New Statistical Learning Methods for Estimating Optimal Dynamic Treatment Regimes
- PMID: 26236062
- PMCID: PMC4517946
- DOI: 10.1080/01621459.2014.937488
New Statistical Learning Methods for Estimating Optimal Dynamic Treatment Regimes
Abstract
Dynamic treatment regimes (DTRs) are sequential decision rules for individual patients that can adapt over time to an evolving illness. The goal is to accommodate heterogeneity among patients and find the DTR which will produce the best long term outcome if implemented. We introduce two new statistical learning methods for estimating the optimal DTR, termed backward outcome weighted learning (BOWL), and simultaneous outcome weighted learning (SOWL). These approaches convert individualized treatment selection into an either sequential or simultaneous classification problem, and can thus be applied by modifying existing machine learning techniques. The proposed methods are based on directly maximizing over all DTRs a nonparametric estimator of the expected long-term outcome; this is fundamentally different than regression-based methods, for example Q-learning, which indirectly attempt such maximization and rely heavily on the correctness of postulated regression models. We prove that the resulting rules are consistent, and provide finite sample bounds for the errors using the estimated rules. Simulation results suggest the proposed methods produce superior DTRs compared with Q-learning especially in small samples. We illustrate the methods using data from a clinical trial for smoking cessation.
Keywords: Classification; Dynamic treatment regimes; Personalized medicine; Q-learning; Reinforcement learning; Risk Bound; Support vector machine.
Figures
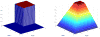
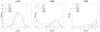
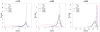
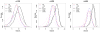
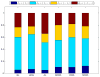
Similar articles
-
Use of personalized Dynamic Treatment Regimes (DTRs) and Sequential Multiple Assignment Randomized Trials (SMARTs) in mental health studies.Shanghai Arch Psychiatry. 2014 Dec;26(6):376-83. doi: 10.11919/j.issn.1002-0829.214172. Shanghai Arch Psychiatry. 2014. PMID: 25642116 Free PMC article.
-
Adaptive contrast weighted learning for multi-stage multi-treatment decision-making.Biometrics. 2017 Mar;73(1):145-155. doi: 10.1111/biom.12539. Epub 2016 May 23. Biometrics. 2017. PMID: 27213913
-
TREE-BASED REINFORCEMENT LEARNING FOR ESTIMATING OPTIMAL DYNAMIC TREATMENT REGIMES.Ann Appl Stat. 2018 Sep;12(3):1914-1938. doi: 10.1214/18-AOAS1137. Epub 2018 Sep 11. Ann Appl Stat. 2018. PMID: 30984321 Free PMC article.
-
Estimating Individualized Treatment Rules Using Outcome Weighted Learning.J Am Stat Assoc. 2012 Sep 1;107(449):1106-1118. doi: 10.1080/01621459.2012.695674. J Am Stat Assoc. 2012. PMID: 23630406 Free PMC article.
-
Bayesian inference for optimal dynamic treatment regimes in practice.Int J Biostat. 2023 May 17;19(2):309-331. doi: 10.1515/ijb-2022-0073. eCollection 2023 Nov 1. Int J Biostat. 2023. PMID: 37192544 Review.
Cited by
-
On restricted optimal treatment regime estimation for competing risks data.Biostatistics. 2021 Apr 10;22(2):217-232. doi: 10.1093/biostatistics/kxz026. Biostatistics. 2021. PMID: 31373360 Free PMC article.
-
Deep reinforcement learning for personalized treatment recommendation.Stat Med. 2022 Sep 10;41(20):4034-4056. doi: 10.1002/sim.9491. Epub 2022 Jun 18. Stat Med. 2022. PMID: 35716038 Free PMC article.
-
Learning Individualized Treatment Rules for Multiple-Domain Latent Outcomes.J Am Stat Assoc. 2021;116(533):269-282. doi: 10.1080/01621459.2020.1817751. Epub 2020 Oct 19. J Am Stat Assoc. 2021. PMID: 34776561 Free PMC article.
-
Precision Medicine.Annu Rev Stat Appl. 2019 Mar;6:263-286. doi: 10.1146/annurev-statistics-030718-105251. Annu Rev Stat Appl. 2019. PMID: 31073534 Free PMC article.
-
Tree based weighted learning for estimating individualized treatment rules with censored data.Electron J Stat. 2017;11(2):3927-3953. doi: 10.1214/17-EJS1305. Epub 2017 Oct 18. Electron J Stat. 2017. PMID: 29403568 Free PMC article.
References
-
- Bartlett PL, Jordan MI, McAuliffe JD. Convexity, Classification, and Risk Bounds. JASA. 2006;101(473):138–156.
-
- Bellman R. Dynamic Programming. Princeton: Princeton Univeristy Press; 1957.
-
- Blanchard G, Bousquet O, Massart P. Statistical Performance of Support Vector Machines. The Annals of Statistics. 2008;36:489–531.
-
- Blatt D, Murphy SA, Zhu J. A-learning for approximate planning. 2004. Unpublished Manuscript.
-
- Bradley PS, Mangasarian OL. Feature Selection via Concave Minimization and Support Vector Machines. Proc. 15th International Conf. on Machine Learning; San Francisco, CA, USA: Morgan Kaufmann Publishers Inc; 1998.
Grants and funding
LinkOut - more resources
Full Text Sources
Other Literature Sources