Single-cell chromatin accessibility reveals principles of regulatory variation
- PMID: 26083756
- PMCID: PMC4685948
- DOI: 10.1038/nature14590
Single-cell chromatin accessibility reveals principles of regulatory variation
Abstract
Cell-to-cell variation is a universal feature of life that affects a wide range of biological phenomena, from developmental plasticity to tumour heterogeneity. Although recent advances have improved our ability to document cellular phenotypic variation, the fundamental mechanisms that generate variability from identical DNA sequences remain elusive. Here we reveal the landscape and principles of mammalian DNA regulatory variation by developing a robust method for mapping the accessible genome of individual cells by assay for transposase-accessible chromatin using sequencing (ATAC-seq) integrated into a programmable microfluidics platform. Single-cell ATAC-seq (scATAC-seq) maps from hundreds of single cells in aggregate closely resemble accessibility profiles from tens of millions of cells and provide insights into cell-to-cell variation. Accessibility variance is systematically associated with specific trans-factors and cis-elements, and we discover combinations of trans-factors associated with either induction or suppression of cell-to-cell variability. We further identify sets of trans-factors associated with cell-type-specific accessibility variance across eight cell types. Targeted perturbations of cell cycle or transcription factor signalling evoke stimulus-specific changes in this observed variability. The pattern of accessibility variation in cis across the genome recapitulates chromosome compartments de novo, linking single-cell accessibility variation to three-dimensional genome organization. Single-cell analysis of DNA accessibility provides new insight into cellular variation of the 'regulome'.
Conflict of interest statement
Stanford University has filed a provisional patent application on the methods described, and J.D.B., H.Y.C., and W.J.G. are named as inventors. D.R. and M.L.G. declare competing financial interests as employees of Fluidigm Corp.
Figures
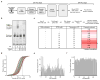
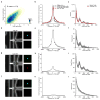
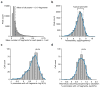
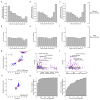
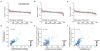
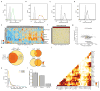
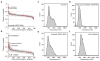
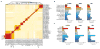
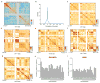
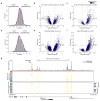
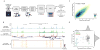
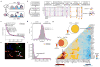
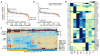
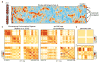
Comment in
-
Single-cell ATAC-seq: strength in numbers.Genome Biol. 2015 Aug 21;16(1):172. doi: 10.1186/s13059-015-0737-7. Genome Biol. 2015. PMID: 26294014 Free PMC article.
Similar articles
-
Efficient chromatin accessibility mapping in situ by nucleosome-tethered tagmentation.Elife. 2020 Nov 16;9:e63274. doi: 10.7554/eLife.63274. Elife. 2020. PMID: 33191916 Free PMC article.
-
A Single-Cell Atlas of In Vivo Mammalian Chromatin Accessibility.Cell. 2018 Aug 23;174(5):1309-1324.e18. doi: 10.1016/j.cell.2018.06.052. Epub 2018 Aug 2. Cell. 2018. PMID: 30078704 Free PMC article.
-
Coupled Single-Cell CRISPR Screening and Epigenomic Profiling Reveals Causal Gene Regulatory Networks.Cell. 2019 Jan 10;176(1-2):361-376.e17. doi: 10.1016/j.cell.2018.11.022. Epub 2018 Dec 20. Cell. 2019. PMID: 30580963 Free PMC article.
-
Application of Single-Cell Assay for Transposase-Accessible Chromatin with High Throughput Sequencing in Plant Science: Advances, Technical Challenges, and Prospects.Int J Mol Sci. 2024 Jan 25;25(3):1479. doi: 10.3390/ijms25031479. Int J Mol Sci. 2024. PMID: 38338756 Free PMC article. Review.
-
[Advances in assay for transposase-accessible chromatin with high-throughput sequencing].Yi Chuan. 2020 Apr 20;42(4):333-346. doi: 10.16288/j.yczz.19-279. Yi Chuan. 2020. PMID: 32312702 Review. Chinese.
Cited by
-
Heat stress causes chromatin accessibility and related gene expression changes in crown tissues of barley (Hordeum vulgare).Plant Mol Biol. 2024 Oct 22;114(6):115. doi: 10.1007/s11103-024-01509-x. Plant Mol Biol. 2024. PMID: 39436452 Free PMC article.
-
coupleCoC+: An information-theoretic co-clustering-based transfer learning framework for the integrative analysis of single-cell genomic data.PLoS Comput Biol. 2021 Jun 2;17(6):e1009064. doi: 10.1371/journal.pcbi.1009064. eCollection 2021 Jun. PLoS Comput Biol. 2021. PMID: 34077420 Free PMC article.
-
Epigenomic and transcriptomic analysis of chronic inflammatory diseases.Genes Genomics. 2021 Mar;43(3):227-236. doi: 10.1007/s13258-021-01045-8. Epub 2021 Feb 27. Genes Genomics. 2021. PMID: 33638813 Review.
-
Mechanical Regulation of Transcription: Recent Advances.Trends Cell Biol. 2021 Jun;31(6):457-472. doi: 10.1016/j.tcb.2021.02.008. Epub 2021 Mar 9. Trends Cell Biol. 2021. PMID: 33712293 Free PMC article. Review.
-
Single-Cell Sequencing Applications in the Inner Ear.Front Cell Dev Biol. 2021 Feb 12;9:637779. doi: 10.3389/fcell.2021.637779. eCollection 2021. Front Cell Dev Biol. 2021. PMID: 33644075 Free PMC article. Review.
References
-
- Imayoshi I, et al. Oscillatory control of factors determining multipotency and fate in mouse neural progenitors. Science. 2013;342:1203–1208. - PubMed
Publication types
MeSH terms
Substances
Associated data
- Actions
Grants and funding
LinkOut - more resources
Full Text Sources
Other Literature Sources
Molecular Biology Databases