Combined Single-Cell Functional and Gene Expression Analysis Resolves Heterogeneity within Stem Cell Populations
- PMID: 26004780
- PMCID: PMC4460190
- DOI: 10.1016/j.stem.2015.04.004
Combined Single-Cell Functional and Gene Expression Analysis Resolves Heterogeneity within Stem Cell Populations
Abstract
Heterogeneity within the self-renewal durability of adult hematopoietic stem cells (HSCs) challenges our understanding of the molecular framework underlying HSC function. Gene expression studies have been hampered by the presence of multiple HSC subtypes and contaminating non-HSCs in bulk HSC populations. To gain deeper insight into the gene expression program of murine HSCs, we combined single-cell functional assays with flow cytometric index sorting and single-cell gene expression assays. Through bioinformatic integration of these datasets, we designed an unbiased sorting strategy that separates non-HSCs away from HSCs, and single-cell transplantation experiments using the enriched population were combined with RNA-seq data to identify key molecules that associate with long-term durable self-renewal, producing a single-cell molecular dataset that is linked to functional stem cell activity. Finally, we demonstrated the broader applicability of this approach for linking key molecules with defined cellular functions in another stem cell system.
Copyright © 2015 The Authors. Published by Elsevier Inc. All rights reserved.
Figures
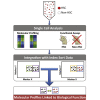
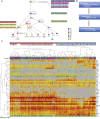
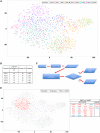
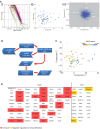
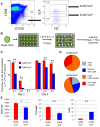
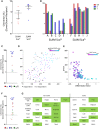
Comment in
-
Bringing Blood Stem Cell Phenotype, Genotype, and Function Closer Together.Cell Stem Cell. 2015 Jun 4;16(6):574-5. doi: 10.1016/j.stem.2015.05.001. Cell Stem Cell. 2015. PMID: 26046754
-
Looking Back: Single-Cell Analysis.Cell Stem Cell. 2017 Jun 1;20(6):758. doi: 10.1016/j.stem.2017.05.015. Cell Stem Cell. 2017. PMID: 28575694
Similar articles
-
Single-cell transcriptomic reconstruction reveals cell cycle and multi-lineage differentiation defects in Bcl11a-deficient hematopoietic stem cells.Genome Biol. 2015 Sep 21;16:178. doi: 10.1186/s13059-015-0739-5. Genome Biol. 2015. PMID: 26387834 Free PMC article.
-
Hoxb5 defines the heterogeneity of self-renewal capacity in the hematopoietic stem cell compartment.Biochem Biophys Res Commun. 2021 Feb 5;539:34-41. doi: 10.1016/j.bbrc.2020.12.077. Epub 2021 Jan 5. Biochem Biophys Res Commun. 2021. PMID: 33418191
-
High c-Kit expression identifies hematopoietic stem cells with impaired self-renewal and megakaryocytic bias.J Exp Med. 2014 Feb 10;211(2):217-31. doi: 10.1084/jem.20131128. Epub 2014 Jan 20. J Exp Med. 2014. PMID: 24446491 Free PMC article.
-
In-vivo differentiation of adult hematopoietic stem cells from a single-cell point of view.Curr Opin Hematol. 2020 Jul;27(4):241-247. doi: 10.1097/MOH.0000000000000587. Curr Opin Hematol. 2020. PMID: 32398457 Review.
-
Differentiation-based model of hematopoietic stem cell functions and lineage pathways.Blood. 2018 Sep 13;132(11):1106-1113. doi: 10.1182/blood-2018-03-791517. Epub 2018 Jul 24. Blood. 2018. PMID: 30042097 Free PMC article. Review.
Cited by
-
The post-stroke young adult brain has limited capacity to re-express the gene expression patterns seen during early postnatal brain development.Brain Pathol. 2024 Sep;34(5):e13232. doi: 10.1111/bpa.13232. Epub 2024 Jan 10. Brain Pathol. 2024. PMID: 38198833 Free PMC article.
-
CRISPR/Cas9 uPAR Gene Knockout Results in Tumor Growth Inhibition, EGFR Downregulation and Induction of Stemness Markers in Melanoma and Colon Carcinoma Cell Lines.Front Oncol. 2021 May 14;11:663225. doi: 10.3389/fonc.2021.663225. eCollection 2021. Front Oncol. 2021. PMID: 34055629 Free PMC article.
-
Stabilizing hematopoietic stem cells in vitro.Curr Opin Genet Dev. 2020 Oct;64:1-5. doi: 10.1016/j.gde.2020.05.035. Epub 2020 Jun 20. Curr Opin Genet Dev. 2020. PMID: 32570191 Free PMC article. Review.
-
Inflammation-induced emergency megakaryopoiesis: inflammation paves the way for platelets.Stem Cell Investig. 2016 May 13;3:16. doi: 10.21037/sci.2016.05.01. eCollection 2016. Stem Cell Investig. 2016. PMID: 27486586 Free PMC article. No abstract available.
-
BTR: training asynchronous Boolean models using single-cell expression data.BMC Bioinformatics. 2016 Sep 6;17(1):355. doi: 10.1186/s12859-016-1235-y. BMC Bioinformatics. 2016. PMID: 27600248 Free PMC article.
References
-
- Adolfsson J., Borge O.J., Bryder D., Theilgaard-Mönch K., Astrand-Grundström I., Sitnicka E., Sasaki Y., Jacobsen S.E. Upregulation of Flt3 expression within the bone marrow Lin(-)Sca1(+)c-kit(+) stem cell compartment is accompanied by loss of self-renewal capacity. Immunity. 2001;15:659–669. - PubMed
-
- Adolfsson J., Månsson R., Buza-Vidas N., Hultquist A., Liuba K., Jensen C.T., Bryder D., Yang L., Borge O.J., Thoren L.A. Identification of Flt3+ lympho-myeloid stem cells lacking erythro-megakaryocytic potential a revised road map for adult blood lineage commitment. Cell. 2005;121:295–306. - PubMed
-
- Akashi K., Traver D., Miyamoto T., Weissman I.L. A clonogenic common myeloid progenitor that gives rise to all myeloid lineages. Nature. 2000;404:193–197. - PubMed
Publication types
MeSH terms
Associated data
- Actions
Grants and funding
LinkOut - more resources
Full Text Sources
Other Literature Sources
Medical
Molecular Biology Databases