Machine Learning: How Much Does It Tell about Protein Folding Rates?
- PMID: 26606303
- PMCID: PMC4659572
- DOI: 10.1371/journal.pone.0143166
Machine Learning: How Much Does It Tell about Protein Folding Rates?
Abstract
The prediction of protein folding rates is a necessary step towards understanding the principles of protein folding. Due to the increasing amount of experimental data, numerous protein folding models and predictors of protein folding rates have been developed in the last decade. The problem has also attracted the attention of scientists from computational fields, which led to the publication of several machine learning-based models to predict the rate of protein folding. Some of them claim to predict the logarithm of protein folding rate with an accuracy greater than 90%. However, there are reasons to believe that such claims are exaggerated due to large fluctuations and overfitting of the estimates. When we confronted three selected published models with new data, we found a much lower predictive power than reported in the original publications. Overly optimistic predictive powers appear from violations of the basic principles of machine-learning. We highlight common misconceptions in the studies claiming excessive predictive power and propose to use learning curves as a safeguard against those mistakes. As an example, we show that the current amount of experimental data is insufficient to build a linear predictor of logarithms of folding rates based on protein amino acid composition.
Conflict of interest statement
Figures
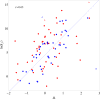
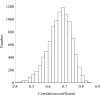
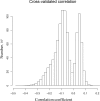
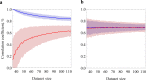
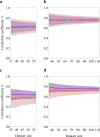
Similar articles
-
Machine learning algorithms for predicting protein folding rates and stability of mutant proteins: comparison with statistical methods.Curr Protein Pept Sci. 2011 Sep;12(6):490-502. doi: 10.2174/138920311796957630. Curr Protein Pept Sci. 2011. PMID: 21787301 Review.
-
Towards more accurate prediction of protein folding rates: a review of the existing Web-based bioinformatics approaches.Brief Bioinform. 2015 Mar;16(2):314-24. doi: 10.1093/bib/bbu007. Epub 2014 Mar 11. Brief Bioinform. 2015. PMID: 24621527 Review.
-
AlphaFold - A Personal Perspective on the Impact of Machine Learning.J Mol Biol. 2021 Oct 1;433(20):167088. doi: 10.1016/j.jmb.2021.167088. Epub 2021 Jun 2. J Mol Biol. 2021. PMID: 34087198
-
Recent Progress in Machine Learning-Based Methods for Protein Fold Recognition.Int J Mol Sci. 2016 Dec 16;17(12):2118. doi: 10.3390/ijms17122118. Int J Mol Sci. 2016. PMID: 27999256 Free PMC article. Review.
-
Prediction of protein folding rates from simplified secondary structure alphabet.J Theor Biol. 2015 Oct 21;383:1-6. doi: 10.1016/j.jtbi.2015.07.024. Epub 2015 Aug 4. J Theor Biol. 2015. PMID: 26247139
Cited by
-
Network measures for protein folding state discrimination.Sci Rep. 2016 Jul 28;6:30367. doi: 10.1038/srep30367. Sci Rep. 2016. PMID: 27464796 Free PMC article.
-
Circuit topology predicts pathogenicity of missense mutations.Proteins. 2022 Sep;90(9):1634-1644. doi: 10.1002/prot.26342. Epub 2022 Apr 23. Proteins. 2022. PMID: 35394672 Free PMC article.
-
Solution of Levinthal's Paradox and a Physical Theory of Protein Folding Times.Biomolecules. 2020 Feb 6;10(2):250. doi: 10.3390/biom10020250. Biomolecules. 2020. PMID: 32041303 Free PMC article. Review.
-
Protein folding in vitro and in the cell: From a solitary journey to a team effort.Biophys Chem. 2022 Aug;287:106821. doi: 10.1016/j.bpc.2022.106821. Epub 2022 Apr 29. Biophys Chem. 2022. PMID: 35667131 Free PMC article. Review.
-
Non-H3 CDR template selection in antibody modeling through machine learning.PeerJ. 2019 Jan 11;7:e6179. doi: 10.7717/peerj.6179. eCollection 2019. PeerJ. 2019. PMID: 30648015 Free PMC article.
References
-
- Sali A, Shakhnovich E, Karplus M. Kinetics of protein folding. A lattice model study of the requirements for folding to the native state. J Mol Biol. 1994;235: 1614–1636. - PubMed
Publication types
MeSH terms
Substances
Grants and funding
LinkOut - more resources
Full Text Sources
Other Literature Sources
Research Materials