OncodriveFML: a general framework to identify coding and non-coding regions with cancer driver mutations
- PMID: 27311963
- PMCID: PMC4910259
- DOI: 10.1186/s13059-016-0994-0
OncodriveFML: a general framework to identify coding and non-coding regions with cancer driver mutations
Abstract
Distinguishing the driver mutations from somatic mutations in a tumor genome is one of the major challenges of cancer research. This challenge is more acute and far from solved for non-coding mutations. Here we present OncodriveFML, a method designed to analyze the pattern of somatic mutations across tumors in both coding and non-coding genomic regions to identify signals of positive selection, and therefore, their involvement in tumorigenesis. We describe the method and illustrate its usefulness to identify protein-coding genes, promoters, untranslated regions, intronic splice regions, and lncRNAs-containing driver mutations in several malignancies.
Keywords: Cancer drivers; Local functional mutations bias; Non-coding drivers; Non-coding regions.
Figures
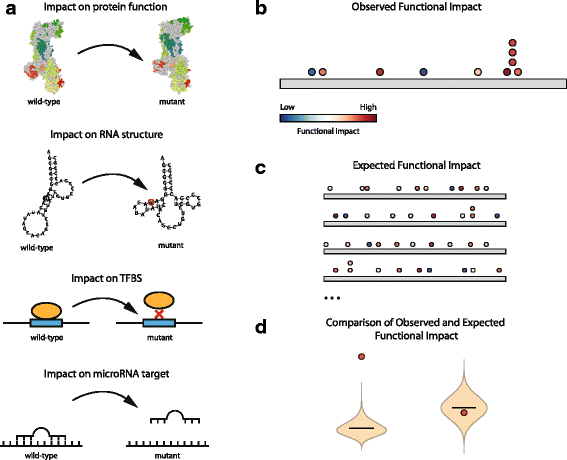
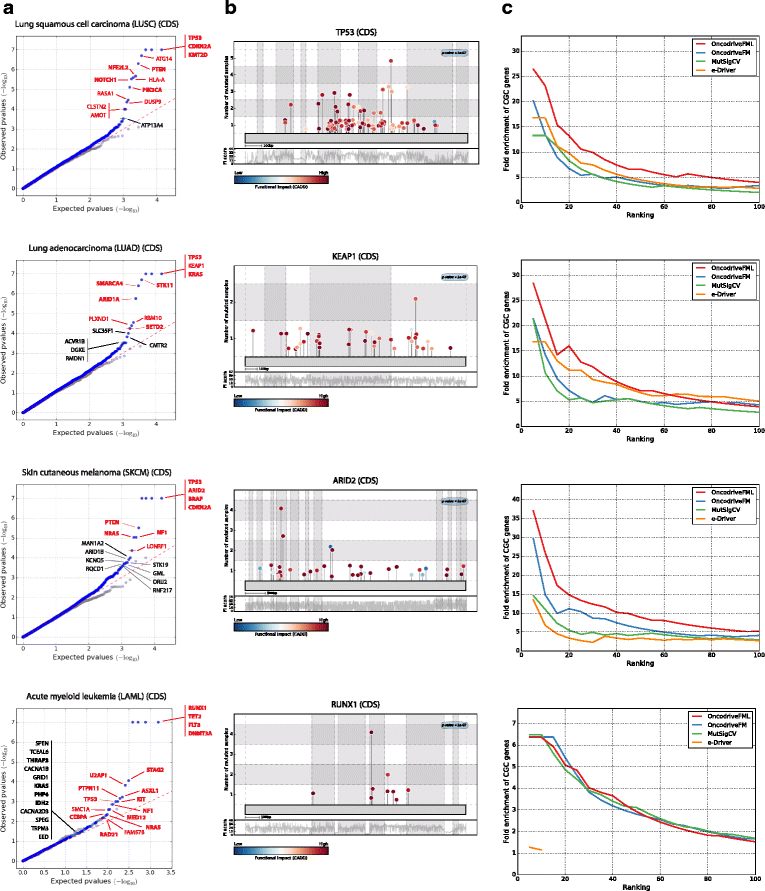
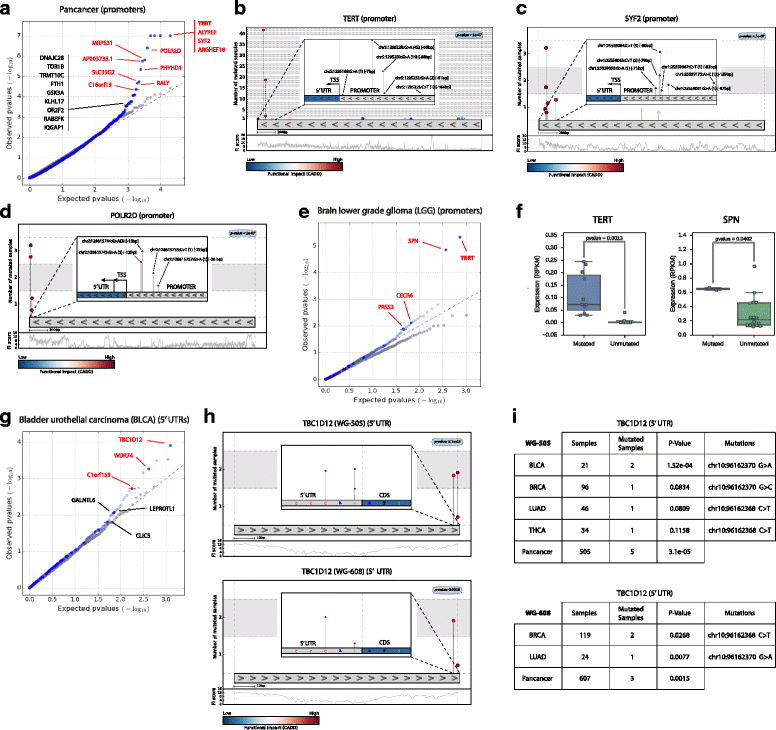
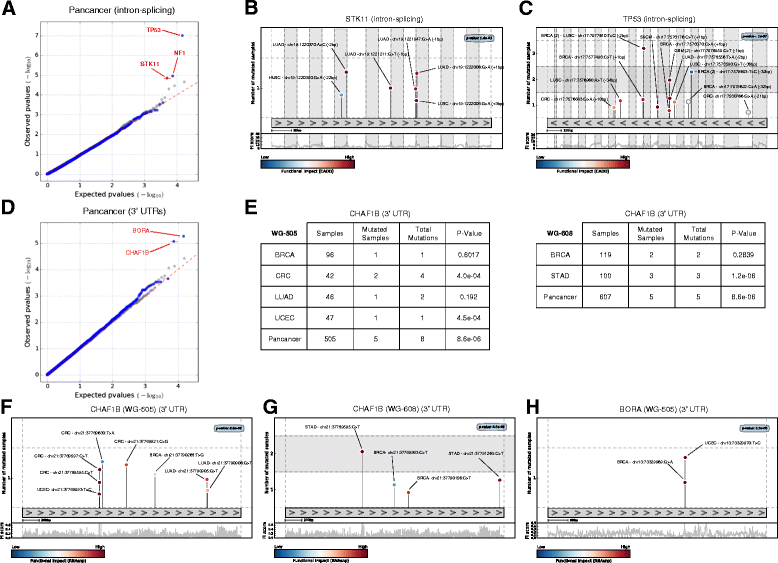
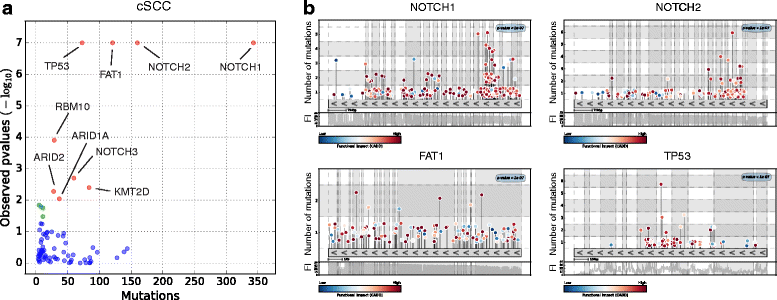
Similar articles
-
CNCDatabase: a database of non-coding cancer drivers.Nucleic Acids Res. 2021 Jan 8;49(D1):D1094-D1101. doi: 10.1093/nar/gkaa915. Nucleic Acids Res. 2021. PMID: 33095860 Free PMC article.
-
Genome-wide mapping of somatic mutation rates uncovers drivers of cancer.Nat Biotechnol. 2022 Nov;40(11):1634-1643. doi: 10.1038/s41587-022-01353-8. Epub 2022 Jun 20. Nat Biotechnol. 2022. PMID: 35726091 Free PMC article.
-
