protein structure prediction; protein-folding simulation; sparse experimental data.
Conflict of interest statement
Disclosure statement
The authors report no conflicts of interest. The authors alone are responsible for the content and writing of this article.
Figures
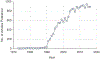
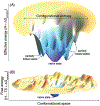
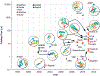
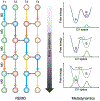
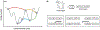
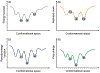
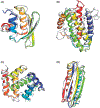
Similar articles
-
Recent Advances in Protein Folding Pathway Prediction through Computational Methods.Curr Med Chem. 2024;31(26):4111-4126. doi: 10.2174/0109298673265249231004193520. Curr Med Chem. 2024. PMID: 37828669 Review.
-
De novo protein structure prediction using ultra-fast molecular dynamics simulation.PLoS One. 2018 Nov 20;13(11):e0205819. doi: 10.1371/journal.pone.0205819. eCollection 2018. PLoS One. 2018. PMID: 30458007 Free PMC article.
-
De novo and inverse folding predictions of protein structure and dynamics.J Comput Aided Mol Des. 1993 Aug;7(4):397-438. doi: 10.1007/BF02337559. J Comput Aided Mol Des. 1993. PMID: 8229093 Review.
-
Toward high-resolution de novo structure prediction for small proteins.Science. 2005 Sep 16;309(5742):1868-71. doi: 10.1126/science.1113801. Science. 2005. PMID: 16166519
-
Principles, challenges and advances in ab initio protein structure prediction.Protein Pept Lett. 2012 Nov;19(11):1194-204. doi: 10.2174/092986612803217015. Protein Pept Lett. 2012. PMID: 22587787
Cited by
-
Comparison of Peptide Ion Conformers Arising from Non-Helical and Helical Peptides Using Ion Mobility Spectrometry and Gas-Phase Hydrogen/Deuterium Exchange.J Am Soc Mass Spectrom. 2018 Dec;29(12):2402-2412. doi: 10.1007/s13361-018-2053-4. Epub 2018 Oct 15. J Am Soc Mass Spectrom. 2018. PMID: 30324261 Free PMC article.
-
Predicting changes in protein thermodynamic stability upon point mutation with deep 3D convolutional neural networks.PLoS Comput Biol. 2020 Nov 30;16(11):e1008291. doi: 10.1371/journal.pcbi.1008291. eCollection 2020 Nov. PLoS Comput Biol. 2020. PMID: 33253214 Free PMC article.
-
Biology and medicine in the landscape of quantum advantages.J R Soc Interface. 2022 Nov;19(196):20220541. doi: 10.1098/rsif.2022.0541. Epub 2022 Nov 30. J R Soc Interface. 2022. PMID: 36448288 Free PMC article. Review.
-
Protein folding in vitro and in the cell: From a solitary journey to a team effort.Biophys Chem. 2022 Aug;287:106821. doi: 10.1016/j.bpc.2022.106821. Epub 2022 Apr 29. Biophys Chem. 2022. PMID: 35667131 Free PMC article. Review.
-
Interfaces Between Alpha-helical Integral Membrane Proteins: Characterization, Prediction, and Docking.Comput Struct Biotechnol J. 2019 May 25;17:699-711. doi: 10.1016/j.csbj.2019.05.005. eCollection 2019. Comput Struct Biotechnol J. 2019. PMID: 31303974 Free PMC article.
References
-
- Abeysinghe S, Ju T, Baker ML, Chiu W. 2008. Shape modeling and matching in identifying 3D protein structures. Computer-Aided Design. 40:708–720.
-
- Amir ED, Kalisman N, Keasar C. 2008. Differentiable, multidimensional, knowledge-based energy terms for torsion angle probabilities and propensities. Proteins. 72:62–73. - PubMed
-
- Anfinsen CB. 1973. Principles that govern the folding of protein chains. Science. 181:223–230. - PubMed
Publication types
MeSH terms
Substances
Grants and funding
LinkOut - more resources
Full Text Sources
Other Literature Sources