TSCAN: Pseudo-time reconstruction and evaluation in single-cell RNA-seq analysis
- PMID: 27179027
- PMCID: PMC4994863
- DOI: 10.1093/nar/gkw430
TSCAN: Pseudo-time reconstruction and evaluation in single-cell RNA-seq analysis
Abstract
When analyzing single-cell RNA-seq data, constructing a pseudo-temporal path to order cells based on the gradual transition of their transcriptomes is a useful way to study gene expression dynamics in a heterogeneous cell population. Currently, a limited number of computational tools are available for this task, and quantitative methods for comparing different tools are lacking. Tools for Single Cell Analysis (TSCAN) is a software tool developed to better support in silico pseudo-Time reconstruction in Single-Cell RNA-seq ANalysis. TSCAN uses a cluster-based minimum spanning tree (MST) approach to order cells. Cells are first grouped into clusters and an MST is then constructed to connect cluster centers. Pseudo-time is obtained by projecting each cell onto the tree, and the ordered sequence of cells can be used to study dynamic changes of gene expression along the pseudo-time. Clustering cells before MST construction reduces the complexity of the tree space. This often leads to improved cell ordering. It also allows users to conveniently adjust the ordering based on prior knowledge. TSCAN has a graphical user interface (GUI) to support data visualization and user interaction. Furthermore, quantitative measures are developed to objectively evaluate and compare different pseudo-time reconstruction methods. TSCAN is available at https://github.com/zji90/TSCAN and as a Bioconductor package.
© The Author(s) 2016. Published by Oxford University Press on behalf of Nucleic Acids Research.
Figures
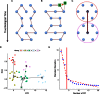
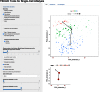
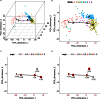
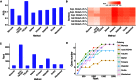
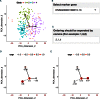
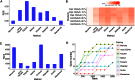
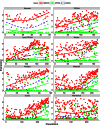
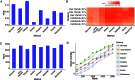
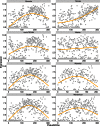
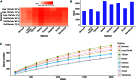
Similar articles
-
Pseudotime Reconstruction Using TSCAN.Methods Mol Biol. 2019;1935:115-124. doi: 10.1007/978-1-4939-9057-3_8. Methods Mol Biol. 2019. PMID: 30758823
-
visnormsc: A Graphical User Interface to Normalize Single-cell RNA Sequencing Data.Interdiscip Sci. 2018 Sep;10(3):636-640. doi: 10.1007/s12539-017-0277-9. Epub 2017 Dec 26. Interdiscip Sci. 2018. PMID: 29280088
-
Integration of single-cell RNA-seq data into population models to characterize cancer metabolism.PLoS Comput Biol. 2019 Feb 28;15(2):e1006733. doi: 10.1371/journal.pcbi.1006733. eCollection 2019 Feb. PLoS Comput Biol. 2019. PMID: 30818329 Free PMC article.
-
High Throughput Single Cell RNA Sequencing, Bioinformatics Analysis and Applications.Adv Exp Med Biol. 2018;1068:33-43. doi: 10.1007/978-981-13-0502-3_4. Adv Exp Med Biol. 2018. PMID: 29943294 Review.
-
Inclusion of temporal information in single cell transcriptomics.Int J Biochem Cell Biol. 2020 May;122:105745. doi: 10.1016/j.biocel.2020.105745. Epub 2020 Apr 10. Int J Biochem Cell Biol. 2020. PMID: 32283227 Review.
Cited by
-
scRNMF: An imputation method for single-cell RNA-seq data by robust and non-negative matrix factorization.PLoS Comput Biol. 2024 Aug 8;20(8):e1012339. doi: 10.1371/journal.pcbi.1012339. eCollection 2024 Aug. PLoS Comput Biol. 2024. PMID: 39116191 Free PMC article.
-
New perspectives on biology, disease progression, and therapy response of head and neck cancer gained from single cell RNA sequencing and spatial transcriptomics.Oncol Res. 2023 Nov 15;32(1):1-17. doi: 10.32604/or.2023.044774. eCollection 2023. Oncol Res. 2023. PMID: 38188682 Free PMC article. Review.
-
Single-Cell RNA Sequencing: A New Window into Cell Scale Dynamics.Biophys J. 2018 Aug 7;115(3):429-435. doi: 10.1016/j.bpj.2018.07.003. Epub 2018 Jul 11. Biophys J. 2018. PMID: 30033145 Free PMC article. Review.
-
Joint dimension reduction and clustering analysis of single-cell RNA-seq and spatial transcriptomics data.Nucleic Acids Res. 2022 Jul 8;50(12):e72. doi: 10.1093/nar/gkac219. Nucleic Acids Res. 2022. PMID: 35349708 Free PMC article.
-
Reconstructing complex lineage trees from scRNA-seq data using MERLoT.Nucleic Acids Res. 2019 Sep 26;47(17):8961-8974. doi: 10.1093/nar/gkz706. Nucleic Acids Res. 2019. PMID: 31428793 Free PMC article.
References
-
- Tang F., Barbacioru C., Wang Y., Nordman E., Lee C., Xu N., Wang X., Bodeau J., Tuch B.B., Siddiqui A., et al. mRNA-Seq whole-transcriptome analysis of a single cell. Nat. Methods. 2009;6:377–382. - PubMed
-
- Mortazavi A., Williams B.A., McCue K., Schaeffer L., Wold B. Mapping and quantifying mammalian transcriptomes by RNA-Seq. Nat. Methods. 2008;5:621–628. - PubMed
-
- Schena M., Shalon D., Davis R. W., Brown P.O. Quantitative monitoring of gene expression patterns with a complementary DNA microarray. Science. 1995;270:467–470. - PubMed
Publication types
MeSH terms
Substances
Grants and funding
LinkOut - more resources
Full Text Sources
Other Literature Sources
Molecular Biology Databases