Cancer regulatory variation.Curr Opin Genet Dev. 2021 Feb;66:41-49. doi: 10.1016/j.gde.2020.11.010. Epub 2021 Jan 7. Curr Opin Genet Dev. 2021. PMID: 33422949 Review.
-
Identification of coding and non-coding mutational hotspots in cancer genomes.BMC Genomics. 2017 Jan 5;18(1):17. doi: 10.1186/s12864-016-3420-9. BMC Genomics. 2017. PMID: 28056774 Free PMC article.
-
Beyond the exome: the role of non-coding somatic mutations in cancer.Ann Oncol. 2016 Feb;27(2):240-8. doi: 10.1093/annonc/mdv561. Epub 2015 Nov 23. Ann Oncol. 2016. PMID: 26598542 Review.
Cited by
-
Genomic landscape of adult testicular germ cell tumours in the 100,000 Genomes Project.Nat Commun. 2024 Oct 26;15(1):9247. doi: 10.1038/s41467-024-53193-6. Nat Commun. 2024. PMID: 39461959 Free PMC article.
-
The genomic landscape of 2,023 colorectal cancers.Nature. 2024 Sep;633(8028):127-136. doi: 10.1038/s41586-024-07747-9. Epub 2024 Aug 7. Nature. 2024. PMID: 39112709 Free PMC article.
-
MutSpot: detection of non-coding mutation hotspots in cancer genomes.NPJ Genom Med. 2020 Jun 5;5:26. doi: 10.1038/s41525-020-0133-4. eCollection 2020. NPJ Genom Med. 2020. PMID: 32550006 Free PMC article.
-
Position-dependent function of human sequence-specific transcription factors.Nature. 2024 Jul;631(8022):891-898. doi: 10.1038/s41586-024-07662-z. Epub 2024 Jul 17. Nature. 2024. PMID: 39020164 Free PMC article.
-
Experimental identification of cancer driver alterations in the era of pan-cancer genomics.Cancer Sci. 2019 Dec;110(12):3622-3629. doi: 10.1111/cas.14210. Epub 2019 Oct 31. Cancer Sci. 2019. PMID: 31594033 Free PMC article. Review.
References
Publication types
MeSH terms
Substances
LinkOut - more resources
Full Text Sources
Other Literature Sources