Wishbone identifies bifurcating developmental trajectories from single-cell data
- PMID: 27136076
- PMCID: PMC4900897
- DOI: 10.1038/nbt.3569
Wishbone identifies bifurcating developmental trajectories from single-cell data
Abstract
Recent single-cell analysis technologies offer an unprecedented opportunity to elucidate developmental pathways. Here we present Wishbone, an algorithm for positioning single cells along bifurcating developmental trajectories with high resolution. Wishbone uses multi-dimensional single-cell data, such as mass cytometry or RNA-Seq data, as input and orders cells according to their developmental progression, and it pinpoints bifurcation points by labeling each cell as pre-bifurcation or as one of two post-bifurcation cell fates. Using 30-channel mass cytometry data, we show that Wishbone accurately recovers the known stages of T-cell development in the mouse thymus, including the bifurcation point. We also apply the algorithm to mouse myeloid differentiation and demonstrate its generalization to additional lineages. A comparison of Wishbone to diffusion maps, SCUBA and Monocle shows that it outperforms these methods both in the accuracy of ordering cells and in the correct identification of branch points.
Conflict of interest statement
The authors declare no competing financial interests.
Figures
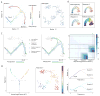
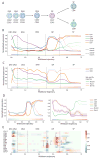
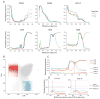
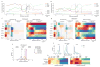
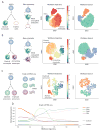
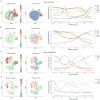
Similar articles
-
A Branch Point on Differentiation Trajectory is the Bifurcating Event Revealed by Dynamical Network Biomarker Analysis of Single-Cell Data.IEEE/ACM Trans Comput Biol Bioinform. 2020 Mar-Apr;17(2):366-375. doi: 10.1109/TCBB.2018.2847690. Epub 2018 Jun 15. IEEE/ACM Trans Comput Biol Bioinform. 2020. PMID: 29994127
-
Reversed graph embedding resolves complex single-cell trajectories.Nat Methods. 2017 Oct;14(10):979-982. doi: 10.1038/nmeth.4402. Epub 2017 Aug 21. Nat Methods. 2017. PMID: 28825705 Free PMC article.
-
Mpath maps multi-branching single-cell trajectories revealing progenitor cell progression during development.Nat Commun. 2016 Jun 30;7:11988. doi: 10.1038/ncomms11988. Nat Commun. 2016. PMID: 27356503 Free PMC article.
-
Thymopoiesis in 4 dimensions.Semin Immunol. 2005 Feb;17(1):95-102. doi: 10.1016/j.smim.2004.09.008. Semin Immunol. 2005. PMID: 15582492 Review.
-
Developmental program of mouse Valpha14i NKT cells.Curr Opin Immunol. 2005 Apr;17(2):122-30. doi: 10.1016/j.coi.2005.01.002. Curr Opin Immunol. 2005. PMID: 15766670 Review.
Cited by
-
From multitude to singularity: An up-to-date overview of scRNA-seq data generation and analysis.Front Genet. 2022 Oct 3;13:994069. doi: 10.3389/fgene.2022.994069. eCollection 2022. Front Genet. 2022. PMID: 36263428 Free PMC article. Review.
-
A Detailed Catalogue of Multi-Omics Methodologies for Identification of Putative Biomarkers and Causal Molecular Networks in Translational Cancer Research.Int J Mol Sci. 2021 Mar 10;22(6):2822. doi: 10.3390/ijms22062822. Int J Mol Sci. 2021. PMID: 33802234 Free PMC article. Review.
-
Diffusion pseudotime robustly reconstructs lineage branching.Nat Methods. 2016 Oct;13(10):845-8. doi: 10.1038/nmeth.3971. Epub 2016 Aug 29. Nat Methods. 2016. PMID: 27571553
-
Quantitative assessment of cell population diversity in single-cell landscapes.PLoS Biol. 2018 Oct 22;16(10):e2006687. doi: 10.1371/journal.pbio.2006687. eCollection 2018 Oct. PLoS Biol. 2018. PMID: 30346945 Free PMC article.
-
Modelling acute myeloid leukaemia in a continuum of differentiation states.Lett Biomath. 2018;5(Suppl 1):S69-S98. doi: 10.1080/23737867.2018.1472532. Epub 2018 Jun 18. Lett Biomath. 2018. PMID: 30271874 Free PMC article.
References
-
- Tang F, et al. mRNA-Seq whole-transcriptome analysis of a single cell. Nat Methods. 2009;6(5):377–82. - PubMed
Publication types
MeSH terms
Grants and funding
LinkOut - more resources
Full Text Sources
Other Literature Sources