Single-cell ChIP-seq reveals cell subpopulations defined by chromatin state
- PMID: 26458175
- PMCID: PMC4636926
- DOI: 10.1038/nbt.3383
Single-cell ChIP-seq reveals cell subpopulations defined by chromatin state
Abstract
Chromatin profiling provides a versatile means to investigate functional genomic elements and their regulation. However, current methods yield ensemble profiles that are insensitive to cell-to-cell variation. Here we combine microfluidics, DNA barcoding and sequencing to collect chromatin data at single-cell resolution. We demonstrate the utility of the technology by assaying thousands of individual cells and using the data to deconvolute a mixture of ES cells, fibroblasts and hematopoietic progenitors into high-quality chromatin state maps for each cell type. The data from each single cell are sparse, comprising on the order of 1,000 unique reads. However, by assaying thousands of ES cells, we identify a spectrum of subpopulations defined by differences in chromatin signatures of pluripotency and differentiation priming. We corroborate these findings by comparison to orthogonal single-cell gene expression data. Our method for single-cell analysis reveals aspects of epigenetic heterogeneity not captured by transcriptional analysis alone.
Figures
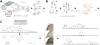
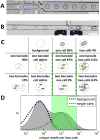
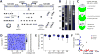
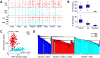
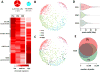
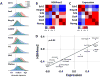
Comment in
-
Technology: Dropping in on single-cell epigenetic profiles.Nat Rev Genet. 2015 Dec;16(12):684-5. doi: 10.1038/nrg4036. Epub 2015 Oct 27. Nat Rev Genet. 2015. PMID: 26503794 No abstract available.
Similar articles
-
Technology: Dropping in on single-cell epigenetic profiles.Nat Rev Genet. 2015 Dec;16(12):684-5. doi: 10.1038/nrg4036. Epub 2015 Oct 27. Nat Rev Genet. 2015. PMID: 26503794 No abstract available.
-
Efficient library preparation for next-generation sequencing analysis of genome-wide epigenetic and transcriptional landscapes in embryonic stem cells.Methods Mol Biol. 2014;1150:3-20. doi: 10.1007/978-1-4939-0512-6_1. Methods Mol Biol. 2014. PMID: 24743988
-
High-throughput single-cell ChIP-seq identifies heterogeneity of chromatin states in breast cancer.Nat Genet. 2019 Jun;51(6):1060-1066. doi: 10.1038/s41588-019-0424-9. Epub 2019 May 31. Nat Genet. 2019. PMID: 31152164
-
The application of next-generation sequencing techniques in studying transcriptional regulation in embryonic stem cells.Yi Chuan. 2017 Aug 20;39(8):717-725. doi: 10.16288/j.yczz.16-390. Yi Chuan. 2017. PMID: 28903899 Review.
-
Dissection of gene regulatory networks in embryonic stem cells by means of high-throughput sequencing.Biol Chem. 2009 Nov;390(11):1139-44. doi: 10.1515/BC.2009.116. Biol Chem. 2009. PMID: 19642873 Review.
Cited by
-
Recent Advances in Single-Cell Profiling and Multispecific Therapeutics: Paving the Way for a New Era of Precision Medicine Targeting Cardiac Fibroblasts.Curr Cardiol Rep. 2021 Jun 3;23(7):82. doi: 10.1007/s11886-021-01517-z. Curr Cardiol Rep. 2021. PMID: 34081224 Free PMC article. Review.
-
Rapid parallel generation of a fluorescently barcoded drop library from a microtiter plate using the plate-interfacing parallel encapsulation (PIPE) chip.Lab Chip. 2022 Nov 22;22(23):4735-4745. doi: 10.1039/d2lc00909a. Lab Chip. 2022. PMID: 36367139 Free PMC article.
-
Single-cell ATAC-seq signal extraction and enhancement with SCATE.Genome Biol. 2020 Jul 3;21(1):161. doi: 10.1186/s13059-020-02075-3. Genome Biol. 2020. PMID: 32620137 Free PMC article.
-
Chromatin immunoprecipitation and an open chromatin assay in zebrafish erythrocytes.Methods Cell Biol. 2016;135:387-412. doi: 10.1016/bs.mcb.2016.04.019. Epub 2016 Jun 20. Methods Cell Biol. 2016. PMID: 27443937 Free PMC article.
-
Single-cell multiomics of the human retina reveals hierarchical transcription factor collaboration in mediating cell type-specific effects of genetic variants on gene regulation.Genome Biol. 2023 Nov 27;24(1):269. doi: 10.1186/s13059-023-03111-8. Genome Biol. 2023. PMID: 38012720 Free PMC article.
References
Bibliography and references cited
Bibliography and References Cited in Online Methods Only
-
- Venables WN. Modern applied statistics with S. 4th. Springer; New York: 2002.
Publication types
MeSH terms
Substances
Grants and funding
LinkOut - more resources
Full Text Sources
Other Literature Sources
Molecular Biology Databases