Comprehensive Classification of Retinal Bipolar Neurons by Single-Cell Transcriptomics
- PMID: 27565351
- PMCID: PMC5003425
- DOI: 10.1016/j.cell.2016.07.054
Comprehensive Classification of Retinal Bipolar Neurons by Single-Cell Transcriptomics
Abstract
Patterns of gene expression can be used to characterize and classify neuronal types. It is challenging, however, to generate taxonomies that fulfill the essential criteria of being comprehensive, harmonizing with conventional classification schemes, and lacking superfluous subdivisions of genuine types. To address these challenges, we used massively parallel single-cell RNA profiling and optimized computational methods on a heterogeneous class of neurons, mouse retinal bipolar cells (BCs). From a population of ∼25,000 BCs, we derived a molecular classification that identified 15 types, including all types observed previously and two novel types, one of which has a non-canonical morphology and position. We validated the classification scheme and identified dozens of novel markers using methods that match molecular expression to cell morphology. This work provides a systematic methodology for achieving comprehensive molecular classification of neurons, identifies novel neuronal types, and uncovers transcriptional differences that distinguish types within a class.
Copyright © 2016 Elsevier Inc. All rights reserved.
Figures
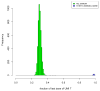
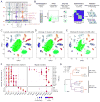
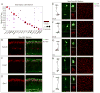
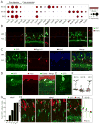
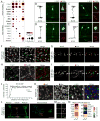
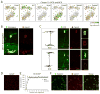
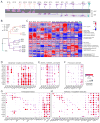
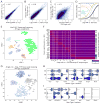
Similar articles
-
Expression of the LIM-homeodomain protein Isl1 in the developing and mature mouse retina.J Comp Neurol. 2007 Jul 1;503(1):182-97. doi: 10.1002/cne.21390. J Comp Neurol. 2007. PMID: 17480014 Free PMC article.
-
Expression of mRNA for glutamate receptor subunits distinguishes the major classes of retinal neurons, but is less specific for individual cell types.Mol Vis. 2007 Jun 18;13:933-48. Mol Vis. 2007. PMID: 17653033 Free PMC article.
-
The final fates of neurogenin2-expressing cells include all major neuron types in the mouse retina.Mol Cell Neurosci. 2006 Mar;31(3):463-9. doi: 10.1016/j.mcn.2005.10.018. Epub 2005 Dec 20. Mol Cell Neurosci. 2006. PMID: 16364654 Free PMC article.
-
Transcriptomics in the RNA-seq era.Curr Opin Chem Biol. 2013 Feb;17(1):4-11. doi: 10.1016/j.cbpa.2012.12.008. Epub 2013 Jan 2. Curr Opin Chem Biol. 2013. PMID: 23290152 Review.
-
Amacrine cell-mediated input to bipolar cells: variations on a common mechanistic theme.Vis Neurosci. 2012 Jan;29(1):41-9. doi: 10.1017/S0952523811000241. Vis Neurosci. 2012. PMID: 22310371 Review.
Cited by
-
Increased Connexin36 Phosphorylation in AII Amacrine Cell Coupling of the Mouse Myopic Retina.Front Cell Neurosci. 2020 Jun 1;14:124. doi: 10.3389/fncel.2020.00124. eCollection 2020. Front Cell Neurosci. 2020. PMID: 32547367 Free PMC article.
-
Evolutionary conservation of VSX2 super-enhancer modules in retinal development.Development. 2024 Jul 1;151(13):dev202435. doi: 10.1242/dev.202435. Epub 2024 Jul 12. Development. 2024. PMID: 38994775 Free PMC article.
-
Pinpointing Cell Identity in Time and Space.Front Mol Biosci. 2020 Aug 14;7:209. doi: 10.3389/fmolb.2020.00209. eCollection 2020. Front Mol Biosci. 2020. PMID: 32923457 Free PMC article.
-
Comprehensive single-cell atlas of the mouse retina.bioRxiv [Preprint]. 2024 Jan 28:2024.01.24.577060. doi: 10.1101/2024.01.24.577060. bioRxiv. 2024. Update in: iScience. 2024 May 08;27(6):109916. doi: 10.1016/j.isci.2024.109916 PMID: 38328114 Free PMC article. Updated. Preprint.
-
Center-surround interactions underlie bipolar cell motion sensitivity in the mouse retina.Nat Commun. 2022 Sep 26;13(1):5574. doi: 10.1038/s41467-022-32762-7. Nat Commun. 2022. PMID: 36163124 Free PMC article.
References
-
- Baas D, Bumsted KM, Martinez JA, Vaccarino FM, Wikler KC, Barnstable CJ. The subcellular localization of Otx2 is cell-type specific and developmentally regulated in the mouse retina. Brain Res Mol Brain Res. 2000;78:26–37. - PubMed
-
- Blondel VD, Guillaume JL, Lambiotte R, Lefebvre E. Fast unfolding of communities in large networks. J Stat Mech-Theory E 2008
-
- Breiman L. Random forests. Mach Learn. 2001;45:5–32.
Publication types
MeSH terms
Substances
Grants and funding
LinkOut - more resources
Full Text Sources
Other Literature Sources
Molecular Biology Databases
Research Materials
Miscellaneous